Medical devices sector, part of the $3 trillion healthcare industry in the United States, is an area of growth and innovation where researchers and manufacturers are seeking to integrate accuracy with automation. According to the most recent data reported by SelectUSA, (a program within the International Trade Administration at the U.S. Department of Commerce), the United States held 40 percent of the $140 billion global medical device market in 2015.
There are several emerging applications of AI in the medical devices sector and business leaders and professionals are looking for insights on the impact of this technology.
- Types of AI applications currently in development at industry-leading firms
- Common trends among innovation efforts, the effect of these on the future of healthcare
- Applications that appear to deliver the most value for healthcare professionals
Before presenting examples of applications, we’ll take a look at some common themes that emerged from our research in this sector.
New Trends in AI for Medical Devices
Based on our research, current emerging applications appear to fall into three main categories:
Management of chronic diseases – Companies are using machine learning to monitor patients using sensors and to automate the delivery of treatment using connected mobile apps (Example: Diabetes and automated insulin delivery).
Medical imaging – Companies are integrating AI-driven platforms in medical scanning devices to improve image clarity and clinical outcomes by reducing exposure to radiation (Example: GE Healthcare CT scans for liver and kidney lesions).
AI and Internet of Things (IoT) – Companies are integrating AI and IoT to better monitor patient adherence to treatment protocols and to improve clinical outcomes (Example: Philips Healthcare solution for continuous monitoring of patients in critical condition).
(We decided not to include robotic surgery applications in this article about medical devices. For readers with an interest in robotic surgery, please see our recent article titled: Machine Learning in Surgical Robotics – 4 Applications That Matter.)
Medtronic – Diabetes
Diabetes, which is a leading lifestyle disease, affects about 30.3 million Americans. It is caused by a sustained, high level of blood glucose (blood sugar) which is a primary source of energy from eating food. Normally, insulin which is a hormone made by the pancreas facilitates the transfer of glucose into the cells of the body to generate energy. If insulin is lacking or insufficiently processed in the body, blood glucose levels cannot be properly controlled.
Medtronic is among the medical device companies forging into the AI space, and aiming to help diabetics manage their condition more efficiently for a better quality of life. In September 2016, Medtronic announced that its Sugar.IQ app, a mobile personal assistant developed in collaboration with IBM Watson, would be tested by its first users.
The company outlines the following three main features of the Sugar.IQ app:
- Insights – As the app uncovers behaviors associated with glucose patterns, personalized messages will be delivered in real-time to help people with diabetes understand how specific actions and habits affect their glucose levels.
- Glycemic assist – People with diabetes can ask the app to follow specific food- or therapy-related actions and events. By following items, Sugar.IQ will help discover the impact these items have on their personal glucose levels.
- Food logging – The app will quickly and easily track food in a diary to deliver meal-related insights that illustrate how specific foods impact an individual’s glucose levels.
The company claims that the app continuously monitors blood glucose levels by analyzing data generated from Medtronic glucose sensors and insulin pumps. These devices would be attached to the user’s body. Models, or algorithms, initially built on data from 10,000 diabetics are being improved to predict when blood glucose levels will fall outside of healthy ranges. Machine learning would reportedly be used to carry out the predictive analytics.
In the following quote from an IBM case study on the collaboration, Huzefa Neemuchwala, Head of Data & Informatics Innovation in Diabetes Service and Solutions at Medtronic explains the goals and challenges associated with the effort:
“Traditionally, Medtronic has provided systems such as continuous glucose meters and insulin pumps, which are physical devices used predominantly by people on insulin therapy to monitor glucose levels and administer insulin directly to the body. If we can harness these devices and provide continuous feedback on individuals’ glucose levels, the potential exists to support millions of people in the daily management of their condition.
The growing popularity of wearable technology also means that all-important biometric data on diet, exercise, sleep and medication is now becoming easier to capture. The challenge is to gain actionable insight from this massive volume of data, and deliver it to users quickly enough for them to make appropriate decisions.”
In June 2017, Medtronic announced the U.S. launch of its MiniMed 670G system, an insulin pump approved by the Food and Drug Administration (FDA). This is the first technology of this kind approved by the FDA, the company said. The medical device maker claims that the system automates the delivery of a baseline level of insulin to stabilize blood glucose throughout the day and night.
