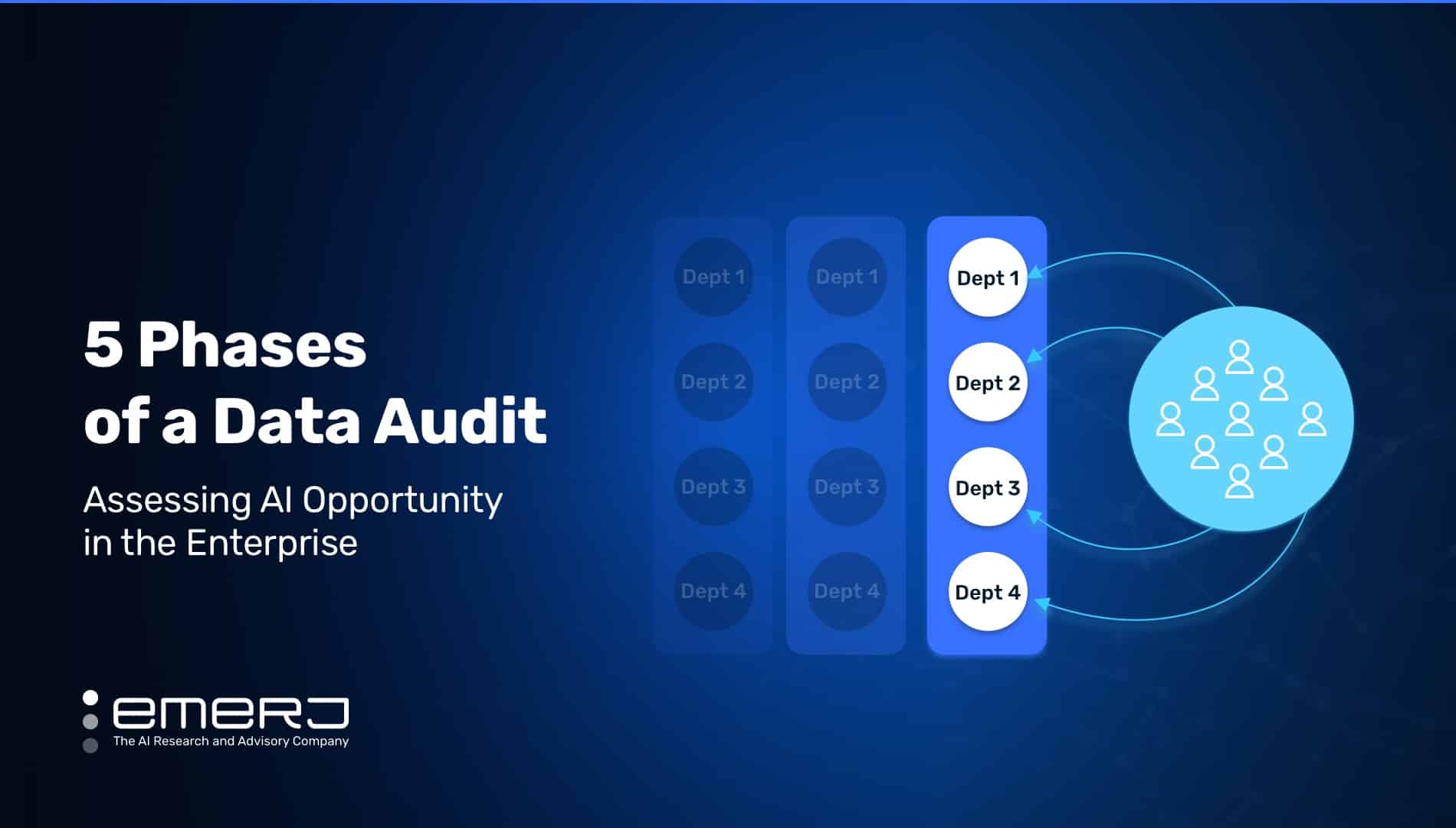
Emerj serves enterprises to form their AI strategies, and data audits are part of Emerj’s framework for identifying high-ROI AI projects. In this article, we’ll break down a slice of this framework, walking through some pragmatic steps leaders can take to drive toward industry-leading outcomes in their organization.
In the domain of artificial intelligence, it’s known that experienced data scientists are needed for architecting effective solutions, and it’s also recognized that business leaders and subject matter experts must have a clear vision to drive toward successful business outcomes for AI projects.
The overlap needed to empower effective strategic decisions about investments in AI is less clear. While executive leaders needn’t become data scientists, it’s critical that business leaders have a practical and conceptual – if not technical – understanding of the organization’s data and infrastructure as a gateway to understanding AI opportunities in their organization.
