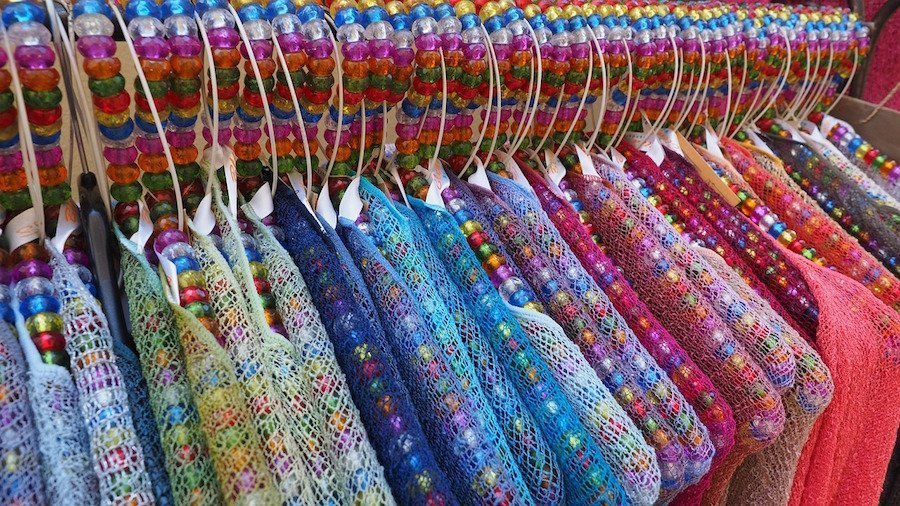
The fashion industry has grown at 5.5 percent annually in the past decade, according to the McKinsey Global Fashion Index, and in 2016 was estimated to be worth $2.4 trillion.
It has been hounded by challenges, the same report said. Sales growth slowed to at most three percent by the the end of 2016. The report also said that apparel companies have been innovating internally to reshape the industry, such as shortening the fashion cycle
Email marketing tools for clothing retailers offer personalized messaging, targeting distinct segments such as regular shoppers or inactive shoppers, as well as those that abandon carts.
Away from the fashion front, robotics have entered the retailers’ factories and warehouses to boost productivity and efficiency.
This article will highlight how applications are using AI for the strategies of:
- Marketing and Purchase Recommendations
- Market Intelligence and Trend Prediction
- Robotics in Clothing Production
The below applications for retail fashion use a variety of AI technologies — from computer vision to robotics. All still require inputs from the individual shoppers or enterprise operators, whether it is providing personal data, email address, uploading images.
Marketing and Purchase Recommendations
Affinity
One standalone recommendation engine is Affinity, a mobile app that uses machine learning and human user input regarding personal style, fit, body shape and even event attire appropriateness to suggest a variety of looks. To start, users take a survey that asks them to rate fashion items by tapping a heart or an X symbol. The company claims this process teaches the algorithms about the user’s personal style preferences and sends this data to the app’s fashion search and recommendation tool.
Once the user has signed up, she will receive weekly recommendations of curated items that match her style and preferences. Affinity follows an affiliate program business model where users click an item on the Affinity site or app, which brings them directly to the retailer’s site. When a customer makes a purchase, this provides Affinity with a cut of the retailer’s profit.
On the IOS mobile app or the website, the company presents a variety of products from athletic wear, shoes, dresses, jeans, bags, jewelry and eyewear. Among the brands available are Stella McCartney, Neiman Marcus, J Crew, Louboutin, Barneys New York and Jimmy Choo. At the moment, the app is not available on Android phones.
CEO Abigail Holtz said in an interview:
“We use technology for things like big computations and analyses — uncovering preferences, ranking and scoring algorithms, etc. The biggest issue [with computer vision and machine learning] is quality/accuracy, though it is also the case that building even moderately good models to categorize all our attributes would likely take years and require constant turning and re-training. Over time, we will utilize more and more computer vision and machine learning to replace manual human processes, but we will always prioritize solving problems well above full automation.”
Prior to founding Affinity, Holtz was a product manager at Google from 2010. It is not clear however, who is responsible for AI advancements in the company or if Holtz managed AI related projects.
From our research, we could not find any case studies relating to this company’s work with retailers.
Finery
Finery is a wardrobe management application that asks users for their email address and permission to access email receipts of fashion purchases. It then claims to be able to determine what apparel are already in the user’s wardrobe. Using this data, the company claims that its algorithms can suggest looks using the user’s existing pieces, and clothing recommendations that could match the user’s current style. According to the company, the application’s Wishlist feature recommends identifies aspects of a wardrobe that have not been purchased and can recommend items that could complete the user’s wardrobe.
