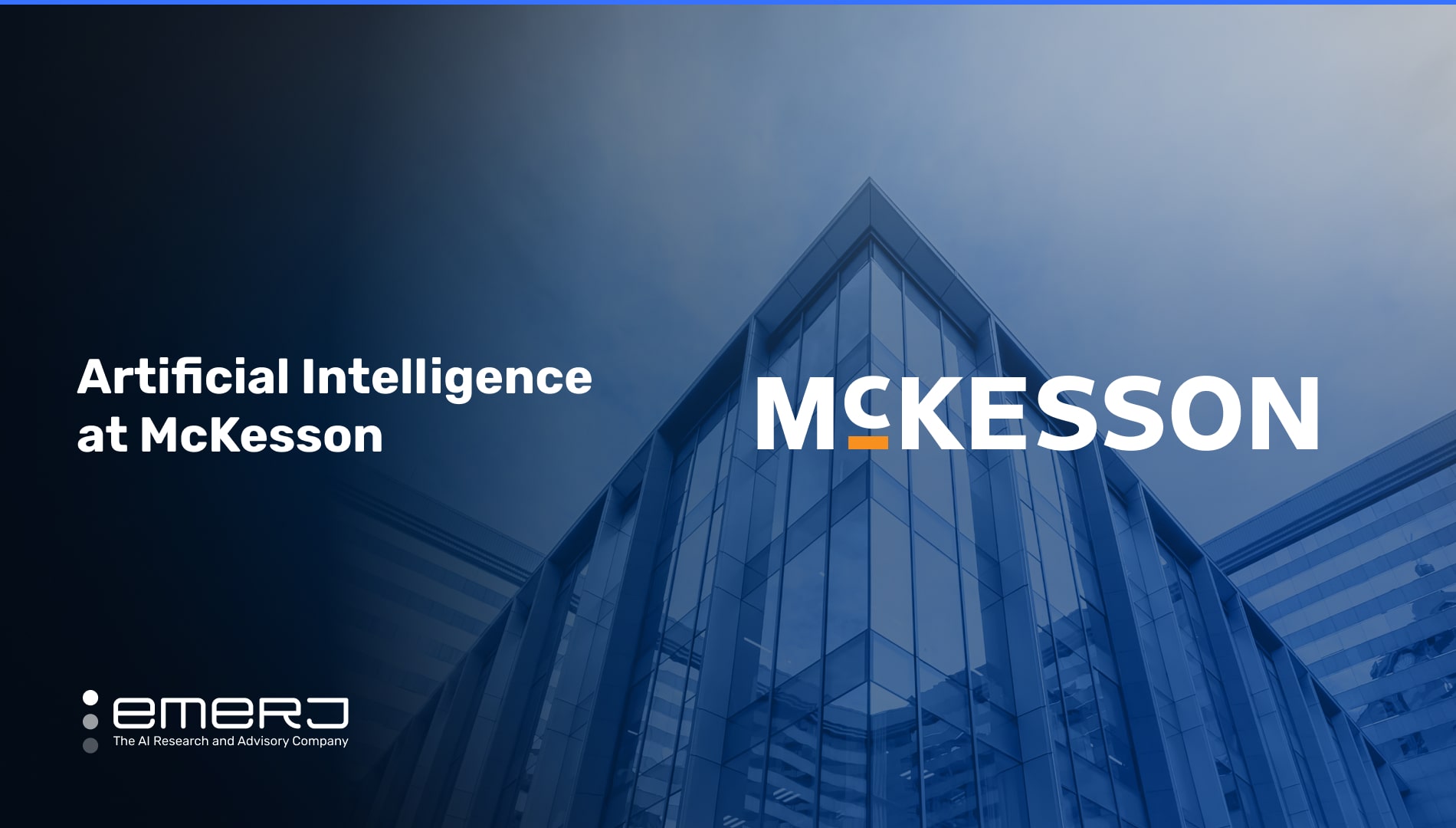
Founded in 1833 and headquartered in Irving, Texas, since 2019, McKesson is a leading distributor of pharmaceuticals and medical supplies. They also provide health information technology and care management tools.
