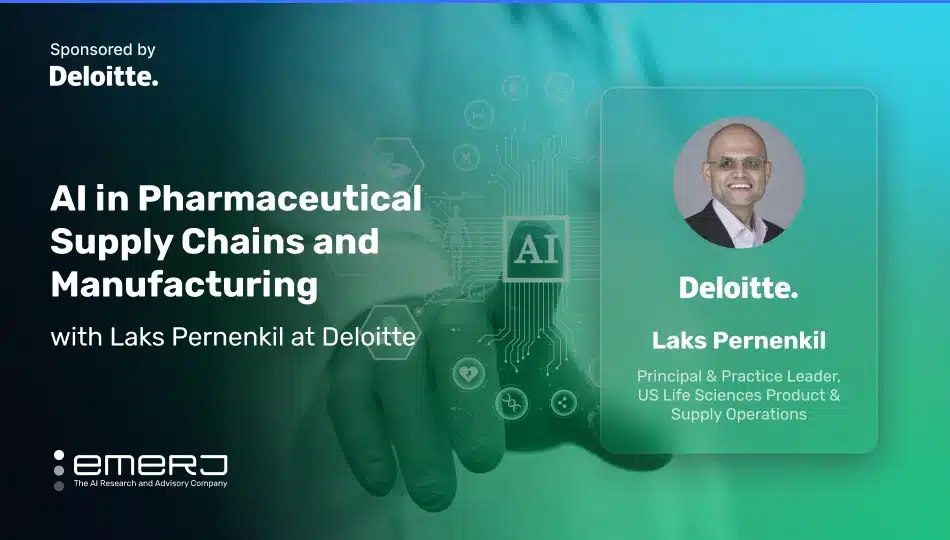
This interview analysis is sponsored by Deloitte and was written, edited, and published in alignment with our Emerj sponsored content guidelines. Learn more about our thought leadership and content creation services on our Emerj Media Services page.
Safe and effective drug development requires the successful implementation of clinical trials. In recent years, the increase of data-driven approaches to personalizing patients’ healthcare is, in-turn, driving demand for medicines developed with cutting-edge machine learning and AI-enhanced techniques, which is why a lot of the AI focus in supply chain manufacturing centers around clinical trials and drug development.
Along with the benefits of AI-enhanced clinical trials and drug development processes, emerging AI use cases in life sciences extend beyond far beyond these workflows, including demand forecasting, quality control, and supply chain optimization. Now pharmaceutical leaders are coming to understand the new status quo depends on their ability to maintain a competitive advantage in improve supply chain performance.
Emerj CEO and Head of Research Daniel Fagella recently sat down with Laks Pernenkil, a Principal at Deloitte specializing in life sciences, to talk about challenges in manufacturing for pharmaceutical supply chains where data and new AI capabilities are driving real value for business leaders.
The following article provides life sciences and healthcare leaders with three principal actionable takeaways from their discussion:
- Leveraging industry trends with document processing: Leveraging paper-based data with machine learning to Identify three current trends that enable pharmaceutical companies to use AI for backward-looking analytics and forward-looking decision-making.
- Data governance for report generation: Criteria for assessing the readiness and completeness of data necessary to leverage generative AI use cases in manufacturing report generation.
- Understanding AI to guide investments in the technology: Helping industry leaders conceptualize the benefits of AI in order to support their decision-making regarding investments in AI initiatives.
Listen to the full episode below:
Guest: Laks Pernenkil, Principal, Life Sciences at Deloitte
Expertise: Performance improvement, operational excellence, market entry strategy
Brief recognition: Laks has served with Deloitte since 2008. Prior to Deloitte, Laks lead delivery temas in large-scale, complex manufacturing and supply chain operating model transformations. He has an MBA from MIT Sloane School of Business and a dual B Tech & M Tech degree along with a bachelors in Chemical Engineering from the Indian Institute of Technology, Madras.
Leveraging Industry Trends with Document Processing
Pernenkil begins his podcast appearance by explaining how — during his two decades in technical operations, manufacturing, supply chain quality, and multiple areas of pharmaceuticals and medtech — he has never before encountered the current heightened level of surprise clients have today regarding the pace at which AI and data have taken over the entire focus of organizations. Every single one of his clients is actively considering what AI means for their business, specifically in operations.
He emphasizes how data has always been relevant. Operations departments have always needed data, particularly when making decisions pertaining to supply chains, manufacturing, or quality, because they’re highly engineered products and processes.
Pernenkil then describes three current trends in the life sciences supply chain space:
- Unused data is getting more attention than ever
- Availability of new ‘industry borrowed’ technologies to capture data
- Regulators themselves leveraging AI
Regarding the industry spotlight on unused data that Laks observes among his clients, he makes a brief anecdote: “My thesis advisor for my Ph.D. would often say the best way to reduce any variance or any error in a process is not to measure it. In the industry, it’s always been the norm that, in the past and a long time before, you don’t measure if you can’t explain why the data is the way that it is,” he says candidly.
