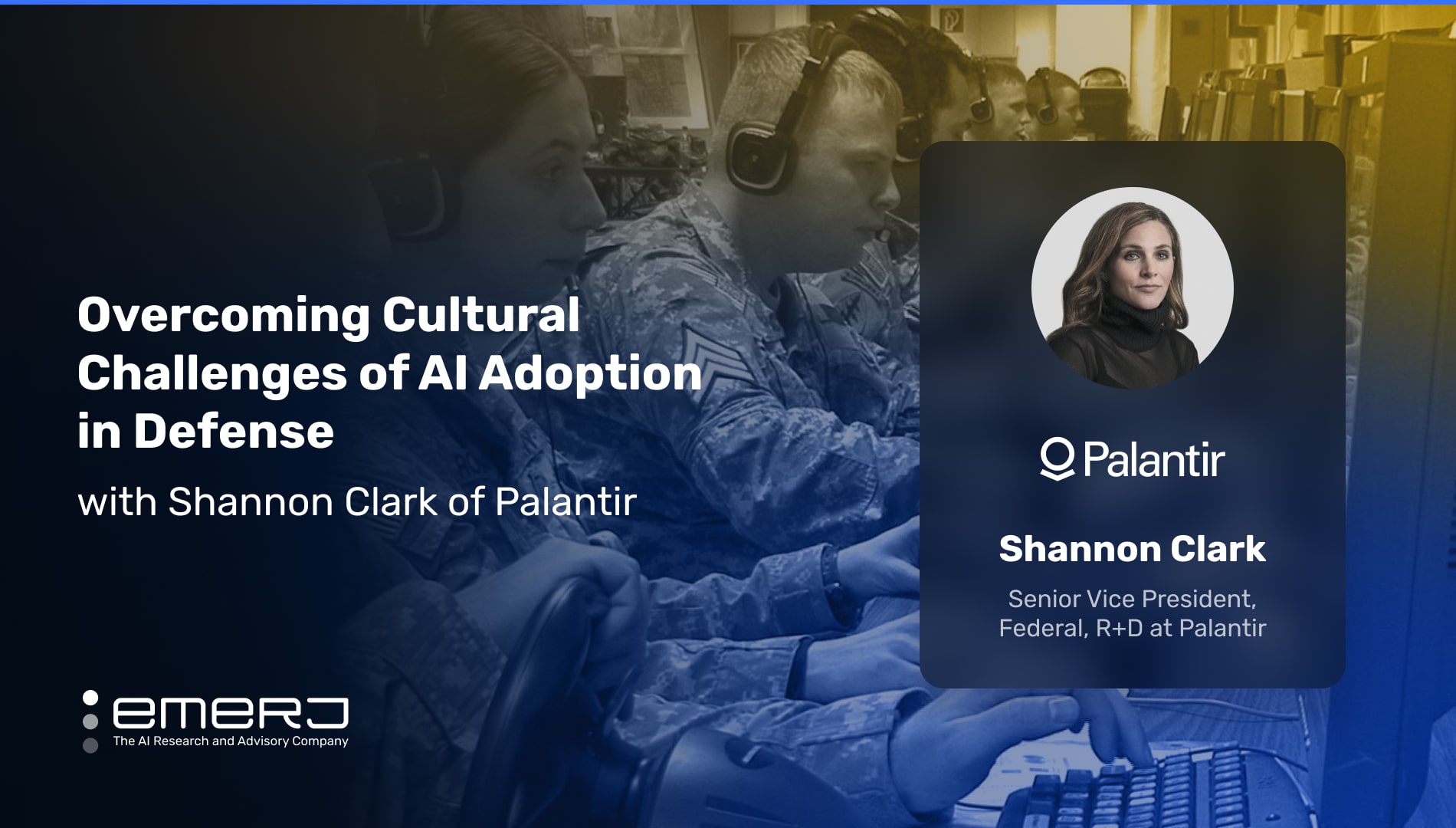
AI adoption poses several challenges for organizations, including the need for specialized skills and expertise, integration with legacy systems, data quality and accessibility, and the ethical implications of AI.
Additionally, institutional resistance to change, employee fear of job loss, and concerns about AI’s ethical implications are among many factors that can hinder adoption. Overcoming these challenges requires a strategic approach that involves cultural and organizational changes, proper training, and the development of best practices for AI adoption.
According to academic studies in public sector productivity, implementing any technological change in the public sector costs more money and time, and the resistance to change is much higher than in the private sector. It stands to reason that governments and private sector operations, by nature, are resistant to change themselves.
By proxy, the defense sector provides fertile ground for developing best practices in AI adoption, particularly for large, legacy institutions with tremendous trouble in affecting organizational change quickly.
As RAIN AI CEO Gordon Wilson tells Emerj, often it’s these exact organizations that have the resources to deploy AI effectively in the first place, “It is really only companies with massive computing budgets that can train their own models and deploy them,” he tells Emerj of LLMs. “Right now, we have a massive subsidization of those applications that people are running on at places like APIs like OpenAI. But the bottom line is that adoption costs are too high.”
Yet when so many critical data storage functions for the very operation of society depend on the public sector, they must stay up to date with modern technology.
Emerj CEO and Head of Research Daniel Faggella recently spoke with Shannon Clark, Senior Vice President, Federal, R&D at Palantir Technologies, to discuss the realities and challenges of updating legacy systems in the public sector. In the following analysis of their conversation, we examine two key insights on overcoming two main obstacles to AI adoption.
- Overcoming emotional challenges: Demonstrating quick wins and showing how data can expedite work encourages subject matter experts to suggest efficiencies for faster AI adoption.
- Overcoming cultural challenges: Public sector entities need more private sector product and software engineering talent to understand the fundamental nature of specific data challenges.