In 2022, McKesson reported revenue of $264B, representing nearly an 11% increase from the previous year. McKesson’s customers include retail chains, independent retail pharmacies, hospitals, health systems, integrated delivery networks, and long-term care providers.
McKesson has implemented AI solutions by partnering with providers, acquiring companies, and investing in companies through their corporate venture fund. In 2019, Emerj reported on various AI initiatives both in and outside the enterprise. This article extends on that reporting and examines three use cases demonstrating how McKesson currently uses AI to diagnose diseases, improve and expand contact services, and pack pharmaceutical shipments:
- Improving contact services: Leveraging automation via intelligent virtual assistants powered by conversational AI to extend customer support and improve existing call flow.
- Keep delivery promises for pharmaceutical shipments: Using robotics to process and pack orders while alleviating staffing shortages in distribution centers.
- Diagnosing disease: Train machine learning models using an analytics-powered healthcare map to help doctors diagnose illness in current patients and also diagnose cancer earlier and with more precision.
Use Case #1: Improving Contact Services
Operating expenses for call centers in the healthcare industry depend on performance on a number of metrics. These metrics include:
- Average handle time
- Average call transfer rate
- Abandoned call rate
According to one study, the cost-per-call for maintaining an internal on-premise call center for one particular specialty practice in urology was $4.02 per call. A pharmaceutical business intelligence firm found that well-known drug companies earmark $3.2 million on average to an individual medical information call center.
McKesson wanted to improve customer service, expand their contact center availability, and better meet service delivery requirements. McKesson considers contact services as a vital part of their offerings primarily because of the department’s role in supporting patient medication.
McKesson enlisted the help of Five 9 to update their contact center. At the start of the overhaul, they had to quickly onboard human agents. In fact, McKesson staffs its contact center with an additional 2,000 agents to meet a 3-month period of seasonal demand. Their plans include adding IVAs at existing points within the current call flow.
The role of IVAs will be to automate use cases, including prescription refills. This is an example of eliminating a redundant task in order to free up time to allow contact center agents to focus on customer interaction for issues that require a more personalized approach.
Screenshot showing the voice portion channel of Five9 IVA workflow (Source: Five9)
Five9’s cloud-based call center solution offers benefits over traditional legacy, on-premise call center solutions. Before implementing IVA, essentially every customer inquiry required input from a live agent. Five9 IVA utilizes multiple types of AI to achieve call resolution effectively by using:
- Natural language processing
- Sentiment analysis
- Speech recognition
- Text-to-speech capabilities
McKesson also plans to adopt Five 9’s Agent Assist technology, which will provide help to contact center agents in real-time to improve customer engagement.
Before deciding to implement IVA, McKesson experienced a high average for both handling time and call abandonment rates. These issues were further compounded by a labor shortage resulting from difficulty with both recruiting and retaining contact center agents.
While the initiative is in progress and outcome data is not finalized, McKesson has seen the following benefits:
- Deflecting more than 40,000 calls per month during a 10-month period
- $2.2 million annualized savings
Use Case #2: Keep Delivery Promises for Pharmaceutical Shipments
McKesson wanted a way to lower the costs associated with distributing medications as a pharmaceutical wholesaler. The margin on generic drugs is a crucial source of profitability for wholesalers. Still, intervention from policymakers has put downward pressure on generic drug prices, reducing that margin as a result. McKesson also needed a way to address the labor shortage they experienced since qualified personnel were in short supply.
The pharmaceutical industry faces several challenges when distributing medications, including:
- Quick turnover of medicines within very short time frames
- Initiating multiple shipments per day to pharmacies
- Quickly fulfilling home delivery of medications
It was fortunate timing that Covariant came out of stealth mode in January 2020, just ahead of the pandemic. However, McKesson was already at the forefront of robotics. In 2010, McKesson signed on four leading acute-care hospitals to use their robotic medication dispensing and integrated pharmacy automation solutions.
McKesson is one of the three leading wholesalers that distribute prescription medications; the top three wholesalers account for more than 90% of all wholesale drug distribution in the US. Wholesalers’ business practices have a significant influence on which drugs are distributed to patients.
McKesson decided to use the Pick-it-Easy Robot by KNAPP to compensate for their worker shortage. KNAPP provides intelligent automation solutions for intralogistics and manufacturing.