In previous Emerj Plus articles, I’ve covered the importance of executive AI fluency. While most data audit resources are technical, and geared towards IT leaders or data scientists – this article is designed to be an overview for executive leadership with no technical background.
Why Should Large Enterprises Conduct a Data Audit?
Reason 1: Avoid Wasted AI Spend
Emerj is often engaged for advisory after millions have already been wasted on poorly-chosen pilot projects. We’ve heard many tales of large investments made with incorrect assumptions about how ready teams were to deploy technology and how ready the technology was to deliver results. Gartner also predicts: “through 2022, 85 percent of AI projects will deliver erroneous outcomes due to bias in data, algorithms or the teams responsible for managing them.”
Let’s be clear; AI is a strategic technology, not a tactical one. And because AI requires a new set of skills, a new set of tools, a new way of operating, it’s important that leaders take this new way seriously and pick a direction they can commit to in the longer-term. Leaders should be preparing themselves not just to plug in AI on a small project, but to double down for a transformation.
To succeed with profitable AI deployment, leaders need:
- Robust understanding of the practical capabilities delivered by AI solutions under consideration.
- High-level alignment among leaders and stakeholders, with a vision for long-term transformation.
Securing these qualities among leadership ensures that pragmatic and supportive steps can be taken to mitigate risks or pivot course when inevitable challenges arise with the implementation of new technologies. And the successful execution of a thorough data audit is an especially strong way to nurture this necessary understanding and alignment among enterprise leaders.
Reason 2: Properly Assess AI Project Options
A data audit affords leaders a clearer view of:
- Which data streams might be most valuable for AI applications.
- Which AI applications might be worth trying and testing now.
With these areas of understanding – leaders can select AI projects intelligently, bearing in mind the inevitable deployment challenges and the (probably harsh) realities of their current data infrastructure and AI readiness.
When leadership (whoever is cutting the check) and project stakeholders (subject matter experts, data scientists, external AI vendors) are on the same page about realistic opportunities and AI readiness, the most blatant mistakes of AI project selection are likely to be overcome.
The 5 Phases of an AI Data Audit
From an executive perspective, an AI data audit can be broken down into the following phases or steps:
- Determine Objectives for the Data Audit
- Determine the Cross-Functional Data Audit Team
- Determine Where to Focus (departments, functions, processes)
- Explore Focus Areas
- Summarize Audit Findings
The graphic below is intended to be a visual representation of phases two through four. In the subsections below, we’ll explore each of the phases in greater depth.
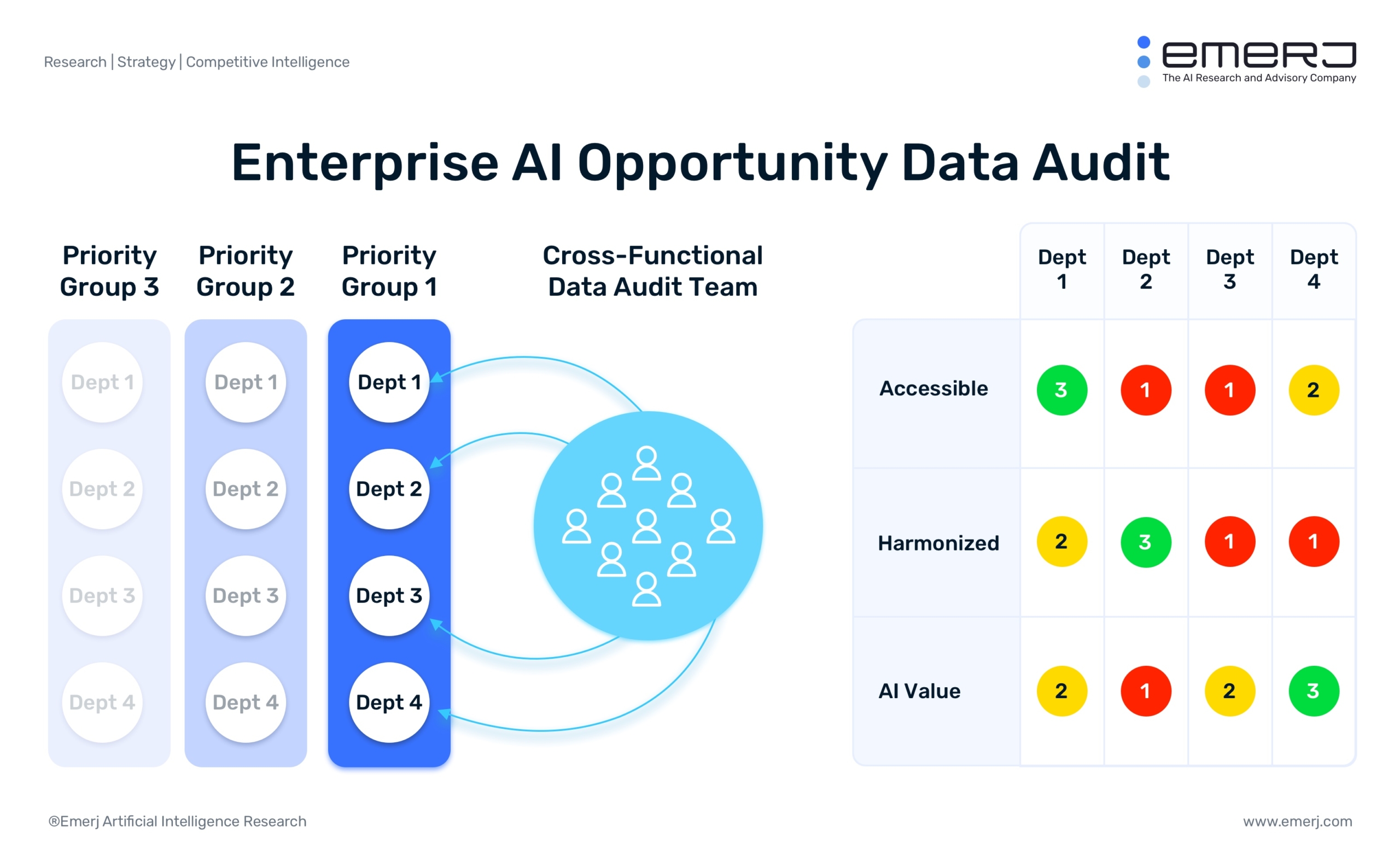
1. Determine Objectives for the Data Audit
Clearly defining the audit’s high-level objectives and deliverables upfront aligns leadership and focuses questions and investigations toward the highest-value opportunities.
A data audit will almost always aim to (a) gain an objective understanding of the company’s most important data assets, and (b) determine the potential AI value or improvement opportunities for each of those important data assets.