He then continues, noting that a typical manufacturing floor creates a terabyte of data every day, a meager 5% of which is used in operations. Subsequently, pharmaceutical operations across the market and “our clients are out to change that,” Laks articulates, placing exacting detail on the scope of the problem.
He then provides a brief but thorough overview of what he calls typical L0L1 systems and manufacturing architecture, explaining that:
- Manufacturing architecture is divided into six levels, 0-5.
- Level 0 includes sensors on the production floor, and level 5 consists of enterprise planning systems.
- 70-80% of data is generated at the L0 and L1 levels
- The remaining 20% is split between metadata associated with all other systems that control the manufacturing process and the resulting business systems.
Laks explains how initially bringing machine learning and AI onto the production floor covered use cases and applications focused on trying to get control of the manufacturing process, inventory, distribution, and logistics of your products in the marketplace.
He explains a specific use case in which 80-90% of his clients are trying to actively deploy products in the marketplace, where a lot of deviations are required to be reported by regulations that don’t have an impact on the products themselves. Someone has to adjudicate whether or not each deviation is a risk to the product or patient. Laks notes that factory employees can scan these deviations and surface the top 5% of significantly critical deviations for a human to look at more closely. He likens the example as being a ‘legacy’ use case.
The deviations reporting example also mirrors a use case discussed around the 19-minute mark of the program, where Laks is asked to describe an emerging use case where AI is used to measure and manage supplier performance and explain how the use case helps manage expectations in the supply chain. Laks notes that a typical pharma company uses 470 data points to evaluate how a supplier is doing, 80-90% of which consists of manually sifting through documents.
Summarizing previous points on the subject of document processing, Laks emphasizes to the podcast audience that using large language models to process data from certificates of analysis, invoices, and other supplier communications in order to determine how the supplier is performing is going to be a crucial enterprise capability for pharmaceutical companies going into the future.
Data Governance for Report Generation
After discussing the deviations in reporting use cases, Laks then turns to how his clients are now increasingly considering assistive, generative AI (GenAI) applications, in many cases, to submit annual reports. Typical companies have millions of documents, including standard operating procedures and manuals. Laks explains that a large language model can serve as an assistant for reading all those documents and generating content on its substance.
He insists that these report processing and generative capacities of GenAI are also the reasons that supplier management is another area primed for GenAI adoption, which would allow executives to see in real-time how suppliers are performing and identify trends to increase efficiencies. Companies can use that data to assist suppliers in adjusting their performance.
When asked what it might look like to train a system that identifies critical deviations, Laks notes that there are two parts to answering the question:
- The readiness of the data
- The completeness of the data to train and use in AI systems
In explaining the enormous lift of ensuring data readiness, Laks says that, while his clients have invested in systems and data, they might have yet to devote resources to cleaning and transferring the data into a usable format. As a general trend, he thinks clients only bother cleaning datasets that are required. Thus, Laks and his team used several unique ways to clean their clients’ data that required little human intervention, including graph methodologies.
Turning to the completeness of the data, Pernenkil specifies that the question here then becomes, “Do you have enough variation in your training data for the AI models to detect?”
Because first-generation AI and newer GenAI capabilities blend deterministic and probabilistic technologies, Laks describes training models as both an art and a science, a significant reason why these systems will need humans in the loop for the foreseeable future. Laks also notes that human feedback will be critical every time a manufacturing process in operations or supply chain changes in order to ensure that AI models will one day be able to help leaders better predict those changes.
He describes how he and his team have used new anomaly and trend detection algorithms to build training datasets and train AI models to recognize their lack of context, which will require more training for those models.
When asked to expand on what industry leaders might need to understand in order to make firm investments in AI, Pernenkil explains that AI creates more value the more it is used by colleagues on the front lines, including those in manufacturing, supply, and logistics. “A lot of the time, AI helps in correcting for what might be wrong steps that a particular operator might be taking, or an adjustment, or immediate turn to what’s called a ‘golden path’ for operations, or trying to get back to that golden path,” Laks tells the Emerj podcast audience.
Understanding AI to Guide AI Investments
Continuing on the subject of client-related use cases, Laks explains in depth how and why his team always asks their clients to think of value as a starting point – especially to resist the short-term fear of missing out that comes with all tech cycles.
He advises that if there is no value to be had from the proposed use cases, clients should only pursue that AI implementation if they are popular. He summarizes, “So starting with that value and then driving down to the use cases is another way to ensure that you’re delivering value back to the patient.”
He follows up firmly reminding the audience that value is only measured in a handful of ways, many of which seem less objective the more AI adoption initiatives mature, concluding that “[value] needs to tie back to something specific that the operations leadership team cares about as part of their objectives and goals.”
When asked about what advice he has for leaders who want to make AI a really high ROI endeavor but aren’t sure where AI would fit into their businesses, he offers additional insight. He explains that data mining for information on complaints or non-conformance to reduce the human invention required is a traditional starting point for pharmaceutical firms including many of his own clients.
Other possible starting points include inventory (on the supply chain side) and extracting more value from the manufacturing assets. It’s beneficial for Deloitte’s clients to deploy AI to manage their networks, including their manufacturing and outbound distribution networks, since they’re selling such costly products.