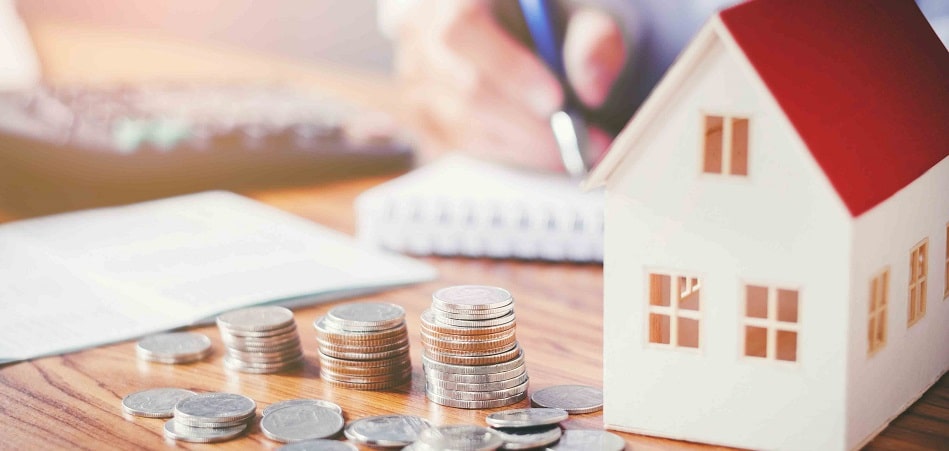
AI may have a role to play in digitizing the paper-heavy mortgage process, facilitating more streamlined search and discovery for entities across a variety of digital and scanned PDF documents. We spoke with Dan Cortright, Senior Director of Product Management at Iron Mountain, about just that. Courtright discusses how AI could help approve loans quicker, better assess risk, and allow employees to pull up documents they need to respond to customer requests.
Listen to the full interview from our AI in Banking Podcast below:
Subscribe to our AI in Banking Podcast with your favorite podcast service:
Guest: Dan Courtright, Senior Director of Product Management – Iron Mountain
Expertise: Document management strategy and operations.
Brief Recognition: Prior to Iron Mountain, Courtright was VP Program Management of Mr. Cooper’s Titan Project. He spent time at PwC and holds an MBA from The University of Texas at Austin.
Interview Highlights
(2:30) Where does AI play a role in search and discovery in mortgage today?
Dan Courtright: The first thing that pops in my mind for search and discovery, and I’d say lending, is LIBOR. So LIBOR is a benchmark index that’s going to be retired in a couple of years at the end of 2021. One of the big pieces is understanding a bank‘s or lender’s exposure to any contract that has that language in it. So if…there’s a piece of a contract that’s going to be retired to go away, a company has to understand what their exposure is.
To do that, they need a way to search the content that they have. The best [way] is AI and machine learning. I’m running through their content to figure out one, what contracts use that language, and two, what’s that fallback language? Where’s their exposure, and how do they mitigate that exposure?
It gave me an expanded-upon mortgage, but it’s any sort of contract where there’s going to be an adjustable rate on that interest. And so if that contract is pegged on LIBOR and that contract has a maturity date or is going to extend past 2021, that bank will have exposure.
So they’ll need to do some research to figure out what they do and what new benchmark they want to use. But it’s any contract that was written with a borrower where they have an adjustable rate typically and [where] they’ve used LIBOR [as a benchmark].
LIBOR was the most popular. I think today it still is. But people are gradually moving away, whether it’s a US-based contract or a Europe-based contract. Each kind of went their own separate ways.
(05:30) What’s the impetus for search and discovery technology as it relates to LIBOR?
DC: Each contract generally has fallback language…With that fallback language, it’s understanding. What does it mean that the index isn’t available?
And so typically a firm will have to get their legal team involved to understand, “okay, based on this language, this is what we should do. We have to create an agenda. Maybe we have to send a notice to a borrower.”
But the financial risk is I think two parts. One is from a borrower’s perspective. Do they fear other damages associated with that changing of the index? So I’ll make something up.
Let’s say they moved from LIBOR or to some other index and for some reason that cost the borrower a lot more money. There’s substantial damages. Most likely the borrower is going to file some sort of litigation against that company if they aren’t happy with what that is.