Similar to the algorithms used in the Sugar.IQ app, the company claims the MiniMed 670G system is trained on algorithms to “self-adjust basal (baseline) insulin delivery every five minutes” based on how much insulin is needed at a given time.
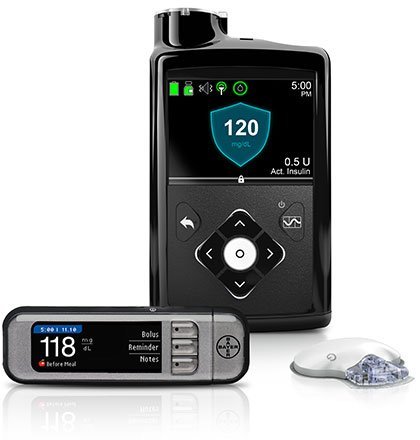
Johnson & Johnson’s exit from the estimated $1.7 billion insulin pump marker positions Medtronic to increase its market dominance to an estimated 74 percent (up from 61 percent) by 2019, according to a StarTribune report in November 2017. Johnson & Johnson previously controlled an estimated 11 percent of the market.
GE Healthcare – Medical Imaging
In November 2017, GE Healthcare and NVIDIA announced efforts to gain further traction in the medical device sector. GE Healthcare said it has 500,000 imaging devices on the global market. The two companies are reportedly involved in a decade-long partnership to integrate NVIDIA AI platform with GE Healthcare’s imaging devices.
The collaborators claim they are using AI to improve the speed and accuracy of computerized tomography (CT) scans. Algorithms are engineered to reorganize small patterns of organ damage that may be missed when a physician is simply viewing a scan. By capturing these finer details, there is potential for this technology to support faster diagnoses and reduced errors.
CT scans involve the combination of images from multiple X-rays of the same object through computer processing. Compared with a simple one-dimensional X-ray image, CT scans can generate “cross-sectional images, or slices, of the bones, blood vessels and soft tissues inside [the] body.”
Increased speeds mean less radiation exposure for patients, which may contribute to faster treatment times and better clinical outcomes. For example, the companies mention that the new CT system (part of GE Healthcare’s Revolution Family which is FDA approved) is “two times faster” than the previous system and is anticipated to more quickly identify liver and kidney lesions due to the high volume of data accessible through NVIDIA’s AI platform.
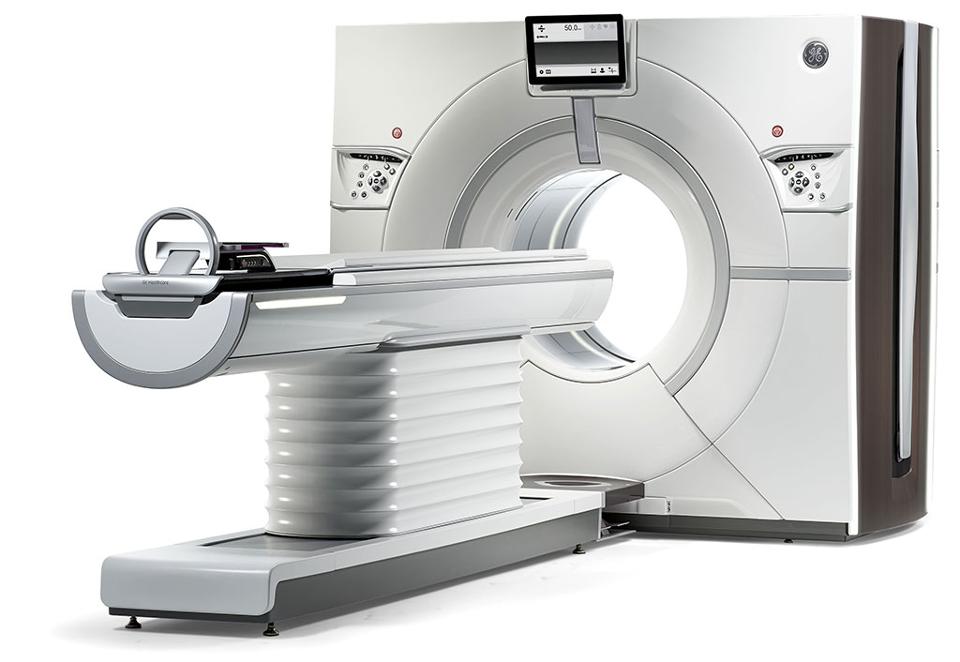
For a deeper dive into medical imaging, interested readers are encouraged to reference Deep Learning Applications in Medical Imaging.
