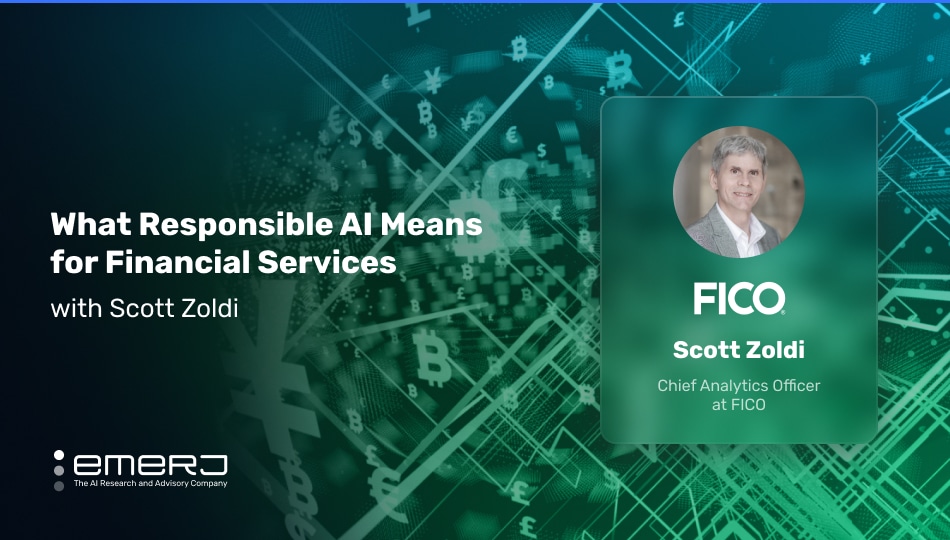
Implementing responsible AI in the financial sector is crucial for ethical practices, fairness, and transparency. Financial institutions must prioritize data privacy, address biases, ensure explainability, and practice ongoing monitoring. By doing so, they build trust, mitigate risks, and foster sustainable growth.
If only it were that easy. As a new report from FICO on the state of ethical AI practices in financial services illustrates, many companies are ill-prepared to reinforce ethical considerations in their AI adoption initiatives.
In particular, a survey of over 100 executives in the report found startling findings: while 52% of respondents report that they are a higher priority than 12 months ago, 43% of organizations struggle to make their AI governance structures and processes meet regulatory requirements.
AI systems heavily depend on data created or collected by humans. This data can include user-generated content, information from online platforms, or data captured by sensors and devices. Any biases that exist in humans, intentional or unintentional, can find their way into these AI systems through the data they utilize and pave the road to unintended and disastrous results in unsanitary data governance practices.
On Emerj’s ‘AI in Business’ podcast, Senior Editor Matthew DeMello recently sat down with Scott Zoldi, Chief Analytics Officer at FICO, to discuss the report, what responsible AI means for financial services and the guidelines to implement ethical AI in the company.
Responsible AI involves robust data protection measures, unbiased decision-making, clear explanations, regular audits, and adherence to ethical frameworks. However, it is challenging and requires a comprehensive framework and diligently following guidelines.
In the following analysis of their conversation, we examine two key insights:
- Critical considerations for ethical AI: Building considerations for the robustness, explainability, ethics, and audibility of AI systems at their initial adoption phase.
- Steps to implementing ethical AI: Surveying AI use, developing guidelines for selected methodologies, and aligning with a model development governance standard to implement ethical AI.
Listen to the full episode below:
Guest: Scott Zoldi, Chief Analytics Officer at FICO
Expertise: Fraud analytics, cybersecurity, explainable and ethical AI, unstructured data analytics, unsupervised machine learning and utility analytics
Brief Recognition: A seasoned technology executive with over 25 years of experience in AI, machine learning, and advanced analytics, Scott Zoldi leads the development of innovative analytics solutions for FICO’s clients. He holds a Ph.D. in computer science from Duke University and is a member of the Forbes Technology Council. He has 120+ authored patents, with 80 patents granted and 47 patents in progress.
Critical Considerations for Ethical AI
In citing a survey in the new FICO report ‘State of Responsible AI in Financial Services’, Scott mentions that only 8% of companies have achieved AI maturity. He suggests that this is could because of a couple of factors:
- Lack of a single, consistent playbook for responsible AI in financial services
- The need for a unified standard to be applied across the entire organization
- The constant bombardment of AI hype diverts attention from building robust, explainable, ethical, and auditable models.
Scott emphasizes the need to consolidate AI governance under a solitary and clear framework:
“There aren’t many playbooks for this. I think there are parts that financial services organizations understand, but they really need to organize themselves around a standard, which would be applied across the entire financial services organization, and that takes energy. There’s a lot of different models being developed, along with lots of different opinions. And so this one model development governance standard, which the company will embrace and develop their AI around is really hard. It’s very transformative.”
