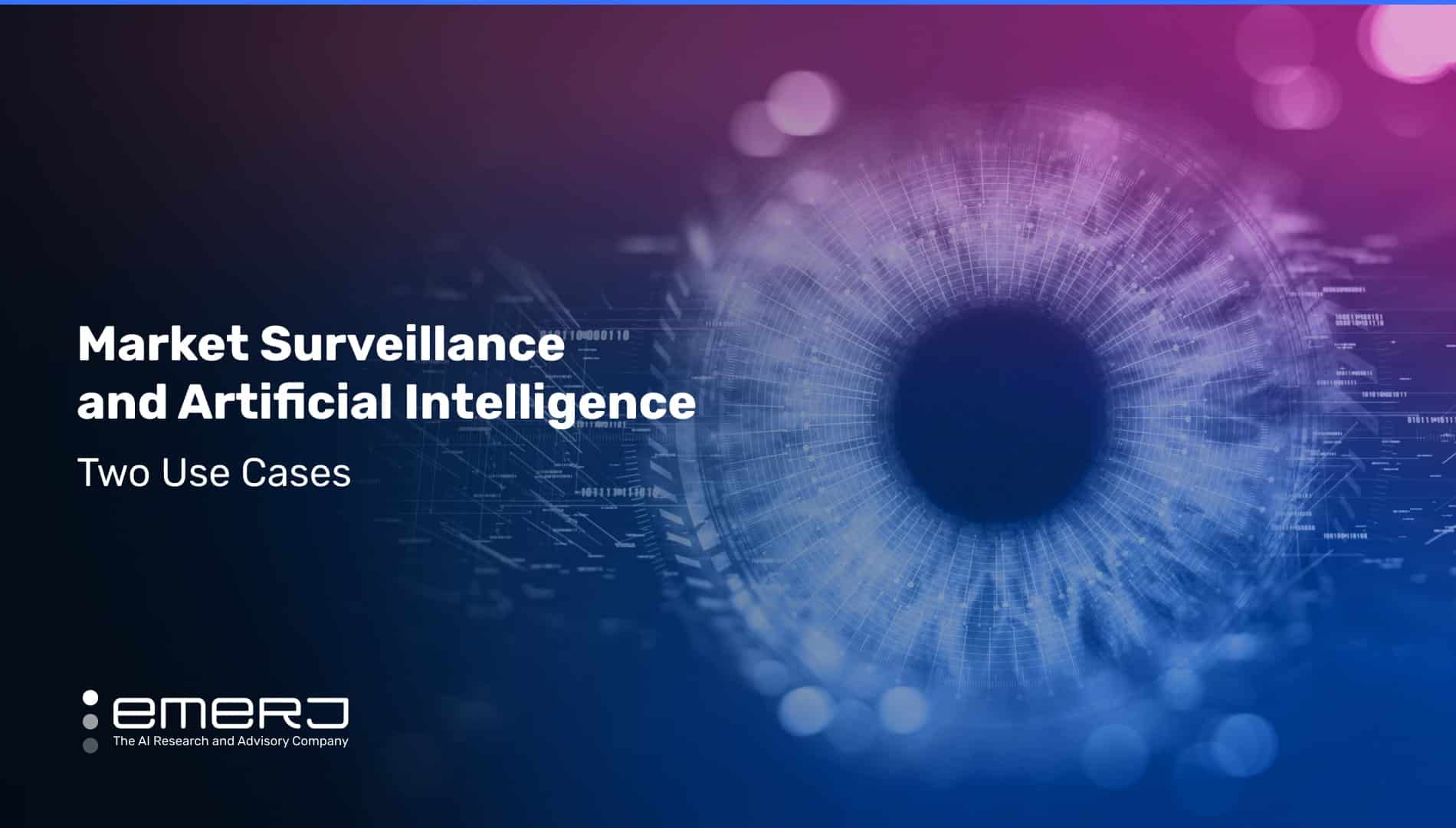
Market surveillance refers to activity authorities conduct to ensure that products available to consumers adhere to applicable laws and regulations. Additionally, market surveillance in banking and finance takes a somewhat specific form.
Market surveillance in finance aims to prevent market manipulation and other illegal or unethical behaviors, including fraud, money laundering, and insider trading.
The applicable laws and regulations relevant to market surveillance require financial institutions to comply with multiple trade monitoring mandates, including the Dodd-Frank Swaps Surveillance regulation and know-your-customer (KYC) and anti-money laundering (AML) compliance.
The case for using AI is well-established and almost necessary in today’s financial markets. In a policy brief from the Brookings Center on Regulation and Markets, Jo Ann Barefoot, CEO & Cofounder of the Alliance for Innovative Regulation, explained the following, “Today, there is so much data that not only can we use AI, but in many fields like financial regulation we have to use AI simply to keep up.”