Listen to the full episode below:
Guest: Shannon Clark, Senior Vice President, Federal, R&D at Palantir
Expertise: Enterprise leadership, public-private sector collaborations, counter-terrorism, national security, defense systems, weapons technology, artificial intelligence and corporate governance.
Brief Recognition: Shannon Clark leads innovation, strategy, and growth with Palantir’s Defense clients. During her decade-long tenure at Palantir, Ms. Clark has led multiple facets of the business and product, including Palantir’s Edge AI work. Before joining Palantir, Shannon was a Director for Counter-terrorism Policy on the National Security Staff at the White House. She planned, directed, and coordinated the development of counter-terrorism strategies for the NSS.
Overcoming Emotional Challenges
Shannon begins by relating the work of making legacy systems AI-ready to performing an autopsy on someone’s data to find out what is underneath the ‘skin.’ She further compares the process to ‘peeling the onion’ layer by layer to see what’s wrong with a few datasets and what are the essential datasets to take further.
Many employees – in both the public and private sectors, but especially private – have that attachment to their data. They are understandably worried that the system will disappear if they give up their data, and their job will become irrelevant. Yet as Ms. Clark mentions, getting access to the correct data is more of an emotional challenge than a technical one.
According to a study from the UK Joint University Council and Public Administration committee, there is an individual challenge in addition to the system and organizational level challenges to data and AI adoption. The study’s authors noticed that high-risk-averse individuals are less likely to adopt and utilize big data.
To address this challenge in the field, Shannon recommends showing stakeholders a quick win, like how valuable their data can be and how the datasets can make the process faster, quicker, and more efficient.
Shannon shares a story about a teammate who flew to talk to an organization about an outdated legacy data system. The employee responsible for the system had been there for over two decades and knew everything about the code, down to which symbol would translate to what outcome. She emphasizes that they didn’t want to take away the person’s job but wanted to make the datasets more powerful.
As she sees it, the solution to the emotional challenge is to make people understand that AI aims to make the data valuable and improve their job rather than work against it.
In another defense-focused episode of the AI in Business podcast, Raytheon Head of AI Shane Zabel tells Emerj that one of the best ways vendors and AI stakeholders can demonstrate early wins is through a proof of concept (POC).
“You do learn a lot about a relationship with your vendor by actually going off and doing some POC,” Zabel explains. “That helps you explore what the business relationship looks like, the nontechnical aspects of doing artificial intelligence and machine learning, and how that’s going to look.”
Shannon continues to point out that, to harness AI’s power fully, the government needs to bring a distinctly educated AI workforce. The problem is that many small AI companies need help with the acquisition process. They find it challenging to convey the value of their product and figure out how to price and acquire it. It is especially difficult for startups trying to become part of large programs.
Shannon believes that we need to level up the community and educate the current acquisition workforce about the value of AI. She compares the current situation to the early days of cybersecurity when people needed to understand the potential of the technology entirely, and the opportunity to effectively regulate the technology slipped through the fingers of lawmakers.
She encourages young folks entering the acquisition community to learn about AI and help bridge the gap between generations.
Overcoming Cultural Challenges
Ms. Clark shared an experience when she worked on a project with the Department of Defense to produce computer vision algorithms and put them on legacy systems. While it did not work from a user standpoint, the systems also were not ready to adapt to AI. After a careful investigation, the DoD learned they had to start from the search because the legacy systems were not AI-ready.
Further, the speaker shares her perspective on how the government can hire and retain talented engineers to work on complex problems. She disagrees with the notion that the government needs to hire more engineers, as she believes that many gifted engineers are not interested in working under the bureaucracy and timelines of government projects.
Instead, she suggests that the government outsource engineering work to product and software companies with the best talent.
As someone who spent a part of her career in the intelligence community, Shannon tells Emerj she understands the challenges of working in bureaucratic environments and thinks it is essential to provide engineers with more freedom to work on their projects how they want. She believes outsourcing engineering work can help reduce bureaucracy and give talented engineers more opportunities to work on challenging problems.
While she appreciates the Air Force’s initiative to teach people how to code, she does not think the government should force DoD to hire more engineers. Instead, she suggests it is more efficient and cost-effective to partner with companies with talented engineers on staff and devote them to working on some of the government’s complex problems.
By doing so, the government can tap into the expertise of the private sector while reducing bureaucracy and providing talented engineers with more freedom to work on their projects.
She pushes back on the idea that some individuals or organizations may need to be smarter to acquire or understand the importance of a talented engineer in-house.
Instead, Shannon emphasizes the need for leaders to understand what it means to bring AI in-house and clearly understand the rubric for what makes an effective engineer. She further highlights the importance of showing up with a working product rather than just a PowerPoint presentation when demonstrating capability.
Ms. Clark recommends selecting a few different AI groups and requiring them to show how their products work with real-life data. She hopes the government will continue to lean into this approach and give smaller AI companies a chance to demonstrate their capabilities rather than relying on the same old vendors.
Lastly, she emphasizes allowing room for failure and experimentation in engineering projects and following the concept of ‘fail-fast.’ While nobody politic of taxpayers has much of a stomach for paying for failed projects, She believes failure is essential to learning and that engineers should be encouraged to think creatively and push the envelope.
Citing an example using expensive Palantir satellites, Shannon emphasizes many prototypes and experimental initiatives among important defense priorities may not work the first time – but it’s the only way to know if it will work the second or third time.
Throughout her appearance on the podcast, she reiterates her passion for building products beyond the basic requirements and her desire to see companies be more creative in their approach to engineering. She emphasizes that a large contract should leave room for exploration and experimentation and not just stick to a list of a hundred things.