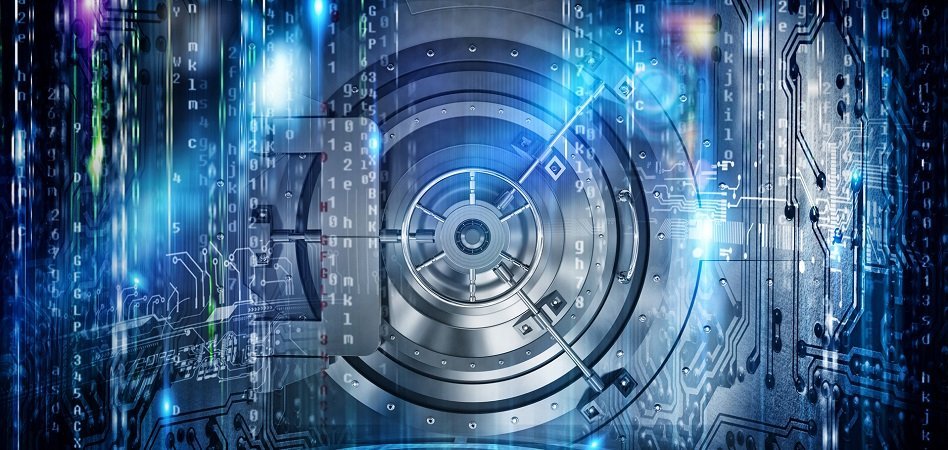
Banks are in one of the best positions for leveraging AI in the coming years because the largest banks have massive volumes of historical data on customers and transactions that can be fed into machine learning algorithms. We recently completed our Emerj AI in Banking Vendor Scorecard and Capability Map in which we explored which AI capabilities banks were taking advantage of the most and which they might be able to leverage in the future.
When it comes to big data in banking, banks might be primed to think about using their customer data to build a conversational interface or chatbot to improve the customer experience and, perhaps most importantly, attract millennial customers who are used to getting their needs met quickly over the internet. Despite this, banks are unlikely to leverage their customer data nowadays.
In fact, although over 35% of the press releases we explored for our report mentioned conversational interfaces, they represent only 8% of the total funding for AI vendors selling into banking. In other words, banks are talking about chatbots, but that’s not where the money is. What we found is that banks more than anything want to spend their money automating fraud detection and compliance, despite the fact that they won’t often talk about these innovations because they aren’t enticing to customers.
That said, customer-service related projects appear to be next in line for AI development at the top banks. These banks will start working with chatbot vendors and building their own chatbots in-house after they’ve begun to get a better understanding of the basics of artificial intelligence, including best practices for adopting it in the enterprise.
In this article, we’ll explore a few AI and third-party data vendors that offer big data platforms and machine learning to banks so that they might use their historical data for:
- Fraud and anti-money laundering
- Sales and entering new markets
We begin our exploration of how banks can use their big data with MapR’s data platform, which they claim is useful for building fraud detection algorithms.
Leveraging Big Data for Fraud and Anti-Money Laundering
MapR
MapR offers an enterprise-grade data platform which it claims can help financial services institutions create a data system that could help streamline operations in risk management, fraud detection, and compliance, creating a data environment in which to run anomaly detection, natural language processing, and predictive analytics algorithms.
The company’s MapR-DB platform, one of its products, purportedly allows banks to store their documents in a searchable database through which they could leverage machine learning. The company also states it can enrich enterprise databases with data from third-party data vendors including Equifax, Experian, TransUnion, DataStream International, and Bloomberg. This data can be used to create more robust and accurate machine learning algorithms for use-cases such as fraud detection and anti-money laundering, two AI use-cases that are at the top of bankers’ minds nowadays.
Below is a short 2-minute video demonstrating how the MapR-DB database, which is a component of MapR’s platform:
MapR claims to have helped Credit Agricole establish a data lake and aggregate all its internal data such operational systems and analytical systems as well as external data and make it accessible to customers, partners, and collaborators. According to the report, the integration took two years.
As a result of implementing MapR, the bank’s data scientists are now able to explore new datasets within the data lake to build new algorithmic models and enrich existing models. Its business intelligence teams use the tool to support decision-making at the company.
MapR also lists Audi, Boehringer Ingelheim, CJ Energy Services, Cisco, Credit Agricole, Eastern Bank, Ericsson, and HP, among others as one/some of their past clients.
Ted Dunning is the Chief Application Architect at MapR. He holds a PhD in computer science from Sheffield University. Previously, Dunning served as CTO at Deepdyve and Chief Scientist at several companies such as SiteTuners, Veoh Networks, ID Analytics and HNC Hardware.
Teradata
Teradata offers big data analytics which it claims can help financial services companies automate financial and accounting processes, minimize financial fraud and cybersecurity risks, and enhance customer experience using machine learning.
For instance, to predict financial crimes such as fraud, Teradata’s software could collect data across a bank’s different products and channels. One way to mitigate fraud risk is to stop it at the point where customers apply for accounts. The system would check data about previous customers, applications, transactions conducted via credit card, debit card, online, branch banking, ATMs, wire transfer, mobile, and call center.
The algorithms would compare the data and recognize anomalous patterns such as the same individual applying for several accounts using different addresses, devices, or permutations of the same name such as “John Jones Smith,” “JJ Smith,” “J. Jones Smith,” etc. Once the patterns are recognized, the application would then be able to predict which applications are potentially fraudulent and disapprove the customer’s application.
