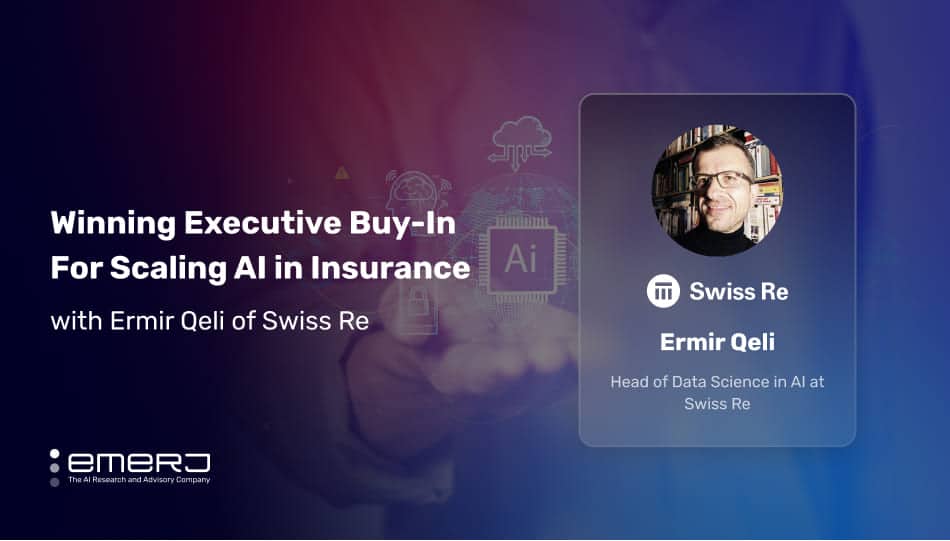
In the insurance sector, integrating AI can significantly enhance customer experience by leveraging advanced technologies similar to those in contact centers.
In a recent episode of the ‘AI in Business’ podcast with Emerj, Jason Aubee, Senior Vice President of Sales and head of North American Revenue at TechSee, highlighted that traditional contact centers face a key challenge: they rely on narrow communication methods like voice calls or chatbots, which limit the ability to understand customer issues fully.
This gap often leads to longer call times and unresolved problems, as agents focus on surface symptoms instead of root causes. This inefficiency can frustrate customers and increase operational costs for businesses.
The Consumer Financial Protection Bureau has harsh criticism of low-grade deployments of AI in customer service workflows (often referred to as chatbots), given the relatively small information portal they have to work with. Still, the bureau estimates that a little over a third of the U.S. population has interacted with a banking chatbot to address serious financial inquiries.
Aubee also suggests that by incorporating visual data and multi-modal communication, AI can expedite issue resolution and improve comprehension, such as visually guiding policyholders through claims processes or troubleshooting insurance-related issues more efficiently.
These insights highlight the diverse ways in which AI is driving innovation in the industry and the challenges financial leaders – especially in insurance spaces – face in trying to help customers with complex problems. From visual data applications that enhance user interactions to advanced language models that streamline data analysis, the potential of AI is reshaping how insurance companies operate and engage with clients.
Emerj Senior Editor Matthew DeMello recently had a conversation with Ermir Qeli, Head of Data Science in AI at Swiss Re, to talk about how advancements in AI, particularly large language models, are transforming the insurance industry by improving the management of unstructured data, streamlining contract analysis and claims processing, and enhancing customer interactions.
From that conversation, we bring you the below two key insights:
- Grounding customer facing AI for improved interactions: Driving context-aware systems to ensure customers are better informed with responses demonstrating fluency in accurate company data.
- Upskilling key users and drive early adoption: Train a small group of key users, demonstrate the value of AI, and build their trust to establish a foundation for broader scaling.
Listen to the full episode below:
Guest: Ermir Qeli, Head of Data Science & AI, Swiss Re
Expertise: Data Mining, Machine Learning, Information Visualization
Brief Recognition: Ermir Qeli joined Swiss Re in 2014 as a Senior Data Scientist and now leads the Data Science and AI division. Over the years, he has played a pivotal role in establishing the company’s first Data Science team and driving the development of Swiss Re’s Data & Analytics Strategy. He holds a PhD in Computer Science from The Philipp University of Marburg.
Grounding Customer-Facing AI for Improved Interactions
Ermir opens the podcast by emphasizing how advancements in AI, particularly generative models like ChatGPT, have transformed the way insurance and reinsurance industries manage unstructured data.
A few years ago, addressing these challenges required extensive data science projects. However, with the rapid development of large language models, AI now offers practical and accessible solutions that were once difficult to imagine.
In insurance, contracts serve as the core product, representing a promise to clients that they will be supported during adverse events. Producing and interpreting these contracts involves significant effort, as they contain complex and detailed terms.
Previously, answering even simple questions, such as whether a contract includes a “war exclusion” clause, required manual effort, or a dedicated data science initiative. Today, AI models like ChatGPT make it possible to query contracts directly, enabling users to quickly access specific information without having to comb through lengthy documents.
These AI advancements also extend to claims processing, which involves unstructured data like emails, phone calls, and other customer communications. Efficient analysis of this data is critical for providing timely and effective customer service. With current AI capabilities, insurers can now process and analyze large volumes of claims data at scale, speeding up response times and enhancing customer satisfaction.
Overall, AI’s ability to handle unstructured data is revolutionizing how insurers manage contracts and claims. By streamlining these processes, the industry is not only improving operational efficiency but also delivering better experiences to its clients.
“The most popular use cases of larger language models in financial services at large and insurance-specific are found around a better customer experience, a better call center experience, a better chatbot experience. We all recall our chatbot experiences prior to ChatGPT; the answers seemed rigid, and you had the impression that, actually, you spent more time than if you searched this stuff yourself. And ultimately, they did not work so well. Now, when you check ChatGPT, most of that interaction seems seamless.
The answers, of course, have to be controlled and have to be grounded in the data that an insurance company provides. But in a nutshell, that process has become simpler. And that, of course, will also change the way how the retail insurers interact with their customers.”
—Ermir Qeli, Head of Data Science in AI at Swiss Re
Upskill Key Users and Drive Early Adoption
Ermir then discusses the challenges of scaling AI solutions and achieving widespread adoption, emphasizing that building prototypes and running small pilots is relatively easy, but scaling these solutions to generate meaningful business impact is difficult. A significant hurdle is change management, as it requires convincing stakeholders to adopt new processes and approaches, which can be inherently resistant to change.
He stresses the importance of involving business stakeholders from the very beginning (“day zero”) to manage this change collaboratively. Starting from “day zero” includes upskilling users, gaining their trust in the technology, and helping them understand how it works.
Using his personal experience with ChatGPT, Ermir explains how initial skepticism about new technology can evolve into widespread usage once its value is clear.
For businesses, achieving critical mass in user adoption is essential to unlocking the full potential of AI. He notes that even the most advanced models will fail to deliver business impact if they are not used effectively for their intended purpose.
In the end, Ermir highlights that the ultimate goal of implementing AI or new technologies is achieving business impact. However, projecting future implications can be challenging due to reliance on assumptions. While forecasts can help, they are inherently uncertain, especially for new processes or technologies.
To address this, he suggests focusing on proxies for business impact — indicators that signal potential value even before full-scale adoption.
One key proxy is individual user adoption: if users start integrating the technology into their workflows, it’s a positive sign of its potential impact. However, adoption can mean something other than scaling to thousands of users immediately. Even a small group of key stakeholders using the technology effectively can drive meaningful results and set the stage for broader success.