The 3-minute video review of the application shows how the user can add items to the Wardrobe and Wishlist. Clicking on a product image gives the user details about the item. The application or site also features some stylists from whom the user might find tips.
Within the company, Oscar Mejia leads the machine learning development. He has a Master of Science degree in computer software engineering from Harvard University.
From the site, it is not clear which brands and retailers are clients of Finery. We could find no case studies on this product.
Hook
Hook is a search engine marketed to consumers which, the company claims, can keep track of global fashion trends, collect customer data points and find similar looking items from multiple retailers.
Developed by Intelligence Node, the application claims to use deep learning algorithms to give retailers the benefit of collecting and analyzing customer data points — specific keywords, online navigation patterns, price points on their shopping cart, actual purchases, and their “likes,” among others — to determine what users are searching for.
In addition, the application is reported to be able to track exact and close matches to their product, potentially giving retailers the opportunity to differentiate themselves, the company claims. According to the website, the application carries items from more than 5,000 brands such as Gap, JC Penny, H&M, Old Navy, TopShop and Nike.
On the consumer side, using the mobile app or online website enables them to compare prices, find similar products, receive alerts about sales, and keep track of fashion trends from hundreds of brands.
To start, users can upload an image of an outfit they want to search and purchase. The application’s algorithms accesses the online catalogs it carries and comes up with curated recommendations, as shown in the short video below. The video also compares Hook with another recommendation engine called Tailor.
Yasen G. Dimitrov is the Chief Analytics and Chief Data Officer at Intelligence Node for four years. Within the company, he leads the the data science team, responsible for operationalizing the analytics using machine learning.
From our research, we could not find any case studies about Hook’s work with any retailers. We also could not find case studies about this specific product on Intelligence Node’s website.
Vue.ai
Vue.ai, an eCommerce platform developed by Mad Street Den, claims that its AI-driven email marketing solution called VueMail offers email personalization in real time to keep customers engaged after they leave the Vue eCommerce site.
Similarly to the other applications, Vue’s site claims to allow a user to make a preference profile, while also shopping around for different products. When the user abandons a cart, the machine learning algorithm takes note of product details and sends a personalized email which recommends similar products with similar colors, patterns, cuts and styles.
The company claims the algorithms also have the capability to distinguish regular shoppers and sends personalized emails with outfit recommendations. The algorithms choose the recommendations based on products previously purchased by the shopper.
Another type of personalized email contains product promotions, aimed at increasing the chances of shoppers’ continued loyalty. According to the website, these emails are sent to inactive customers, and showcase curated recommendations and offers based on their purchase history.
The 2-minute video below shows the Vue.ai eCommerce website and explains how machine learning, deep learning and computer vision technologies work together to personalize the content for each site visitor.
According to the video, first-time users are initially shown popular brands or product categories on the homepage. The algorithms take note of the user’s behaviour such as browsing through product categories, including cameras, or brands, like Samsung. The more time a shopper spends on the site, the more data is gathered about their behaviour, which trains the AI technologies better.
In case study, Vue.ai claims that an eCommerce marketplace achieved a 5- to 15-percent increase in revenue after sending VueMail personalized emails to its customers. Another unnamed US apparel eCommerce retailer saw 46-percent increase in click-to-open rate and double the industry average revenue after implementing VueMail. The clients are unnamed, so we advise readers to take the results with some reservation.
On top of personalized email, Vue.ai by Mad Street Den also claims to offer solutions for AI-powered catalog tagging, styling and searching and is targeted at catalog managers, merchandisers, and product managers. One solution called VueTag is used to curate items in online catalogs with attributes such as category, gender, color, pattern, dress length, sleeve length and neckline.
To start using VueTag, the user first uploads the images and product descriptions to the application. The application’s computer vision algorithms have been trained to extract visual attributes of the products such as category, gender, color, pattern, sleeve length, necklines, silhouette. They can also tag the image based on textual information. Once the tags have been extracted, the program sends the tags to members of the Vue team for quality assurance. If they find that an image has been inaccurately tagged, it is sent back into the system for retraining. Finally, the tagged images are delivered to the online catalog.
Zilingo is an eCommerce marketplace that brings together fashion brands from Southeast Asia. The company used a suite of Vue.ai applications when it was setting up the website. To help its merchants streamline curation of product descriptions and images, the company says that it used VueTag. This helps the company’s thousands of sellers, who each put up 5,000 to 10,000 products per month.