The other piece is even just from a regulatory perspective. In Europe they even said…they potentially might start fining or tying some of these institutions if they aren’t gradually moving away from that index with their contracts. So there is kind of this not borrower-initiated but more of a regulatory issue of fines if they’re not seeing the adoption and move away from LIBOR.
And I think it gets pretty complex. Again, I’m from lending, but when you start ignoring the investment channels and assets like credit default swaps and all these things are bundled together. When you start talking about securities and how they’ll trade on the market, that if there’s LIBOR involved, what does that mean?
I don’t think we know yet really. It’s far away. The new indexes they want to move to haven’t been truly established yet. But just back to the AI and machine learning, getting a grasp on the exposures where it can be used. It’s the OCR being able to have that information at your fingertips of what a machine was able to pull out from your content or your contract.
So one is there’s not a consistent fallback language in a contract, but it’s pulling out those individual entities, pulling out the field level information from those contracts.
What’s the borrower’s name? What’s the loan amount? It’s specific language that they’re looking for, but being able to isolate that and train the system to pull from it based on everybody’s own kind of contract repository.
(09:00) Where else can AI for search and discovery be valuable when it comes to mortgages?
DC: I’d say that the next use case that really could pop to my mind is…when a bank or servicer are trying to recoup funds from an investor based on what they paid. And so what generally happens is that it just requires a lot of searching of content and understanding, basically, “What invoices do I have support for?” It’s almost like submitting your own claim. Even if you had your house need an insurance claim on a roof or something.
You have to provide support. You have to search through this wide array of documents to one, find the invoices or supporting documentation, and then also filter through that as well.
Maybe you’re wanting to search by the invoice amount, but it’s really focused on the invoice side. Having a machine learn an algorithm to one, turn that content into some information to the OCR, and two, pull those individual entities out and train it.
Again, having that information at your fingertips to search for it and file a claim. Obviously that’s done in mass, but rather than having someone have to actually manually just look at all these documents.
(10:30) What does the process of labeling these documents beforehand look like?
Training data in AI is always a hot topic because typically you need a lot of it. And getting a lot of data can be expensive because that data has to be correct. The data you feed into it must be right or else you’ve kind of wasted some time there.
But with that you can have to do it manually upfront, right? It’s looking at the invoices, a good sample, a good variation, making sure the information is correct and getting that high vine.
Now typically what I’ve seen or what you can do is start with a low sample set, and then if you have a good exception management process, feed those good pieces that you’re getting through the exception management back into the system.
The system will basically self-heal and get better and better. So if you have a really solid exception process, you can start actually with a very limited small amount of data. As long as you have enough people or manpower to really process those exceptions in an efficient and very accurate way, that system can get trained up pretty quickly.
I think in lending, I don’t think an AI or machine learning system will ever be perfect. I think there will always be required some sort of workflow or exception management. There’s so many documents, and it can get so messy, and I just don’t see that happening. Potentially in the future, when maybe everything is using a smart doc, meaning that the data is embedded. We might be able to get away from it. But right now, I think for a considerable amount of time, there will always have to be an exception management process. AI, it will never be perfect.
(13:30) What future capabilities of AI search and discovery for lending are you looking forward to?
DC: So I don’t know if I’d say I’m going to be looking forward to this because it can be related to risk and audit. But in banking in general, there’s just a lot of regulation, a lot of oversight and people, or staff, or operations having to react to questions or maybe just questions about their information.
I think leveraging AI and machine learning technology will be able to answer those questions quicker. Having the information right there. I’m picturing like Iron Man, right?
You ask a question; you have everything ready for you. I think that we’re pretty far from that, but being able to allocate time that would have otherwise been spent answering or trying to address risk-related items can be spent elsewhere because you have all of your information in the content and what you need at your fingertips.
(15:00) When would the 360-degree customer view come in handy in risk-related decisions?
DC: 360-degree customer feedback. The one thing that I think of is joining all that information into one area, and I think that’s where today we struggle a lot. It’s the integration point, that we have technology, and it’s advancing so quickly, with machines and the amount of horsepower we have with processing, but especially in the banking world, they are still using a lot of legacy technology.
So some of their information is kind of locked up in these areas that are very hard to access, and they’re not hard to access because they want it that way. It’s because of the technology.
So until we kind of break through that and have really strong, easy integration to some of these other systems, it’s going to be really hard to get that 360-degree view of anything. It’s just really hard to integrate.
Subscribe to our AI in Banking Podcast with your favorite podcast service:
Header Image Credit: I95 Business