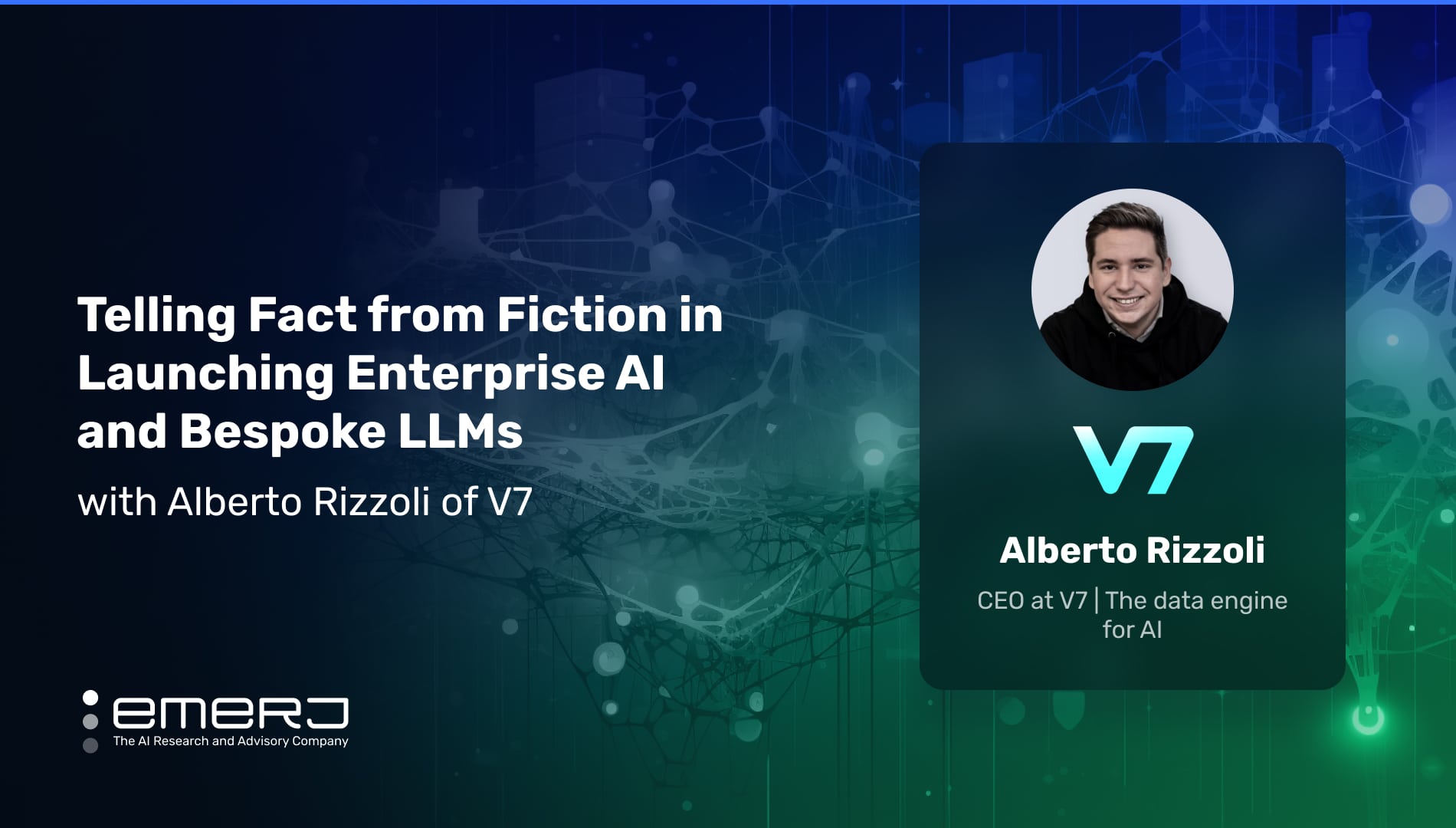
Navigating AI’s challenges in transforming critical business operations applications are two pivotal aspects driving what is becoming a right of passage for nearly every industry in the evolution of modern enterprises. As organizations strive to harness the power of AI, they encounter intricate challenges, from data extraction to the complex task of molding AI into a tool that enhances various business functions.
Simultaneously, the transformative potential of AI – particularly new cutting-edge tools in generative AI, including large language models (LLMs) – in business applications holds the promise of reshaping industries across the board. These capabilities offer a new dimension of efficiency and productivity, from user-facing software co-pilots that enhance user interactions to analyzing massive datasets for strategic insights.