KNAPP’s robot is powered by Covariant’s universal AI platform, known as the Covariant Brain, which enables the robot to recognize many different features of pharmaceutical products. McKesson is able to process orders automatically.
This video from Covariant, running just short of four minutes, offers a demonstration of the Covariant Brain platform and workflows.
Screenshot of the Covariant software dashboard identifying items in a warehouse repository for robotic transport. (Source: Covariant)
Additionally, Covariant Brain uses fleet learning, which allows an individual robot to learn from other robots it’s connected to. Robots could be across the globe from one another, but any particular piece of knowledge one robot learns is distributed to all other connected robots.
In a 2022 press release, Vice President of Strategic Distribution and Operations at McKesson, Todd Kleinow explained, “US medicine packaging is very complex, posing a great challenge to fully automatic robotic picking. Getting there was not exactly easy, but today, we can pick a large part of the product range with the Pick-it-Easy-Robot.”
While there are no exact figures publicly available to show the impact using robots has on McKesson’s bottom line, implementing automation in their distribution centers to achieve increased speed and allows McKesson to remain competitive.
Since deploying the Pick-it-Easy Robot in their distribution centers, McKesson is more easily able to keep their delivery promises and comply with the legal requirements of the Drug Supply Chain Securities Act (DSCSA).
Use Case #3: Diagnose Cancer Earlier and More Precisely
Achieving earlier and more accurate diagnoses provides patients with better care via both more effective treatments and better outcomes. Treatment costs are reduced as well. Cancer diagnosed in later stages is more costly to treat.
According to one study, the per-patient, per-month healthcare costs for patients diagnosed with lung cancer are the highest for patients diagnosed at Stage 4 and lowest for patients diagnosed at Stage 1.
McKesson believes that they can detect cancer much earlier, and they’ve committed to doing so. Through their venture capital arm, McKesson Ventures, they have invested in Komodo Health. They use Komodo Health’s AI platform to diagnose cancer earlier and more precisely.
Founded in 2014, Komodo’s primary aims are to:
- Accelerate disease research and drug development
- Strengthen real-world evidence studies
- Unlock digital health innovation
- Offer patients new therapies
Komodo Health is trying to solve the problem of cancer diagnosis by using data analytics and AI to analyze the medical journeys of more than 300 million patients. Komodo Health is training its algorithms to detect cancer before symptoms are evident, and they’re learning by analyzing 50 billion clinical encounters.
Previously, healthcare companies needed help to utilize their data effectively. Legacy systems relied on the use of spreadsheets or insurance claims databases and aggregating claims contained in those sources. This static approach was ineffective because, by the time stakeholders were provided with information, it needed to be updated.
The legacy approach also fails to identify hidden links in healthcare data. There are patterns and insights that a siloed, static approach cannot uncover.
Komodo Health created its Healthcare Map as a solution to the above issues. The Healthcare Map uses both advanced data analytics and AI for each patient’s individual interactions with the healthcare system, and it does so for 325 million patients. The care that is evaluated might have occurred in or out of network, at a specialist’s office, an urgent care center or a retail clinic. The Healthcare Map serves as the basis for Komodo’s cloud-based analytic software solutions.
Screenshot of the Komodo Health Map dashboard. (Source: Komodo Health)
Komodo presents insights gleaned from its analytics platform in the form of fast fact sheets. Their Fast Facts on lung cancer sheet highlights are derived from data available on Komodo’s Healthcare Map.
Komodo provides insights to drive change for patients by guiding the work of:
- Federal and state agencies
- Policymakers
- Academic institutions
- Payers
- Drugmakers
- Advocacy organizations
These fast fact analyses provide healthcare stakeholders with detailed insights derived from real-world data to help them identify effective strategies to combat various forms of cancer.
McKesson also uses Komodo’s healthcare analytics and data platform to discover correlations between symptoms of diseases. Millions of patients’ medical records are analyzed; those medical records are derived from the following sources:
- Doctors
- Diagnostic labs
- Prescription data
The platform is powered by predictive analytics tools that enable doctors to both monitor and predict disease progression in their patients.