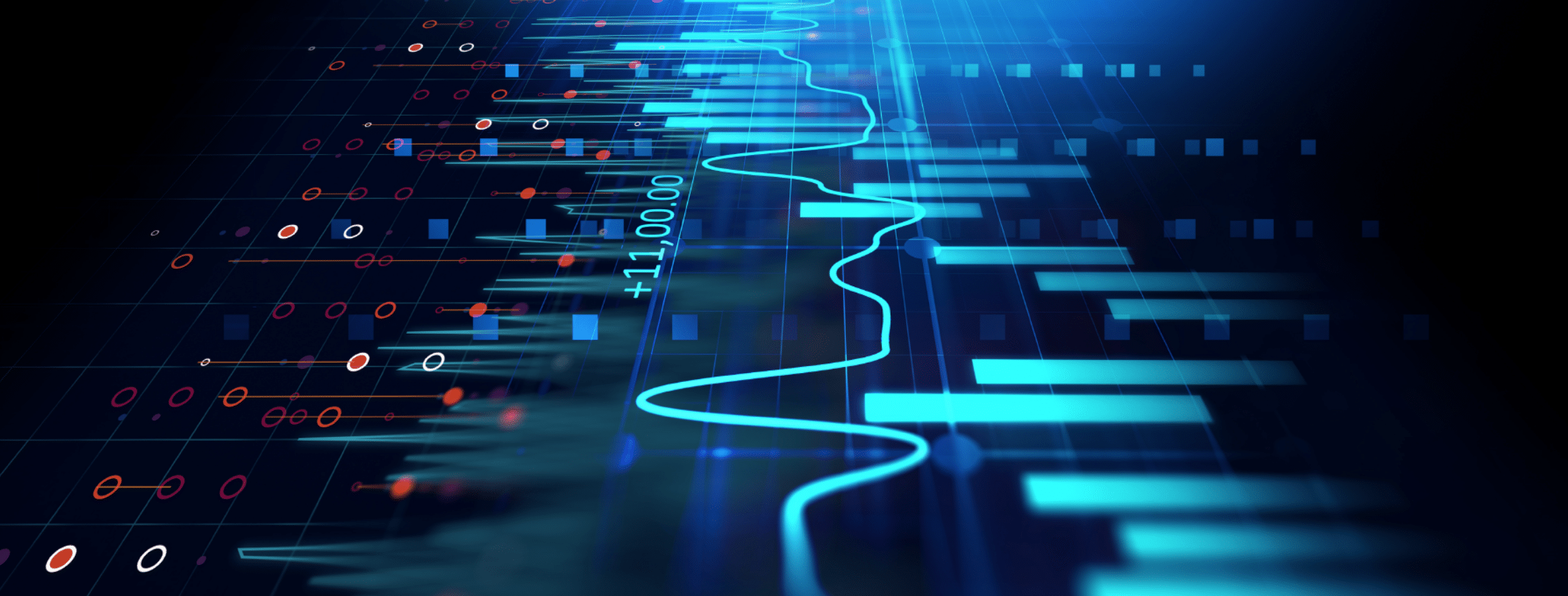
Business leaders, managers, and consultants with an eye on AI aren’t just trying to learn what AI can do, they’re trying to discover ways to gain an AI advantage.
For this reason, discovering AI trends can be particularly important.
- Non-technical professionals who want to contribute to – or even lead – their company’s AI initiatives need to have insight into their sector-specific AI trends.
- Leaders need to understand AI trends to compete in the marketplace, and determine the best strategic AI investments.
- Consultants need to come to their clients and prospects with fresh ideas and open opportunities, to “open the eyes” of their clients in ways that demonstrate competence and value.
Most of the work that we do with our AI Capability Map services is about finding trends in quantitative data – which requires hundreds of hours of expert research, and established frameworks for interpreting and categorizing data for insight.
While this robust research approach is often the right step for companies who are on their way to building a complete AI strategy – it’s easiest to begin with simple secondary research online – and that’s our focus in this article.
In the rest of this very brief guide, we’ll highlight some of the strategies we use in our research and editorial efforts at Emerj.
1 – Follow the Venture Money
While AI startups can raise $2MM or even $10MM with just a timely idea or an impressive resume, $100MM doesn’t come so easy.
Large B and C funding rounds often imply significant evidence of traction within the market. While some companies get lucky, the AI applications raise the most money in a sector are giving those kinds of AI applications the best likelihood of surviving.
Look across the firms who have raised the most money in a given sector (this information can often be found publicly on Crunchbase, Owler, or Pitchbook). Of the 20-80 legitimate AI startups within a given sector (e.g. auto insurance, drug development, trucking, etc) or function (e.g. email marketing, HR, etc), the top 10% of firms by “funds raised” is likely telling a story.