The client claims that the application has made the process simple for its sellers as it eliminates the need for a human army to type product details manually. The client added that this makes curation not only faster but also more accurate. “Shoppable videos and auto-tagging have made our lives significantly better,” said Zilingo’s CEO Ankiti Bose.
The company lists Levis, Macy’s, Diesel and Cosabella as among its clients.
Costa Colbert, Chief Science Officer at Mad Street Den, has Master of Science degrees in electrical engineering and computer science; as well as biomedical engineering from Johns Hopkins University. He also holds a Doctor of Medicine in neuroscience from the University of Virginia.
Market Intelligence and Trend Prediction
Stylumia
Stylumia is a market intelligence application which the company claims can attempt to predict fashion trends with the help of deep learning and AI technologies. According to the company website, the application is able to take unstructured computer vision, natural language processing, behavior data and converts it to fashion insights.
This application is targeted at clothing and apparel manufacturers, brands, retailers or fashion and lifestyle influencers who could potentially use the insights to make informed decisions and insights about future fashion trend.
To use the application or website, a retail client can log in and search colors, products or styles. The company claims that its the application can then collect photos a retailer’s eCommerce sites, text descriptions on social media and customer behavior data to show the client a dashboard of data and visualized graphs centering around a product or style they’ve searched. The company claims it can also use these analytics to offer a future outlook graph visualizations and suggestions of whether a certain fashion trend will be successful.
An example of a use case for this application would be at a fashion show. In a blog post on the Stylumia website, the company describes how the application can extract data from major fashion events where 40 designers could present a total of 24,000 outfits for one season.
Stylumia’s blog noted that the application also has the capability to capture images of clothing using a computer vision-enabled lens. While the fashion show is ongoing, the application’s algorithms will start grouping images of similar clothing items by color and shape: animal print dresses, deconstructed kimonos and coats, black-and-red themed clothes, and other similar trends at the event.
According to the company, this data can then be analyzed by fashion show organizers and designers who need to discover the trends and make predictions for the coming season.
Another use case described by Founder and CEO Ganesh Subramanian is for text-based keyword search by designers or clothing manufacturer. The user can describe the style they are looking for, for instance “yellow and purple striped shirt.” The application’s natural language processing algorithms will crawl through the web to find images of the item.
The company claims that the machine learning application can recognize trends on social media sites such as Instagram and Pinterest, and can be trained for colors and silhouettes. It will also search for the correct items even though the keywords are spelled incorrectly.
The application’s computer vision technology can distinguish the right color of items, having been trained in in the exact attributes and color values of the RGB (red, green, blue) system.
Subramanian also claims that the algorithms can crawl through videos, determine the color of garments through optic mapping using deep learning neural network, and extract a color histogram of the garments in the video.
In a case study, an unnamed client wanted to design a T-shirt collection at the beginning of 2017, using designs launched in past two months by local and international brands as inspiration. The client specified the top 10 percent best-selling designs and Stylumia returned with designs ranked according to popularity. The designs included camouflage print, contrast-colored pockets, round neck raglan and horizontal stripes.
The designer then combined these trends, and received approval from the business team to produce a certain quantity after seeing the rankings provided by the application. The case study claims that the shirts sold out within three weeks at five marketplaces by the end of the season.
The company claims that has 40 clients including Aeropostale, Myntra, Wrogn, Fastrack, W, Biba, Global Desi and Puma.
At present, none of the company’s executives have deep AI expertise. Abhishek Thakur, Chief Data Scientist at boost.ai, serves as AI advisor to Stylumia. He holds a Master’s degree in computer science from the University of Bonn.
Robotics in Clothing Production
Grabit
Grabit claims its robot called Stackit is an automation system that uses robotics, machine learning, computer vision and algorithms to automate shoe production. The company says that the robot’s machine learning algorithms are capable of layering items 20 times faster than humans.
Its hands are fitted with an electroadhesive gripper that produces static electricity that can handle materials such as leather; meshes and composite fibers; and other materials thinner than one milimeter. It can also handle boxes that weigh up to 50 kg.
Stackit is designed to work with factory employees. The computer vision algorithms determine how the materials should be stacked, and lights up portions of a connected glass table where the factory employee should set products down. The robot’s electroadhesive platform picks up the materials, while computer vision-equipped cameras monitor the progress. The machine can also move over to a partially finished shoe shoe and drop it into a heat press.