Emerj Senior Editor Matthew DeMello recently spoke with Alberto Rizzoli, Co-Founder & CEO of V7 Labs, on the ‘AI in Business’ podcast to discuss AI’s potential and challenges, revenue-driven applications. They also delve into AI’s transformative applications, highlighting software co-pilots, data analysis, and human collaboration while addressing AI’s limitations and the significance of accurate training datasets.
In the following analysis of their conversation, we examine three key insights:
- Engaging the entire organization in generative AI model development: Building a knowledge bank in collaboration with all the employees to grasp the business intricacies rather than confining it to the machine learning team.
- Addressing core business problems with smaller, bespoke models: The advantages of deploying smaller models or fine-tuned versions of GPT 3 over foundational models for specific business-critical workflows.
- Finding ROI in generative AI initiatives from tracking existing human workflows: Utilizing AI to enhance data analysis, streamline tasks, and improve user experiences while acknowledging the need for human expertise to address limitations and unfamiliar situations.
Listen to the full episode below:
Guest: Alberto Rizzoli, Co-Founder & CEO, V7 Labs
Expertise: AI, deep learning and computer vision
Brief Recognition: Alberto is an Italian-born entrepreneur and Co-Founder of V7. He has a BSc in Management from Cass Business School, and in 2015, he joined Singularity University’s Graduate Studies Program at the NASA Ames Research Park under a Google-funded scholarship. In March 2016, Alberto was awarded by the President of Italy, Sergio Mattarella, for advancing artificial intelligence for a good cause.
Engaging the Entire Organization in GenAI Model Development
Alberto Rizzoli begins by addressing the desire among business leaders across sectors for an all-purpose GPT-like model that automatically and intimately comprehends specific business problems with minimal training.
Alberto reassures the Emerj podcast audience that such AI capabilities are possible to bring to the enterprise in the advent of generative AI. However, obstacles arise due to the complexities of extracting data from a company’s tech stack, private intranet and other security concerns. Despite these barriers, ongoing efforts are directed at developing tools to tackle these issues.
Throughout the episode, Alberto underscores the importance of identifying suitable problem areas for large language models, along with the challenge of constructing an internal data structure that allows employees to contribute their insights.
Rather than confining model development to the machine learning team, he envisions a future where all employees collaboratively build a collective “knowledge bank,” augmenting the model’s grasp of business intricacies.
He continues to focus on the factors contributing to generating revenue within a business. He stresses that the primary recommendation for applying AI lies in addressing the most significant revenue-generating issues. This recommendation extends to various industries, including financial services and other sectors like insurance and manufacturing.
Rizzoli metaphorically compares AI to a “nuclear hammer” that can be applied to various problems but may not work effectively unless fine-tuned. While he acknowledges the impressive capabilities of AI, he highlights the challenge of tailoring it to specific issues at the heart of business value for the organization. In the process, achieving high accuracy – especially reaching 99% – proves difficult, and replacing human functions with AI is even more challenging.
He recommends looking at customer and employee problems with tremendous business value as the possible basis for The speaker identifies the most valuable issues may be just as intricate to solve.
Still, they serve as the basis for and can act as co-pilot applications that can work alongside humans or employees, assisting with particularly complex tasks. This approach can be applied in various contexts, from helping individuals complete complicated forms to supporting surgeons in challenging tasks like keyhole surgery.
Alberto advises product leaders and business executives to consider how their products can be transformed by imagining the most competent user sitting beside the customer, guiding them on interactions and actions.
This potential is what large AI models can offer, not just in conversational interactions but also in interpreting images and performing tasks like providing sales forecasts. These functionalities can readily be integrated into products today.
Addressing Core Business Problems with Smaller, Bespoke Models
Alberto advises business leaders to fight against the temptation to immediately employ large foundational models like GPT-4 or MedPOM for problem-solving purposes yet emphasizes the importance of understanding successful implementations at a deeper level.
He points out that, more often, smaller bespoke models – even using a finely-tuned version of the more primitive GPT-3 model, a minor language or vision model – are much more effective for well-defined problems than deploying foundational models from the offset.
He points out that some successful implementations today are utilizing foundation models. This counterintuitive approach suggests that using a finely tuned GPT-3 or a minor language or vision model for well-defined problems is often more effective than opting for larger models like MedPOM.