Patterns can begin to emerge when we ask the right questions about the top VC-backed firms:
- AI-enabled capabilities. What specific capability are these technologies enabling? What new ways of running processes or serving clients are these applications making possible?
- Data access and availability. What kind of data do these applications feed off of? What kind of data seems most valuable? What new kinds of integrations are making this data accessible?
- Claims of success. What are the success claims of these top vendors? Do any of them have concrete, quantifiable results to speak of, or do they only mention vague results – or simply list client companies with no success claims at all?
Examining the best-funded legitimate AI firms in a sector is by no means a complete view of AI innovation; it can be the start of “peeling back the onion” on trends in a sector that reading blogs or press coverage would never arrive at.
Related resource: How to Cut Through the Artificial Intelligence Hype – Three Simple “Rules of Thumb”. Sift the real AI companies out from the hype to improve your web research efforts. Hundreds of our readers swear by these simple rules to sift through AI hype.
Related service: Emerj AI Capability Map. Access a comprehensive visual map of AI ROI in your sector, with a complete assessment of enterprise and startup innovation.
2 – Listen to Leading Industry CEOs
Press releases are almost meaningless when it comes to genuine AI insight. In any large sector (eCommerce, retail banking, insurance, etc), the announcements of enterprise companies don’t serve to disclose actual AI investment or adoption, they merely serve to present a brand image.
At Emerj we refer to this as the Lens of Incentives:
- Enterprises tend to emphasize and exaggerate the AI applications that make them look innovative and hip (often customer-facing applications, like chatbots).
- Enterprises de-emphasize or minimize the mentions of their investments in AI applications that might raise suspicion, or put the company’s AI strategy at risk.
For this reason, an enterprise will promote a press release about their AI “chatbot” even before their pilot with the chatbot company begins (and even if the actual bot fails miserably). The same company will almost never divulge the AI company that it uses for cybersecurity (even if they invest millions per year with this vendor), as this might put the company’s data at risk from savvy hackers – or raise security concerns in the eyes of customers.
Instead of press releases, listen carefully to the statements of top management (CEO, COO, etc) at the leading firms in your industry. In banking, this might be JPMorgan or Goldman Sachs, in retail, this might be Walmart and Amazon. Look for speeches and (often even better) panel discussions about AI in their industry – or the future of their industry – and get a sense of the trends they care about most.
These presentations often skirt major issues and usually won’t divulge the crown jewels of a competitive strategy, but they will often present a candid insight into the priorities of leading executives. Find patterns by asking the right questions:
- Threats. Are there any common risks or threats that executives aim to address with AI, or that they think might be caused by AI?
- Future vision. What kind of AI-enabled future are these leaders painting for this industry? What key changes do they think are inevitable? What are the implications for competitive strategy?
Related resource: The AI in Industry Podcast. In addition to doing your own research on the AI perspective of leading executives, let us do the research for you by asking hard-hitting questions. From the Head of Core Machine Learning at Facebook to the former Head of AI at HSBC, the AI in Industry podcast shares the best ideas of leading AI thinkers ever week.
3 – Lock on to Existing Industry Priorities
While artificial intelligence is enabling new business models and capabilities – and while AI may be the new electricity – enterprise “AI transformation” is still many years away in most sectors. Most bankers are not “rethinking” banking in light of AI capabilities. Most brick-and-mortar retailers are not “rethinking” retail. They are focused on whatever they were focused on before AI came on the scene – they’re simply aiming to keep the business running.
This means that AI will often not be adopted where it has the most promise for transformation, but where it has the most alignment with existing priorities in that sector.
These industry priorities can take a number of forms:
Constant priorities of a specific sector –
Example: In the US pharmaceutical sector, blockbuster drugs are key to success and market share – and are the most defensible, profitable opportunity for growth. Consequently, it should be of no surprise that AI applications in drug development have an easier time gaining traction (or at least executive conversations) than applications in, say, sales (where comparatively little is invested in proprietary tech).
Priorities specific to an era –
Example: After 2008, the banking sector has been increasingly focused on compliance and regulation. Not surprisingly, AI initiatives for fraud and compliance are massive compared to other banking functions (marketing or accounting, for example).
While each sector varies, the following questions can provide fruitful initial clues as to where AI is likely to gain traction more easily in a given sector.
- What keeps CEOs in this sector up at night, regardless of AI?
- What do industry leaders already think will separate winners from losers in their industry, and what do they think they need to do about it?
Related resource: Emerj.com “Industry Navigation” page. The Industry Navigation page allows users to search for AI innovations and interviews specific to their sector(s) of focus. Article topics include: Enterprise AI initiatives, AI vendor landscapes, and more.
Header image credit: eCloudvalley