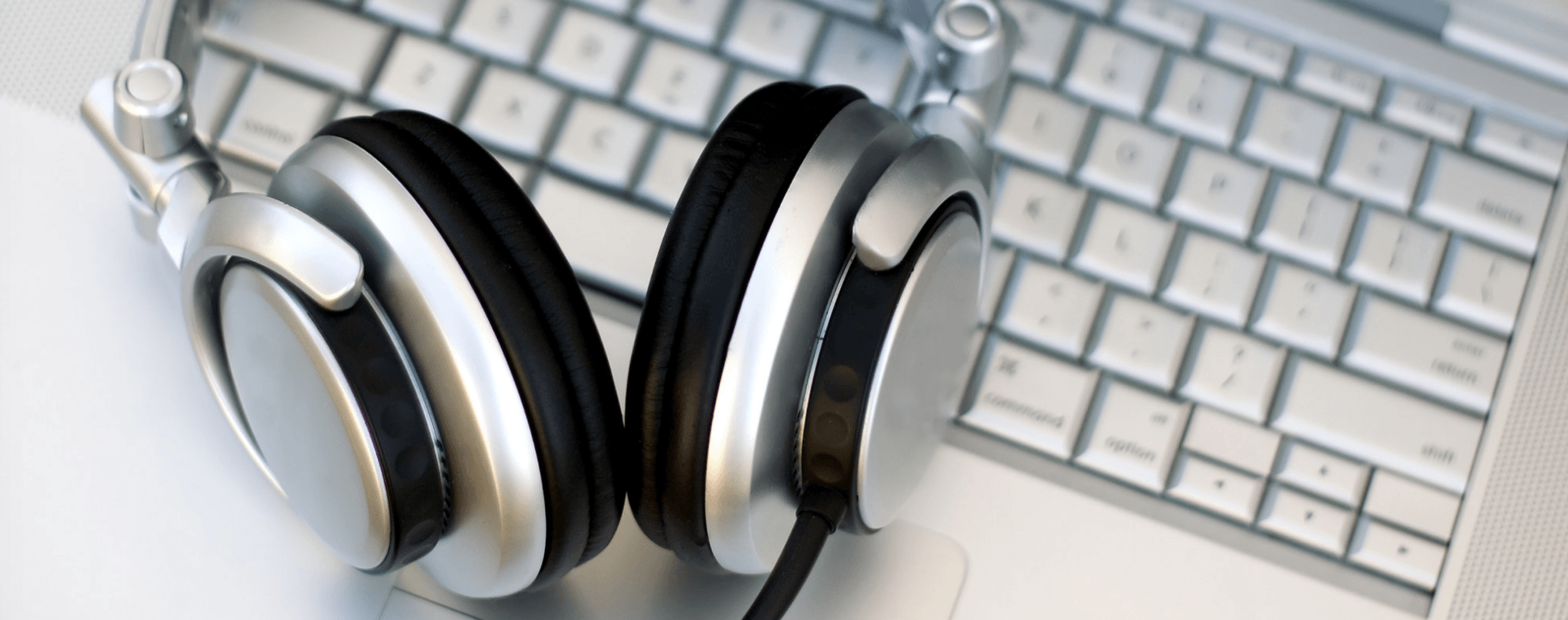
Artificial intelligence — especially machine learning — is at it’s best when it is working with a large, analyzable data set, like text. But much of the data in the world isn’t in text form. Instead, it’s in the form of spoken words on video and audio recordings or even live events. This makes reliable voice transcription an important goal for artificial intelligence.
In addition, voice to text transcription has long been an important business on its own merits in the medical, legal, and media fields, to name a few, and has traditionally been done by teams of human transcribers who charge rates of $3 or $4 per minute. According to the Bureau of Labor Statistics, there were 57,400 medical scribes and 19,600 court reporters (which includes closed captioners for television and other media) in the United States in 2016. Although it’s hard to find statistics for transcription specifically, Grand View Research projects that the global voice recognition market overall will hit $127.58 billion by 2024.
While it might seem like a straightforward project for AIs, since it can be seen as simply converting one kind of data (sound) into another (text), in fact, various factors make voice transcription a significant computing problem. Accuracy is especially important when transcriptions are affecting the accuracy of quotes in news stories, the outcomes of expensive and important trials, or even the lives of patients
Most people who use Siri or Alexa would agree that, while those tools do an admirable job of understanding a user most of the time, most of us wouldn’t trust them with our lives.
Even when an AI system learns to have high accuracy in the best case — for instance, a single clear-voiced speaker in a quiet room — maintaining that accuracy for multiple voices, multiple languages, heavy accents, background noises, crowded rooms, and more becomes very complicated.