– Scott Zoldi, Chief Analytics Officer at FICO
Scott further shares that he has observed that many financial organizations say that their C-level executives and the board need to understand the importance of responsible AI. He feels these executives want to experiment and invest in deep learning but doing so requires thinking of responsible AI to be ethical.
Another finding from the report is that 44% of the executives responded that responsible AI strategies still needed to be defined at the board level. Dr. Seth Dorbin, CEO and Board Member of Trustwise AI and former IBM Global Chief AI Officer, can attest to the report’s findings from his personal experience. He tells Emerj he wouldn’t stop there:
“I agree and would even go a step further with this gap and say very few are established at the board level, and only a handful understands the importance or ramifications of their lack of understanding. It’s a very significant blind spot for most boards. With that said, there are multiple PE firms that I am aware of who are forcing this conversation at the board level for their portfolio companies.”
– CEO and Board Member of Trustwise AI and former IBM Global Chief AI Officer Seth Dobrin
Taking the opportunity, Zoldi talks about what a board-defined responsible AI strategy looks like. He outlines the four critical factors for building accountable and ethical AI models:
- Model Robustness: Models must be robust and capable of functioning in a production environment where data may shift and behaviors can change. Making robust models requires thorough testing and validation to ensure stability and reliability.
Scott points out that some people use auto ML or spend some data through an open-source trainer, and overnight, they call what they’ve developed a model. Instead, models that have an impact on people take much more work to be fully understood, even by the teams developing them:
- Model Explainability: Models must be explainable and interpretable to make the decision-making process straightforward and transparent. It is essential because we must understand what the model is learning and what drives those decisions.
Scott refers to explainable AI algorithms as good, bad, and ugly because every model provides different answers. But the one where the data scientist, a governance team, and a regulator can look at what drives the model and ask questions about the decision’s reliability is important.
- Ethical Models: Models must treat individuals fairly, with the potential impact on different consumer groups being considered.
Scott says that in case of the number of late payments or the total mortgage balance, the model’s decision will have a more significant impact on some types of customers than others. He believes this to be an ethical question the model should know about.
- Auditable Models: Models must be auditable, with a transparent process for responsible development and monitoring in a production environment. He believes creating accountable and ethical models in labs only matters once it starts impacting humans.
Hence he suggests having a monitoring system in place so we know when a model behaves differently than we had anticipated when it passed all the checks during development.
Steps to Implementing Ethical AI
Before implementing ethical AI, enterprises need to define what ethics are for their company and their solutions.
In another podcast episode of the AI in Business podcast, the Executive Director for the Global Deloitte AI Institute, Beena Ammanath, noted that there has been a notion about AI ethics being all about transparency, removing bias, and making it fairer. She feels those are only catchy headlines:
“In my experience working across different industries, fairness, bias, and transparency are crucial. But there are other factors. For example, if you have an algorithm predicting a manufacturing machine failure, fairness doesn’t really come into play. But security and safety are both key issues.”
– Executive Director for the Global Deloitte AI Institute Beena Ammanath
For best practices in developing in-house AI capabilities within an organization, Zoldi advises Emerj’s executive audience on adopting the following three-step framework:
- Survey the current methodologies.
- Develop a set of guidelines should be developed by either a chief analytics officer or an ethics officer, focusing on a few methods the organization will adopt.
- Help to mature current practices by aligning with a model development governance standard to which all future AI and machine learning initiatives will align.
By having a singular approach like this, the organization can better focus its efforts and bring the focus of its data science teams to one particular problem.
He further the impact of the pandemic on financial services organizations and the growing importance of AI regulation. During the pandemic, many consumers have shifted to digital interactions with financial services organizations, and the ability to personalize those interactions based on the individual’s economic history is critical. However, with the increased use of AI, there is also an increased need for regulation, which the US needs to catch up on compared to Europe’s GDPR and high-risk AI definitions.
The executive order on advancing racial equity and the AI Bill of Rights in the US are recent examples of increased focus on AI regulation. Consumers are becoming more informed about the impact of AI on their decisions and are increasingly concerned about the appropriate use of AI, including data privacy and protection against discrimination.
Organizations that differentiate themselves by using AI appropriately and meeting consumer concerns around data privacy and algorithm protections will be more successful.
Scott tells Emerj that the first step in educating organizations about the importance of responsible AI is to make them aware of its potential dangers and risks. Many advanced organizations have already caused severe mistakes in how they designed their systems, resulting in biased, inappropriate, offensive and plain incorrect information being reported. Organizations need to ask themselves if they want to be in such positions and realize the potential consequences if they don’t act responsibly.
Additionally, it’s essential to remind organizations that AI models are just tools and that they can choose more socially responsible options that prioritize ethics, fairness, and safety over raw predictive value. Making interpretability, explainability, and ethics job number one should be a core part of an organization’s DNA when implementing AI.