This article explores two AI use cases used in market surveillance in financial markets:
- Improve detection of malicious activity: A combination of model training methods, including transfer, human-in-the-loop, and deep learning techniques for market surveillance to improve the detection rate of malicious activity.
- Proactively detect regulatory misconduct: Using AI-powered data cleansing capabilities to decrease false positives to improve reviewers’ speed and accuracy.
The article covers each case’s business problem and the impact of each AI solution.
Use Case #1: Improving Detection of Malicious Activity
Machine learning has its place in financial market surveillance for detecting anomalies or outlier behaviors that could indicate suspicious activity. Rule-based systems cannot adequately monitor market activity independently due to the complexity present in financial markets. Also, they make it difficult to keep up with bad actors who routinely adjust their strategies.
Sometimes, the false positive rate of alerts generated from market surveillance can be as high as 99.99%. False positives point to legitimate transactions that were incorrectly flagged as suspicious.
While it is often better to flag a transaction that ends up being legitimate than failing to detect a suspicious transaction (false negative), false positives can erode customer trust. Therefore, improving precision when detecting suspicious transactions is beneficial even if it is not immediately quantifiable.
It’s been a long-standing concern of professionals and executives responsible for compliance solutions within their respective firms. A 2019 Nasdaq Global Compliance Survey of 187 individuals showed that reducing false positives is the main challenge in KYC/AML monitoring.
Surveillance monitoring offers multiple benefits, including:
- Ability to manage reputational risk
- Maintaining regulatory risk
- Reducing operational complexity
- Safeguarding market integrity
The Nasdaq Stock Market is the world’s largest stock exchange by volume traded. In 2019, Nasdaq launched an artificial intelligence solution for market surveillance on The Nasdaq Stock Market. Nasdaq’s solution combines deep learning, transfer learning, and human-in-the-loop training to conduct market surveillance.
Not only is Nasdaq Trade Surveillance (SMARTS) used by over 170 banks and brokers and over 50 exchanges, but it is also the same surveillance technology that their global exchanges use.
The following video, shorter than two minutes in length, displays key areas of the SMARTS dashboard – including visualization of an alert containing order, trade, and market data:
Deep learning is machine learning based on neural networks that mimic the human brain. It allows computers to understand incredibly complex patterns and identify hidden relationships. This component of Nasdaq’s three-pronged solution offers a distinct advantage over the less robust, rule-based solutions.
Transfer learning allows the machine to apply what it has learned to a new but similar problem. These functions enable the detection of new forms of more complex financial crime. Furthermore, the human-in-the-loop learning in Nasdaq’s AI solution allows the analysts to share their knowledge and findings with the machine, freeing their time to focus on investigation and other activities.
One tangible benefit of implementing trade surveillance is minimizing the likelihood of facing a significant fine. Financial firms must adhere to regulations related to preventing market abuse and being able to identify it when it does occur. They run the risk of hefty fines if they fall short. In 2022, FINRA fined E*TRADE for “failing to maintain adequate surveillance systems.”
Use Case #2: Proactively Detect Regulatory Misconduct
Compliance professionals responsible for communication surveillance are inundated with many false positives that legacy systems often generate. Data cleansing, or data pre-processing, is a foundational step in machine learning pipelines. For Deutsche Bank, data cleansing also serves as the basis of a robust solution to generate alerts more efficiently.
Breaking Wave, a fintech firm under parent company Deutsche Bank, implemented Relativity Trace in August 2022. Relativity Trace is an AI-powered communication surveillance product. It can detect regulatory misconduct, including:
- Insider trading
- Collusion
Relativity Trace also includes Relativity Trace Email Thread Deduplication. This feature can locate old content contained within new emails and checks that it was previously analyzed so that a new alert is not generated unnecessarily.
The solution’s data cleansing capabilities identify the following and prevent new alerts from being generated:
- Email headers
- Signatures
- Confidentiality disclaimers
As a result, analysts are no longer bombarded with handling alerts that contain duplicate content.
The following video, less than two minutes in length, showcases the dashboard for the Relativity Trace platform:
The General Manager of Relativity Trace, Jordan Dormash, emphasized the number of hours saved by not having to handle content that should have never been alerted at all. As a result, compliance analysts avoid dealing with duplicate content and can focus on relevant information. This results in improved speed and accuracy for remaining reviews, but provided no specific measured results of the use case.
In terms of email surveillance platforms as the first phases of a larger AI use case, KUNGFU.AI Co-founder and Head of Innovation Steve Meier tells Emerj that the introduction of large language models in the financial technology sphere are enhancing the way humans interact with and use market data.
“Everyone’s going after this,” he says of the fintech rush and trail of acquisitions from the largest financial institutions in its wake, not unlike the use cases we’ve seen from Nasdaq and Breaking Wave above.
Furthermore, many use cases like email context detection stand to be enhanced dramatically over the years with the advancement of large language models like GPT-4. According to Meier, these developments “are conceptually possible and probably work in a narrow environment in isolation in a laboratory, but we’re not quite there yet.”