The short video below demonstrates how Stackit can handle various types of materials from metal to fabric, glass and printed circuit boards, among others. The robot’s gripper uses electroadhesion technology, which is adhesion of two surfaces when they are subjected to static electricity.
The company has raised $25 million in funding, with Nike among its investors.
The company has no official case study, but a news report claims that assembling a pair of Nike shoes involves stacking up to 40 pieces of material to create the shoe upper, a process that takes a human worker up to 20 minutes to arrange. Using Stackit, the report claims this process will take 20 times faster, at a rate of 50 seconds per shoe upper or 300 to 600 pairs of Nike uppers in eight hours, machine learning. At this pace, the report claims that Nike will receive its return on investment in two years.
Aside from shoes, the company website claims that the robot can also be applied in manufacturing processes of apparel, automotive and aerospace. A Nike press release reports that Grabit’s current customers include some Fortune 500 companies in industries such as warehouse automation, parcel handling, consumer products, and textiles. Current investors include Formation 8, Draper Nexus, Danhua Capital, Nike, Samsung, Brother Industries, ABB, Shanghai Electric, Flex, NTT Docomo and the Esquel Group
Harsha Prahlad, is the Co-Founder and Chief Technology and Products Officer at Grabit. He previously led the Advanced Components Group at SRI International and invented Artificial Muscle, Inc., acquired by Bayer Material Science. He received his Ph.D. and M.S. in aerospace engineering from the University of Maryland, and his B.S. in mechanical engineering from National Institute of Technology in Trichy, India.
SewBots
SewBots by SoftWear Automation is a computer vision-powered robot that sews footwear, t-shirts, pillows, bath mats, automotive mats and towels, according to the company. The company website explains that the robots are designed to stabilize and sew cloth and other materials by “mapping” the surface of the material as it sews.
Sewbot’s computer vision algorithms detect distortions in the fabric and adjusts it robotically. The computer vision also guides the robot in cutting, sewing a seam, adding a sleeve, and inspecting the quality of items across a 70-foot long t-shirt production line. The sewing process move the needle rather than the fabric moving through a stationary sewing machine.
The robot’s machine vision system is capable of tracking exact needle placement to within half a millimeter of accuracy, the company claims. Its image processing algorithm-driven camera can capture more than 1,000 frames per second to view each thread, the CEO added.
The Atlanta-based machine vision and robotics startup spun out of Georgia Tech after seven years of research and development working on projects with DARPA and the Walmart Foundation.
CEO Palaniswamy Rajan describes the robot’s sewing process like that of a human seamstress,
“The first thing they do is use their eyes, and based on their eyes, they do micro and macro manipulations of the fabric with their fingers and hands and elbows and feet. So a robot replicates all of those functions.”
The video below has former CEO KP Reddy explains how the robot sew a buttonhole using computer vision cameras at the top and bottom of the fabric:
At the moment, Sewbots are limited to mass-producing simple pieces that require limited sewing skill such hemming and binding. According to the website, the machines are able to produce one T-shirt in 22 seconds. The footwear upper workline can produce one sewn upper in 26 seconds.
One client is Tianyuan Garments Company, of Suzhou, China, which mass produces shirts for Adidas worldwide. The company also counts among its clients Avery Dennison: A company which offers pressure-sensitive, functional materials; branding; weaving and labelling solutions for the retail apparel market.
CTO Barrett Clark oversaw the development and deployment of Sewbot. He obtained his Master’s degree in mechanical engineering from the Ohio State University.
Concluding Thoughts
From our research, we are seeing that many marquis fashion retailers have given payments, such as a cut of purchase, in order to have their products featured and sold on eCommerce sites with recommendation engines. This seems to be the case when looking into applications like Hook and Finery. Through other research, we have also seen that many retailers have brought third-party built recommendation engines exclusively to their own websites.
We are also seeing a growth in robotic design and design trend prediction applications, which may correlate with how marquis companies like Amazon and Facebook are both experimenting with AI technology to design clothes.While IBM has also partnered with Tommy Hilfiger and the Fashion Institute of Technology, Google presented its line of clothing via Project Muze.
However, it may take a while for design work to leave the human hands and passed to robots, according to the MIT Technology Review, as humans still have the stronger edge when it comes to innovation. With robotic applications noted on this list, we’ve similarly seen that the robots detect and manage simple needed tasks, while still requiring humans to handle complex design strategies.
Header image credit: Pixabay