And while voice recognition for vocal commands and voice recognition for transcription seem like similar problems, the latter is actually much more challenging. A voice assistant like Alexa only needs to determine which, if any, of a predetermined list of vocal command is being uttered, whereas a transcription program needs to listen for, and capture, any utterance at all. This wider variety of possible inputs and outputs makes it a harder task for AI.
Because of these challenges, AI experts lack consensus on how quickly, and even whether, computers will completely replace human transcribers. Gerald Friedland, the director of the Audio and Multimedia lab at the International Computer Science Institute at UC Berkeley, told WIRED Magazine in 2016 that “depending who you ask, speech recognition is either solved or impossible. The truth is somewhere in between.”
This research will seek to answer the following questions:
- What major tech companies are in the voice transcription space and how sophisticated are their offerings?
- What do the startup players in the market look like and how are they differentiating themselves?
- How do the needs and capabilities of existing AIs for transcription address different target markets like medical, legal, and media?
The Current Market for AI Transcription
Right now, the market for AI-based transcription is split between large incumbents and startups, who are each approaching the market differently. With some exceptions, larger players tend to offer speech-to-text as an API, as part of a larger product, or as an enterprise-level offering. Startups, on the other hand, are exploring business models to sell transcription software as a service directly to consumers and small businesses.
Major technology companies like Microsoft, Google, Baidu, and Nuance are all publicly involved in the space, but that involvement runs the gamut from research projects to fully realized commercial products, and many of the commercial products that do exist seem to focus more on dictation — transcribing one voice, on which the computer can be trained ahead of time — than transcription more broadly.
Some companies, acknowledging the limited abilities of computers today, offer hybrid human and AI transcription services, or tools that let the user manually “polish” the transcription if it’s taken from a recording.
While a few companies focus specifically on one subset of users (Saykara focuses on medical transcription, for example), most are casting a wide net and either offering general-purpose software or several different products for different market segments (Nuance, which offers specialty software for medical and legal transcription, is a good example).
The Big Names: Microsoft, Google, Amazon, Baidu, Nuance, Apple, Cisco Systems
Companies like Microsoft, Google, and Amazon have been researching voice recognition since the 90s, and that research has only accelerated with the emergence of virtual assistants like Alexa, Cortana, and Google Voice.
Microsoft Artificial Intelligence and Research made headlines a few years ago when they published a paper showing they had achieved parity with human transcribers in terms of transcription accuracy. They created a computer with a 5.9 percent error rate, the same rate as professional transcribers.
Since then, the company has continued to develop its technology and has integrated it into Cortana and the Xbox, but also has released a product called Dictate that lets users type by speaking in Outlook, Word, or Powerpoint. Google similarly added Voice Typing to Google Docs in 2015, as well as to many Android phones. Both Dictate and Voice Typing work well for dictating notes, but not so well for, for instance, transcribing a conversation in a crowded room, or turning a recording into a text document without listening to it in real time. They are more consumer tools than enterprise offerings.
Amazon offers a service called Amazon Transcribe as an API through Amazon Web Services. It can transcribe English or Spanish, timestamps words for easier checking, and works well for phone audio, according to the company, which also says support for multiple speakers is coming soon. The service is priced at $0.0004 per second, orders of magnitude cheaper than traditional human transcription services that can cost 2 to 5 cents per second.
Google also offers a speech-to-text API, at a cost of $0.006 for 15 seconds. Google claims on its website that it can recognize 120 languages, including proper nouns, and has different modes for phone audio, voice commands, and video audio.
Outside the United States, Chinese tech giant Baidu has been a major leader in artificial intelligence for voice transcription on the research side, implementing deep learning neural networks to create three versions of its “Deep Speech” project.
“Today’s world-class speech recognition systems can only function with user data from third party providers or by recruiting graduates from the world’s top speech and language technology programs,” the company wrote in a blog post last fall. “At Baidu Research, we have been working on developing a speech recognition system that can be built, debugged, and improved by a team with little to no experience in speech recognition technology (but with a solid understanding of machine learning). We believe a highly simplified speech recognition pipeline should democratize speech recognition research, just like convolutional neural networks revolutionized computer vision.”
On the commercial side, Baidu offers SwiftScribe, a web-based transcription software that is free, but requires a sign-up. It’s currently in beta and can only transcribe from a recording, not in real time.
Burlington, Massachusetts-based Nuance Communications is considered one of the biggest names in transcription software, and with good reason. The company was founded in 1994 as a spin-off of SRI International’s Speech Technology and Research (STAR) Laboratory to commercialize early speech recognition technology that the laboratory initially developed for the US government.
