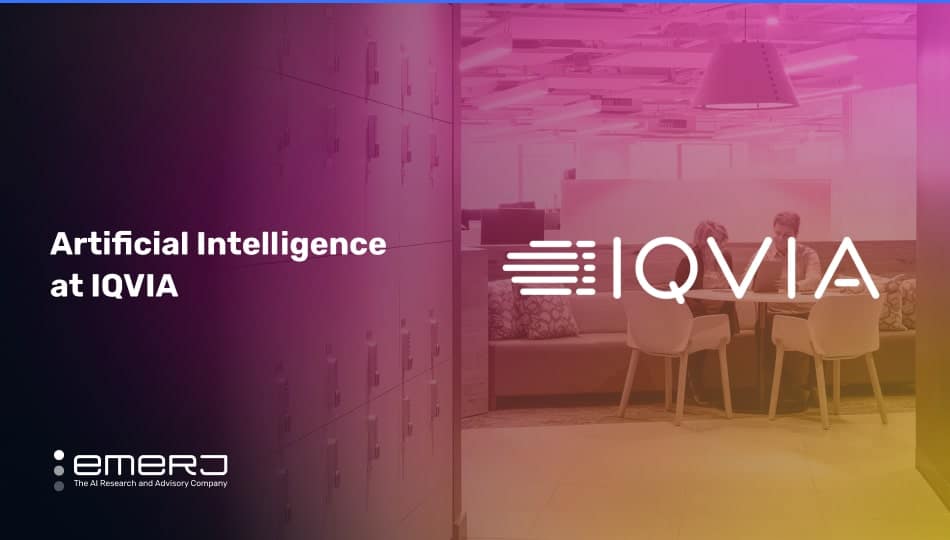
IQVIA is a Fortune 500 health information technology (HIT) and clinical research company that provides advanced analytics, technology solutions, and clinical research services for various healthcare stakeholders.
The company produces a suite of technologies designed to help life sciences organizations accelerate innovation, improve efficiency, and continuously bring new treatments to markets globally. IQVIA’s primary services are biopharmaceutical development, professional consulting, and commercial outsourcing, with a particular focus on Phase I to Phase IV clinical trials and associated laboratory services.
IQVIA’s 2022 annual report cites revenues of $14.41 billion. The company is traded on the NYSE (symbol: ‘IQV’). Its annual report claims a workforce of 86,000 employees across more than 100 countries.
The role of AI and machine learning at IQVIA is significant and multifaceted, with the technologies leveraged to enhance various aspects of healthcare delivery and pharmaceutical development. These areas include drug discovery, development, and safety; patient value and treatment alternatives; and business and operations (e.g., manufacturing efficiency, healthcare provider engagement, sales and marketing optimization).
In this article, we examine two timely, actionable use cases for life sciences executives:
- Skilled talent identification and acquisition: Using machine learning algorithms, natural language processing, and predictive analytics to expedite skilled talent acquisition in areas of front-end interface design and CRM development, expediting product design and delivery while reducing costs.
- Predicting patient eligibility for early treatment: Using machine learning algorithms to more efficiently identify and predict prospective patient success for primary and secondary treatments, dramatically improving HCP engagement and increasing efficiency in patient identification and transition rates.
Use Case #1: Skilled Talent Identification and Acquisition
Per the case study published by its partner BairesDev, IQVIA was facing a talent shortfall in several operational and technical areas (unsurprisingly, a tech talent shortage remains a problem globally.) The company required additional skilled talent to achieve operational flexibility, scale critical products, and improve digital assets.
To assist the company in finding the talent necessary to help IQVIA scale its critical products, BairesDev used a proprietary “AI-powered team-building suite” called Team Recommendation Engine. According to BairesDev, machine learning and complex algorithms power the engine to match engineers to IQVIA’s required skills and qualifications.
The company is rather vague on the workings of its proprietary recommendation engine. Still, we can deduce the likely AI technologies used based on research and well-established precedents for AI-driven recommendation systems:
- Genetic algorithms: to enhance the recruitment process by iteratively refining candidate selections using evolutionary techniques to match job criteria optimally.
- Machine learning algorithms: to analyze vast amounts of disparate data such as candidate information, job requirements, and project goals.
- Natural Language Processing (NLP): to parse and understand job descriptions, process requirements, and candidate qualifications.
- Predictive analytics: to forecast job performance and fit by analyzing historical data and identifying trends that correlate with success in specific roles.
The data inputs into BairesDev’s Team Recommendation Engine likely include:
- Candidate information: data such as resumes, portfolios, professional profiles, and any other data candidates may submit.
- Historical data: past performance data of candidates in similar roles or projects that could be used to inform the candidate matching process.
- Job descriptions: detailed descriptions of roles, including required experience, skill levels, and educational qualifications.
- Project requirements: specific needs for the project(s), such as domain expertise and technical skills.
The primary output of the system is a curated list of engineers that the tool determined to best fit the project. Prior to the implementation of the platform, it’s likely that project managers manually reviewed and matched engineer profiles to project needs.
Regarding business outcomes, BaireDev’s case study highlights the effectiveness of the system in addressing talent shortages. The study states that the proprietary software was able to match the top 1% of job candidates with IQVIA’s talent needs.
Use Case #2: Predicting Patient Eligibility for Early Treatment
IQVIA’s enterprise client aimed to encourage the early adoption of their primary and secondary oncological treatment for patients who might not respond well to later-stage therapies. The clients also wanted to target the patients at the highest risk of failure at the late stages of treatment.
IQVIA reportedly integrated machine learning algorithms with its Real World Data (RWD) portfolio for the solution. Typically, algorithms enable the rapid and precise processing of large volumes of patient data.
According to the case study report, leveraging these algorithms accelerates the identification and linkage of patients and healthcare providers (HCPs), expedites patient initiation by targeted HCPs, and swiftly facilitates the monitoring of treatment transitions. The solution may also enhance the prediction of treatment success for patients who have yet to be diagnosed.
Input data stated or implied in IQVIA’s case study include:
- Large datasets from IQVIA’s RWD portfolio, including patient health records, treatment histories, diagnostic information, and HCP interaction and communication data.
- Conditions and parameters for patient segmentation, including demographics, disease type and stage, treatment history, risk factors, genetic information, behavioral data, clinical outcomes, socioeconomic status, etc.
Stated or implied outputs of the solution include:
- Lists of identified and segmented patients
- Relationships between patients and HCPs
- Insights into the patient journey and treatment transitions
- Predictive models identifying potential future patients
IQVIA’s mention of increased speed and accuracy, along with dramatic improvements and reported business outcomes (below), suggests a transformation in end-user workflows. Prior to solution implementation, the client likely relied on a slower (e.g., manual, semi-automated, or disparate/siloed analytic methods/systems) means of data analysis, resulting in slower processing times.
Additionally, a limited ability to predict future patient cases or understand complex conditions is implied, as is slower commercial success due to inefficient patient journey insights.
A screenshot of the case study report PDF page showing how the AI system was able to improve patient outcomes over a business rule approach. (Source:IQVIA)
Results-wise, IQVIA reports the following outcomes:
- 15x increase in patient identification precision
- 10x increase in HCP linkage precision
- 7x improvement (28% to 4%) in treatment transition rate over three months compared to prior rules-based model
- Approximate 7x (41% to 8%) improvement in successfully predicting which HCPs would begin treating a patient within three months.