Philips Healthcare – AI and IoT
Wearable technology is a rapidly growing area of interest for researchers and device manufacturers alike. Industry analysts project that the sector will reach $25 billion by 2019. However, there are important factors that must be considered when it comes to implementing wearables in a clinical setting.
A 2016 research article by the on the subject highlights reliability and validity as two key areas of importance for clinicians:
“Reliability and validity of wearable are two potential concerns. HWTs are generally marketed based on the premise that they will play an imperative role in improving thewellbeing – health and fitness – of the people. However, most manufacturers are not in a position to offer “tangible” evidence to prove the effectiveness of these devices.
Comparative studies that have been carried recently on the effectiveness of various HWTs designed for tracking physical activity have illustrated significant variations in terms of accuracy between different devices. The error margin demonstrated by these devices is stated to be up to 25 per cent. This discrepancy is of the highest magnitude hence this reflects the problems wearable medical apps may create..before releasing any HWD to the market as [a] health or medical application, its reliability should [be] carefully address.”
– Integration of Wearable Technologies into Patients Electronic Medical Records (2016)
Philips Healthcare’s IntelliVue Guardian Solution is a patient monitoring system, which uses AI to predict when a life-threatening crisis may occur in a patient for effective, early intervention. The company claims that its Early Warning System combines “software, clinical decision support algorithms and mobile connectivity,” and this is where wearable devices come into play.
For example, a clinician can place a cableless device embedded with sensors on a patient’s wrist to track vital signs such as blood pressure. The IntelliVue Guardian Solution software would use machine learning to identify any significant changes in the patient’s vital signs based on algorithms trained on large datasets of similar patient data. If an important change is identified, data would be transmitted to IntelliVue monitors or mobile devices to notify the caregivers.
In a 2015 independent study evaluating the use of the IntelliVue Guardian System in the waiting room of an emergency department, results showed that “178 patients (79 percent of all participants) were able to be discharged from hospital after their visit to the waiting room” and did not have to be admitted to the hospital. Nearly 70 percent of clinicians who used the system felt that they could easily identify patients who needed immediate medical assistance.
Future of AI Tech in the Medical Device Industry
The effort to make medical devices more reliable, accurate and automated is generating a growing interest in finding ways to integrate AI. Medical imaging is an area that is steadily gaining traction while clinical valid wearables are still emerging. However, developing and improving devices to support the management and treatment of chronic diseases will most likely continue to be a major area of focus.
Americans are living longer so identifying innovative methods of managing care in older adults will become increasingly important. Life expectancy in the United States has seen a generally upward trend, from 69.7 years in 1960 to 78.7 years in 2015.
With patient adherence to treatment being a challenge in chronic disease management, major healthcare companies such as Medtronic are seeing opportunity in automating the process of how patients receive medication. By shifting the responsibility of adherence from the patient to a reliable, automated medical device, there is potential to reduce the economic burden of chronic disease and to improve quality of life.
Looking to the future, data scientist Francesco Corea, PhD, projects that there will be two trends:
- Medical devices integrating AI and virtual reality, and
- The conversion of AI devices for medical applications.
“You already have examples of companies using medical VR devices, but the integration with AI and the real-time feedback (and following adaptation) is not straightforward. The second category I see emerging is devices that are not thought to initially be pure medical devices but that ends up to be such (e.g., biometrics payments, companies human augmentation as value proposition, etc.).
The reason for this second class is that often the commercialization cycle for pure medical devices is pretty long and the efforts (and regulation) huge. Creating something that has a business-viable use case and then looking at the medical implications makes a ton of sense to me.”
As AI becomes increasingly commonplace in the medical device sector, the process for how safety and efficacy are demonstrated will be a significant consideration for clinicians seeking to implement the devices, regulatory agencies and device manufacturers.
Header image credit: SK&A