Below is a short 3-minute video demonstrating how Teradata’s cloud service can accommodate big data and perform analytics. The video explains how an unnamed client moves its data system of with 320 terabytes of data space into the Teradata cloud to perform 25 million queries monthly:
Teradata claims to have helped Lloyds Banking Group better understand their retail and commercial and develop strategy and pricing by establishing an analytics system. The case study reports that the client integrated customer insight, marketing and digital aspects of the bank to unify the data.
As a result, 24% of the bank’s income came from leads generated by the analytics system. The bank has also been able to establish a new product strategy using customer data from the analytics system. It has also been able to manage costs, detect potentially fraudulent activities, and build credit risk models. The biggest benefit of the big data analytics system, according to the case study, is being able to better explore the customer journey.
Teradata also lists Verizon, Siemens, Roche, P&G, Maersk, 3M, and British Airways as some of their past clients.
Stephen Brobst has been the CTO at Teradata since 1999. He holds a PhD in computer science from the Massachusetts Institute of Technology. Brobst currently also is an instructor of artificial intelligence and advanced machine learning at The Data Warehousing Institute. Previously, Brobst served as Lecturer at the Boston University and Co-founder and CTO of Nextek Solutions.
Big Data in Banking – Sales and Marketing
Axtria
Axtria offers a Cloud Information Management service, which it claims can help banking, financial services, and insurance companies explore new sources of data that banks could use to target the right customers, motivate the sales team to drive productivity, and streamline reporting. Axtria claims that their platform makes it easier for data scientists to find the data they need to train machine learning algorithms and that their platform performs 90% of the data preprocessing in the cloud. This in theory allows data analysts to spend more of their time on actual analysis.
From our numerous interviews with machine learning and data science experts on the AI in Industry podcast, we’ve come to understand that nowadays, most of a data scientist’s job involves cleaning and preparing data. This isn’t the highest-value thing they could be doing on a day to day basis. Ideally, they’d be building and tweaking algorithms. As such, many banks are likely to adopt data platforms that are designed for use with machine learning, especially as they start building data science teams in house to create compliance and customer service-related AI applications.
Axtria claims to have helped an unnamed credit card issuing company customize its targeting of customers through analytics. The client wanted to better understand its customers to match them appropriately with the right products.
The company then used Axtria’s machine learning algorithms to segment customers on credit card usage habits, risk profile, and purchasing habits. Customers with similar attributes were then profiled and grouped into key segments, scored via predictive analytics and mapped to appropriate products per segment.
Among the attributes the client company looked at were average account balance, spending and payment habits, and attrition rates. The client then used the data to predict current and future buying power of the customers and expected product margins per segment.
The case study reports that matching the right customer profile and segment resulted in a 20% increase in sales for target product lines. However, the client was unnamed, so we caution readers to take this case study with a grain of salt.
Axtria does not reveal the names of its clients, but has raised $44.7 million in funding from Helion Venture Partners, Richard Braddock, Deshpande Foundation, Fred Khosravi and Amarpreet Sawhney.
David Wood is a Principal at Axtria. He holds a PhD in operations research from the University of California, Berkeley. Previously, Wood served as Senior Principal at marketRX, a Cognizant company and Executive Director at Health Products Research, Inc.
McKinsey
McKinsey offers Panorama an application that combines banking and financial data sources and proprietary McKinsey data for predictive analytics, which the company claims can help banking, financial services institutions, and private equity firms identify which global markets they may want to enter and which financial technology company they can invest in.
McKinsey explains that the dataset is a market sizing database of more than 60 global markets, which allows banks to determine which markets to review and determine which one to enter or exit.
The database also contains a global database of fintech companies, allowing banks to determine the nuances between companies and their products and identify which companies they would like to invest in.
McKinsey explains that banks have access to the data set’s interface to perform analytics and visualizations of the data.
The company states that the machine learning model behind the software was trained on more than 100 million data points about 60 markets across the globe, and knowledge from more than 300 banking experts. The data would then be run through the software’s machine learning algorithm. This would have trained the algorithm to discern which data points correlate to banking markets by region and product, financial technology innovations, or global payments products.
The software would be able to predict which markets or fintech companies are the best to invest in. This may or may not require the user to upload information about their the new market the bank is eyeing to enter or the financial technology company they would like to invest in into the software beforehand.
McKinsey claims to have helped an unnamed bank in Europe reduce risk and improve capital planning by building algorithmic models for the bank’s portfolio products. McKinsey needed to assemble and integrate the bank’s disparate legacy data systems from companies it had acquired over the course of its growth throughout the years . After the data preparation and integration,McKinsey reports that its team built 15 to 20 algorithmic models which has allowed the bank to forecast revenues, the impact of Brexit on mortgage and deposit balances, and budget estimates, among others.
The case study also reports that the models have helped the bank make forecasts that would its strategic planning and business decisions for the future. It has also provided the bank with a better understanding of the various parts that contribute to the business’s growth to make informed decisions.
McKinsey also lists Swissair, Kmart, and Global Crossing as some of their past clients.
Ari Libarikian is the Senior Partner responsible for the Insurance Advanced Analytics and Data practice globally at McKinsey. He holds an MS in electrical engineering and computer science from the Massachusetts Institute of Technology. Previously, Libarikian served as Research Associate at Bell Labs and an Optical Networks Engineer at Nortel Networks.
Header Image Credit: bitrazzi.com