In deploying smaller, bespoke models, Alberto tells the podcast audience that the focus is on addressing specific issues in practical business contexts, such as manufacturing. For instance, on a production line, the priority is to detect defects, not read or analyze Shakespeare accurately. Smaller models boasting 99% accuracy still are the most reliable choice for this task.
However, Alberto foresees large models improving progressively, potentially surpassing smaller models in specific functions, provided the computational resources are available, although they might remain resource-intensive. The speaker advises using large models during prototyping, especially in cases where they’re beneficial. Still, beyond that stage, they recommend consulting data scientists to determine whether it’s pragmatic to continue with these expensive, large models that offer versatile capabilities.
Ultimately, he compares these choices to using a Swiss Army Knife versus a precise screwdriver, suggesting that the right tool should be chosen for the specific task.
Drawing from the concept outlined in the book Thinking, Fast and Slow, Alberto tells Emerj that V7 has drawn a parallel between the brain’s two modes of thinking and the realm of deep learning models.
These models, such as object detection and text classification, resemble fast thinking and are capable of swiftly recognizing offensive language or identifying objects. Conversely, the slower thinking mode is exemplified by expansive language models that serve as orchestrators, connecting various tools, including smaller models and conventional software.
Finding ROI in GenAI initiatives From Tracking Existing Human Workflows
He discusses the successful applications of LLMs. One practical application is the development of user-facing software co-pilots that transform software interactions into conversations. He highlights Shopify’s “sidekick system” as an exemplary case where the software acts as a co-pilot, guiding users on entrepreneurship and software usage.
However, the speaker notes that while this approach works well for businesses like Shopify, it might only suit some enterprises. For most companies, especially those without products like Shopify’s, one of the most promising returns on investment with LLMs lies in analyzing large volumes of data. In scenarios like hedge funds combing through extensive documents, these models can efficiently process and provide coherent responses based on queries.
Rizzoli emphasizes high ROI is observed in back-office processes and certain user-facing functionalities. Still, the latter demands a team well-versed in the user experience of large language models. The speaker suggests waiting and observing how others lead in this field for those without such expertise.
“So one piece of advice I would always give is never alienate your humans; ultimately, both the people that will teach the AI will use the AI, and they will have to be good friends. So the implementation of AI should always be seen as a way of supercharging the talent that is already there.”
– Alberto Rizolli, V7 CEO
The speaker notes that AI’s performance is tied to the quality of human training, with AI essentially learning from imitation. As AI becomes co-pilots to humans, they must handle mundane tasks. It might involve tasks like document processing or data extraction. Determining the tasks where human intervention is needed presents a challenge but is essential.
These areas, where AI needs human expertise to improve, become valuable sources of unique data and intellectual property that give a competitive edge. Just as data collection is vital, addressing AI’s limitations with human skills leads to continuous AI improvement, making it a crucial resource.
He provides an example within the manufacturing context to illustrate his point. He discusses a scenario where an AI encounters an anomaly it’s unsure about, possibly due to an unusual event that wasn’t part of its training data – like a scratch on silicon caused by an unexpected incident. He mentions that the model should ideally be able to detect whether such examples are outside its training distribution and assign a reliable confidence score.
Instances that the model cannot confidently handle should be routed to humans for further assessment. The assessments can be managed through software where humans are notified to review and tag images for retraining. He mentions that a similar consensus system can be used in medical cases, where human agreement with the model’s output is required before releasing results.
Alberto emphasizes the importance of involving humans when data is outside the model’s training distribution, highlighting that AI is proficient within its training scope but tends to guess when faced with unfamiliar situations. The critical takeaway is to not solely rely on AI, particularly for cases beyond its training scope, and to involve humans to expand the model’s knowledge and distribution of data.
Lastly, Rizzoli delves into the concept that AI’s fundamental goal is to persuade humans that its answers are correct. He emphasizes that achieving humility was challenging and is still being explored. His primary advice is understanding the data distribution, visualized as a bell curve, to avoid errors.
Tasks usually fall within the curve’s middle range due to their repetitive nature. Anything outside of this range should be treated carefully by the model. It can be classified by including relevant prompts or relying on the model’s confidence score or visual characteristics to spot unusual image elements.
“It is to be remembered that once something enters a training set and contains an error rarely makes its way out. And your AI will be forever stunted by this bad training set example. So training data needs to be religiously manicured when created and given the right level of respect because it can become a speed bump you’ll never detect afterward.”
– Alberto Rizolli, V7 CEO