However, the full list of data audit objectives may vary greatly from company to company. Leaders may focus on particular departments, business processes, and investigate specific technical or security requirements. Ask yourself:
- What information do we want to gain from this data audit?
- What do we want to be able to do with the information we gather from this audit?
These questions – and their answers – should also be run by your cross-functional data audit team – which takes us to our next phase:
2. Form a Cross-Functional Data Audit Team
A well-formed team is composed of:
- At least one in-house data scientist. Total reliance on consultants or AI vendors is a surefire way to screw up a data audit. Even if vendors or consultants are involved, someone with the company’s best interests at heart must be involved through the entire data audit process. Executives drinking data insight through the biased filter of a vendor will almost never lead to objective insights about the state of the firm’s data, nor will it lead to the best next steps after the audit.
- A functional business leader. Someone with a strong understanding of the financial constraints of the company or department, as well as the goals of key thrusts of the company or department. This leader should have a firm conceptual understanding of AI capabilities and deployment contexts. This leader is often the champion of the AI data audit process, and is either the one writing the checks, or is the one negotiating with higher leadership to get the funding the project needs.
- Subject-matter experts (SMEs). SMEs from various departments will need to be involved in the data audit process. We’ve written more about the importance of SMEs, and their role in AI projects, in a previous Emerj Plus article titled: The Critical Role of Subject Matter Experts in Applied AI Projects.
This mix will vary from team to team, and the talent needs vary depending on what phase of the process we’re focused on (see our full report The AI Deployment Roadmap to learn more about the talent needs across an AI project lifecycle). Often, IT leadership will be needed to navigate and gain access to byzantine data stores or IT systems.
This team, along with the project sponsor, should come together to further distill and prioritize the audit’s scope, ensuring that the team and its stakeholders have a solid understanding of the high-level requirements and action items necessary for execution.
3. Determine Where to Focus
In this step, the audit scope is defined and locked in. While some audits might touch a dozen business functions, others may only dive into 3 or 4 carefully-selected areas. Even if a broad scope is warranted, some areas will deserve a deeper dive than others.
Three good ways to divide the scope for a data audit include:
- By department – i.e. Compliance, customer service, marketing, etc.
- By business process – i.e. Claims management, recruiting, etc.
- By data type – CRM data, payment fraud and refund data, website user activity data, etc.
Here are some good questions for leaders to consider during this process:
Which functions are likely to have the most important data that could have AI-related value for the business?
Which functions or processes are most important for the business overall, in terms of strategy and vision?
Executing a pragmatic prioritization process ensures that focus areas will receive more attention as initial projects are chosen and implemented.