Today, Nuance’s AI-driven transcription technology is offered to businesses and consumers through its Dragon line of products, which are specialized for different industries and accordingly sit at very different price points.
The company’s general purpose dictation software is called NaturallySpeaking and comes in home and premium editions at $99 and $199, respectively. Dragon Anywhere is a mobile dictation software available as a subscription for $150 per year. All three are designed for dictation and computer voice control, rather than for transcribing interviews or recordings.
Users can transcribe from audio files with Dragon Professional ($300) and Dragon Legal and Medical ($500 each). The latter two are also available in cloud-based versions for organizations, which are priced differently for the enterprise. Dragon Legal and Medical have specialized vocabulary for their respective professions, and Dragon Medical also integrates with hospitals’ electronic health records. Nuance also offers business-specific tools for law enforcement, education, financial services, and more.
Until 2014, Nuance’s technology powered Siri, Apple’s voice-activated mobile assistant. Since mid-2014, though, Apple has had an in-house voice recognition team working on not only Siri, but on built-in dictation tools on iOS devices, as well as a feature that automatically transcribes users’ voicemails. Apple’s tools are definitely geared toward dictating short notes– the built-in dictation tools only work for about 30 seconds at a time.
Another company that provides transcription technology as part of a larger offering is Cisco Systems, which bought Tropo, a startup providing an API for voice and SMS applications to small businesses, in 2015. Through Tropo, Cisco can help customers embed text-to-speech and transcription (in 32 languages) to its customers.
Startup Challengers
Despite the significant research dollars behind voice transcription at the major tech companies, and the integration of the technology at various levels into their core products, only Amazon, Google, and Nuance offer serious standalone commercial options. This leaves a lot of room in the market for new startup players, and there are many.
The following seven companies are not an exhaustive list, but they are some of the most high profile entrants and are included to give an idea of the sort of services startups are offering, how they’re pricing their services, and how they’re differentiating themselves in the market. Data is sourced from Crunchbase, company websites, and occasionally media reports.
Trint
Location: London, England, UK
Current team size: 31
Founded: 2014
Funding: $4.9 million
Pricing: $15 per hour, with discounts for monthly subscriptions
The Pitch: Trint claims to be the first startup to offer consumer-facing pure-AI transcription, Trint’s web app is designed to transcribe long recordings with multiple speakers, which sets it apart from many of the free offerings from large tech companies, and its pricing is significantly cheaper than human transcribers (about 0.4 cents per second, compared to 2 to 5 cents per second for human transcribers). Beyond that, the app, built by frustrated journalists, is designed to make it as easy as possible for the user to make up for the AI’s deficiencies. The transcription is displayed in a browser such that the user can click on a word and be linked directly to the timestamp of the recording, to correct any errors after the fact.
AI Talent: VP of Engineering Simon Turvey completed a PhD at the University of Hertfordshire, focusing on “Analyzing and Enhancing the Performance of Auto-Associative Neural Network Architectures Dates”
Website: https://trint.com/
Simon Says
Location: San Francisco, California, USA
Current team size: 6
Founded: 2016
Funding: unknown/none reported
Pricing: $10 per hour, with discounts for monthly and annual subscriptions
The Pitch: Similar to Trint, Simon Says is designed for the media industry and automatically transcribes audio and video files, while presenting an interface that makes it easy for the user to correct mistakes. Simon Says claims to support 64 languages and 91 dialects and to be able to identify and label multiple speakers. It also allows users to import a wide variety of audio and video file types and export to a wide variety of text formats.
AI Talent: We were unable to find anyone at Simon Says with an academic or work background in artificial intelligence.
Website: https://simonsays.ai/
Demo video: https://www.youtube.com/watch?time_continue=12&v=H147Lhg9iSU
AISense (Otter)
Location: Los Altos, California, USA
Current team size: 17
Founded: 2016
Funding: $13 million
Pricing: Free (limited time)
The Pitch: AISense’s Otter app is designed for teams and workers to record (and, crucially, automatically transcribe) all their meetings in order to create a record of verbal interactions that might otherwise be lost or forgotten. According to the company, the tool learns the user’s voice to increase its accuracy over time, and interfaces with contacts and calendars to remind them to use it. It also automatically pulls out what it thinks are keywords in the conversation and uses them to create a tagging system.
AI Talent: CEO Sam Liang previously worked on AI at Alibaba and Google. VP of Engineering Yun Fu worked at Alibaba and Yahoo. Head of Product Simon Lau’s background includes stints at Oracle and Nuance. Other developers include Microsoft and Cisco vets.
