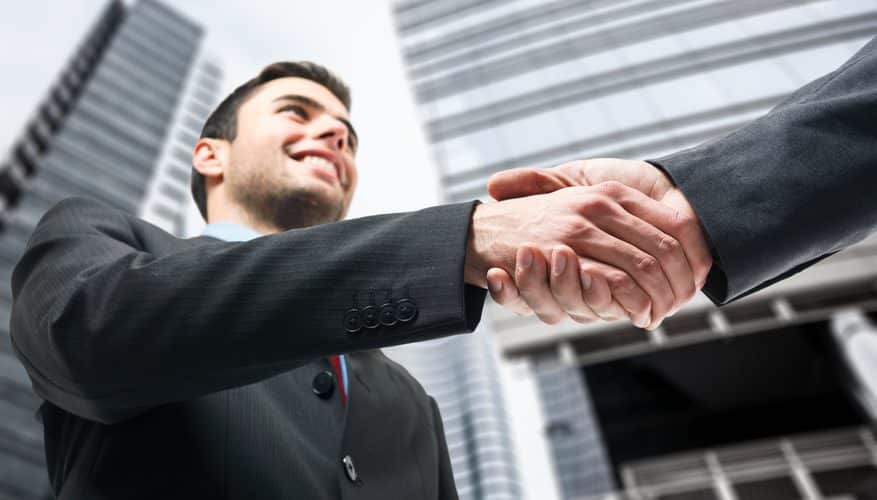
Episode Summary: Prominent technology companies like Google and Amazon lead the way in the B2C world, having access to streams of searches, clicks, and online purchases. They have access to large volumes of consumer data points numbering in the billions that can be used to train machine learning algorithms.
A typical B2B company, on the other hand, might at most make a couple hundred sales per year, and many B2B companies make only dozens. In other words, every sale matters.
In this episode of the AI in Industry podcast, we interview Kiran Rama, Director of Data Sciences Center of Excellence at VMWare, about “propensity to buy” machine learning models and which B2B companies might benefit from them in terms of determining which leads or past customers to whom they can sell. Rama also talks about how B2B companies purchase external or third party data on which they can train these models.
We hope that this interview can help business leaders determine if and how AI can help their organizations identify which leads could yield the highest ROI and which customers are the most primed for reselling.
Subscribe to our AI in Industry Podcast with your favorite podcast service:
Guest: Kiran Rama, Director of Data Sciences Center of Excellence – VMware
Expertise: Deep learning, neural networks for machine learning, data science, computational finance, Adobe Insight, Base SAS
Brief Recognition: Rama holds a PhD in Information Systems from the Indian institute of Management in Lucknow, India. At VMware, he is responsible for driving data science projects that impact sales and marketing among other aspects of the business. Prior to VMware, he served in management roles at Dell, Amazon, and Flipkart. Earlier in his career, he worked at Motorola and Cognizant.
Interview Highlights
(03:30) Explain to us the how the “propensity to buy” model works and why VMware leverages it?
Kiran Rama: The “propensity to buy” model, in its simplest form, is the ranking of customers and their likelihood to buy. The ones at the top are more likely to buy than those at the bottom. However, if you only have 10 customers, you won’t need a propensity model because you would have to pursue all 10.
If sales wants to target a subset of customers or if the customer set is so big and the sales team has limited capacity, that is the time to use the model. For instance, VMware has half a million customers on the VSphere product, our flagship product. We also need to sell our other products, but most of the time, these will be customers that already have VSphere. So how do we go about it?
The “propensity to buy” model uses machine learning techniques and historical data, such as bookings, orders, size of the company, number of employees, and their likelihood to buy from you. This allows the salesperson to focus on, for example, 2,000 accounts. Without this model, the company will use its own business rules.
(07:00) What are the critical factors that affect the likelihood of a B2B company to make a purchase?
KR: One of the buckets is order history. The company hat has bought recently, frequently, and bigger in terms of value is most likely to buy. The stronger the B2B relationship, the more likely to buy.
Second is going to be company firmographics.
The third factor is the customer’s response to marketing campaigns. Those that respond to the campaign are more likely to buy. It also gives the sales and marketing people a chance to learn the customer’s job title, the role they play in the company, and how they influence the customer’s buying decision. Their positive response to a customer survey is also a factor.
Third party or external data about this prospective company also helps, as well as product lifecycle.
(10:30) Third-party data about customers seems to make the “propensity to buy” model more robust. Is it common practice to buy external data?
KR: Yes. They even use external data to update or clean their roster. External data, such as that from Dun and Bradstreet, provides a rich dataset that includes number of employees, sales, headquarters, and other important company information. So most B2B companies will use some form of third party data or external data or publicly available information.
(12:30) How can a business define a type of customer that would deliver the most value to the business and would be the basis to build the “propensity to buy” model?
KR: In building a “propensity to buy” model, it is best to take it from a product level because this data can provide you with sales history. As the product life cycle changes, the nature of the buyers will change.
(15:15) How do you determine which product to spend the most resources on, such as time, buying external data and training algorithms, etc? Do the highest priority products receive the most attention?
KR: Definitely. This criteria is used by sales and marketing teams and top organizations. Ultimately, the model ranks the customers likely to buy, allowing the business to focus on, say, the 50 top customers.
The biggest challenge is demonstrating the results. Control tests are very important. Give about 10 sales people and each give them a subset of test accounts from the model as well as control accounts which would not be under the model. Run the test for three to six months to allow customers time to make decisions. Look at the results afterward and the business could discover that the model works.
But as a matter of standard, we don’t do this. It’s best to maximize sales, pursue all leads on the roster, and give the model the chance to learn.
(18:00) This model could be the standard for the larger companies to better prioritize and better predict the sales propensity of customers. How will this be accessible to more companies and how could it change the business landscape?
KR: There is a big opportunity for B2B companies of all sizes to leverage machine learning. There is no need for massive teams. One or two good data scientists to build the model will do. B2B companies could also benefit from other models such as propensity to respond or to sell for partners, among others. The opportunities are limitless.
(20:10) Combined with the data, you mentioned that finding talent whether internally or externally will be the crux to make things happen.
KR: Aside from that, companies also need to have a mechanism to store historical data. Good data management practices will make the models richer and better to benefit sales and marketing. Rather than use intuition, they can use machine learning.
Subscribe to our AI in Industry Podcast with your favorite podcast service:
Header Image Credit: Bizfluent