4. Explore Focus Areas
Assess AI talent, and engage experts
- Talk to IT experts and data experts within those business functions, processes, and departments.
- Talk to subject matter experts.
- Talk to data scientists in those departments (if present)
Assess data quality
Assessment of the following strata of data quality on a 3-point scale (see the example graphic above) is a good place to start:
- Accessibility – Will the data need to be centralized? reformatted? Are there regulatory matters to consider? How frequently must the data be accessed?
- Harmonization – How harmonized and organized is the data?
- AI Value – Of what value is it to us?
The three factors above are almost always useful strata to score and rank data opportunities, but each company will have its own additional considerations.
Scoring each focus area provides an intuitive view of where the opportunities lie. The image at the top of this article shows an example.
Next, examine potential AI use cases and return on investment within each department, function, and process. Engage subject-matter-experts to map AI implementation opportunities to audit areas. Consider:
With this given data corpus, with this particular business process, within this business department, what are the precedents of use?
This requires a strong understanding of the AI opportunity landscape within your sector, and potentially also strong competitive intelligence to understand how competitors and adjacent industries are proceeding and how exactly AI integrates with their strategies.
5. Summarize Audit Findings
Audit findings are best-summarized in documentation which communicates the insights and opportunities identified, in clear language and graphics for stakeholders at all levels, including in-house data scientists, in-house subject matter experts, and executives.
This documentation should succinctly summarize the findings and frame insights in a pragmatic context about what it means for future AI deployments within the company.
- Where are the strengths and weaknesses of our current data ecosystem, in all of the component parts we analyzed?
- Where are the opportunities to apply AI and deliver value? Which AI applications might be used?
- Where are the opportunities to modernize or improve our data infrastructure to enable future capabilities for the business?
These findings can evolve over time, toward a more robust knowledge base, informing new projects as they evolve.
From Audit to Action
An Audit is for Learning
The importance of finding where we need improvement is more important than finding near-term ROI. Yes, we want to anchor all projects in measurable results, but there are so many hurdles that make near-term projects challenging.
Building critical capabilities to allow us to nimbly deploy these technologies is a serious competitive advantage – so it’s important that we build these skills and capabilities. As much as an initial financial ROI is important, but it’s important that we retain the learnings we need to retain to be stronger in the future.
Please read our full article about elements of AI maturity: Critical Capabilities – The Prerequisites to AI Deployment in Business.
After a proper AI audit we should be able to answer both of the following:
- Where could AI be used to strategic value in our company?
- Where do we need to improve our AI maturity most in order to enable AI capabiliteis in the long term?
Any complete focus on short-termism without factoring in the value of learning is completely missing the point of early AI deployments. While we often have to work under conditions where executives have a less-than-ideal understanding of AI’s challenges and requirements, it’s the responsibility of AI project leads to prepare executives adequately, and to set the right expectations upfront. Doing anything less will result in failed, fleeting AI pilot projects that never make it to deployment (see the Gartner stat from the beginning of this article).
Companies who complete a data audit should record the process they used and the lessons they learned so that the core data audit summary can be applied again – either to different aspects of the company’s data ecosystem or to the same elements of the data ecosystem at a later date (to determine changes or improvements over time).
Select AI Projects
When we have the results of our AI data audit, we can make better decisions about which AI projects to invest in, and ground those decisions not only int he possibilities of AI (which we discovered and worked through as part of this process), but also as part of the current reality of the state of our data infrastructure and talent.
Only with this kind grounding and shared understanding with our stakeholders and internal experts can we expect to invest in the right projects. AI investments made “in a vacuum” (without context on the current state of our data ecosystem, and without a strong understanding of the AI applications that might suit us best) will almost always fail, and won’t even result in retained learning, nevermind business value.
Some execs want to think that AI is IT. But if we’re looking for a sustained advantage, then we need a focus on:
- Current capabilities of AI
- Grounding in our current realities and state of data/talent
- A forward-looking AI/digital transformation vision
Selecting a first AI project is not plug-and-play, it is leaping into a new way of doing business, a new way of working with teams, a new way of handling and treating data. Companies who take it that seriously will pick companies more carefully, but they’ll be astronomically more likely to see both short-term and long-term ROI.
Emerj for AI Services Leaders and Consultants
AI consultants and AI services leaders are a critical force for change within the enterprise. Large organizations need outside experts to help determine AI projects, assess AI opportunities, and guide AI strategy.
If you’d like to determine the industries and companies most in need of your AI services, and if you’d like a proven method for conveying the value of AI projects (turning AI from a “nice to have” to a “need to have”), consider applying to our Catalyst Advisory Program.