Website: http://aisense.com/
Demo video:
Sonix
Location: San Francisco, California, USA
Current team size: 3
Founded: 2017
Funding: unknown/none reported
Pricing: $15 per month or $120 per year with additional $5 per hour for transcription
The Pitch: Sonix offers fast transcription; it claims to render an hour of audio into text in six minutes. But the tool is designed more for radio broadcasters and podcasters, who want to edit audio files visually. With Sonix’s text/audio editor, users can delete a word or phrase in the transcript and automatically clip it out of the audio, or highlight a word or phrase and save the timestamps to find them later. Transcriptions with a lower confidence level are color-coded for easier editing. Sonix also integrates with Final Cut and Adobe.
AI Talent: We were unable to find anyone on the Sonix team with robust AI experience, either in academia or at marquee companies.
Website: https://sonix.ai/
Demo video:
(More tutorials are available on Sonix’s YouTube channel)
TranscribeMe (Hybrid)
Location: San Francisco, California, USA
Current team size: 381
Founded: 2011
Funding: $6.6M
Pricing: 10 cents per minute ($6 per hour) for AI only, between $47.40 and $120 per hour for AI and human
The Pitch: Not all startups using AI for voice transcription are replacing human transcribers completely. Some are offering hybrid services, at an in-between price, that use machines to do a first pass and hire humans to edit and correct the transcriptions. This allows companies to offer the speed of AI but with close to 100 percent accuracy. TranscribeMe! offers variable pricing for different levels of automation and human contribution. It’s “Machine Express” service is AI only.
AI Talent: Cofounder and CTO Victor Obolonkin holds advanced computer science degrees from the University of Auckland, where he studied AI for audio analysis.
Website: https://transcribeme.com/
Verbit.ai (Hybrid)
Location: Palo Alto, California, USA
Current team size: 102
Founded: 2017
Funding: $34M
Pricing: Not listed on website
The Pitch: Another hybrid company, Verbit.AI uses transcribers who are hired and work over the internet. It boasts two layers of human transcribers to even further improve accuracy. The company offers clients transparency into the online transcription and even allows users to download a transcript midway through the process if it’s needed urgently.
AI Talent: CTO Eric Shellef completed a PhD in mathematics at the Weizmann Institute of Science, and worked on speech recognition for Intel Corporation before joining Verbit in 2016
Website: https://verbit.ai/
Demo video:
Saykara
Location: Seattle, Washington, USA
Current team size: 12
Founded: 2015
Funding: $2.5M
Pricing: TBD
The Pitch: Saykara, which only emerged from stealth mode last fall, described itself to CNBC as an “Amazon Alexa for healthcare”. It’s using AI transcription specifically in the medical field, where documentation requirements increasingly hamper doctors’ ability to connect with patients and many hospitals already employ full-time medical scribes. The team hopes a narrow focus will help them to solve the particular problem of medical transcription efficiently.
AI Talent: CEO Harjinder Sandhu co-founded MedRemote, a healthcare-focused speech recognition startup acquired by Nuance in 2005. Sandhu went on to serve with Nuance as Chief Technologist for Healthcare R&D for several years. Other members of the team have backgrounds at Amazon, Microsoft, Google.
Website: http://www.saykara.com/
Concluding Thoughts
Voice transcription is a hard problem, but artificial intelligence seems to have finally reached the point where its ready to tackle it. Though – for now – even the “fully automated” solutions in the market are still in a sense hybrid model; they simply rely on the user, rather than a hired transcriber, to correct the transcript.
As researchers and companies improve and refine their algorithms, it seems evident that transcriptions will become even more accurate, though it’s unclear if they’ll ever hit 100 percent or an effective 100 percent. As they get closer, new use cases, like automated captioning (already available on many YouTube videos), will become more feasible. Additionally, the benefits of using human transcribers will diminish, so it would make sense for the market to increasingly switch over to automated systems.
One consideration that’s going to be increasingly relevant is privacy and security. While switching to automated transcription creates more privacy in some ways (since a human is no longer listening to conversations and interviews) it also has the potential to create new privacy problems if companies like Otter, which encourages users to record all their meetings and conversations, aren’t careful and transparent about what they can and can’t do with that recorded data.
But whatever problems emerge, the potential productivity savings of automated transcription are hard to ignore, and it’s clear the technology is here to stay and getting better. Someday, we might be headed for a world where audio tape and text are thought of not as distinct media, but as two formats for the same content, as interchangeable — and as convertible — as an .mp3 and a .wav file or a text file and a Word document.
Original research and writing for this article was completed by the Emerj team, with final edits and adjustments by Daniel Faggella.
Header image credit: Adobe Stock