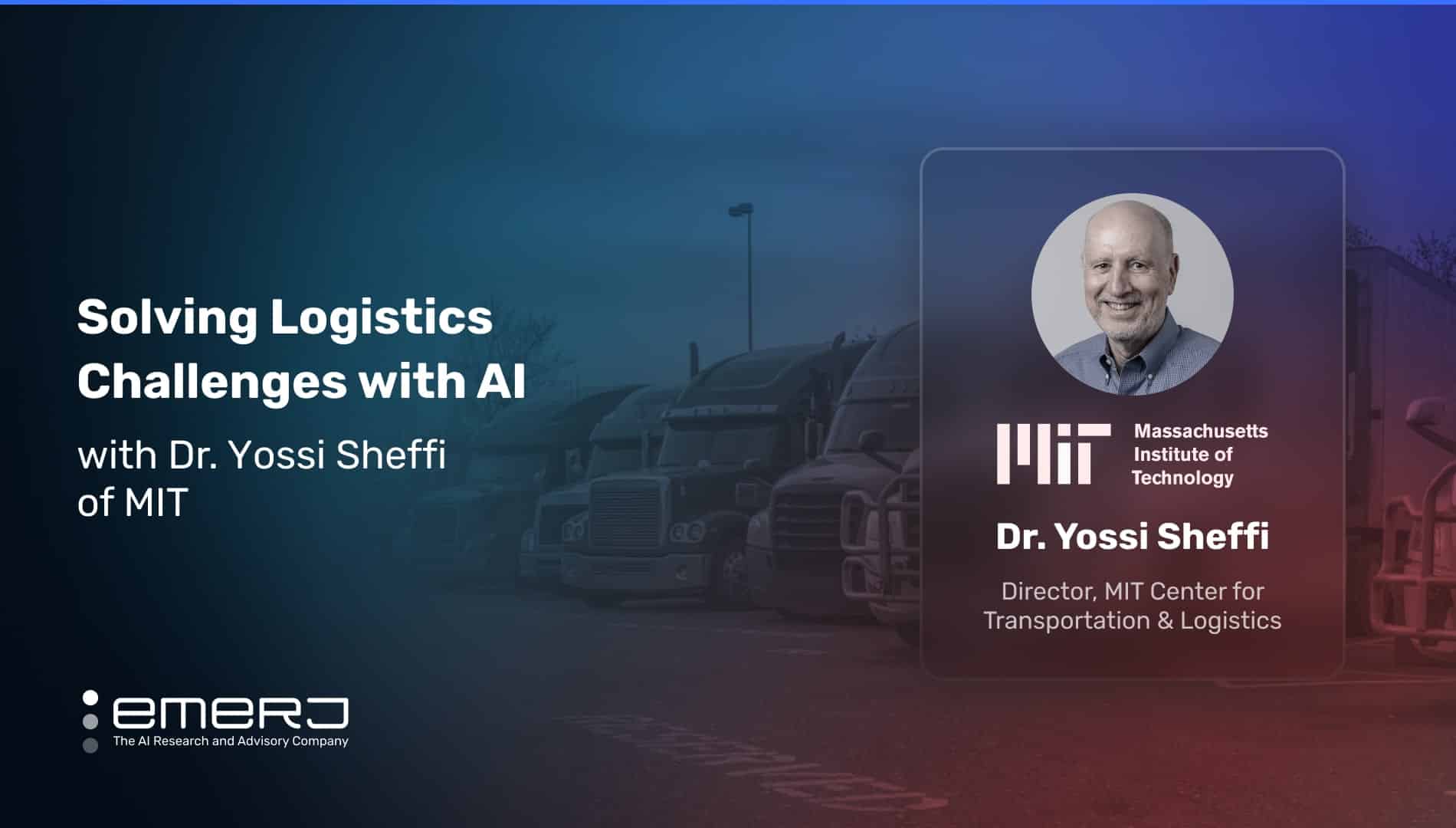
AI is driving change across many industries, including logistics and manufacturing. Companies need to strike the right balance between automation and human intervention. This interaction represents a specific area of study known as human-automation interaction.
Social media platforms represent a significant source of potential data for companies, especially considering the many users many platforms have. As recently as 2023, Facebook had 3 billion users, and that number is growing.
According to the Harvard Business Review, AI won’t replace humans, but rather, humans using AI will prevail over humans who don’t use AI. How do organizations redefine their workflows by optimally combining AI with human judgment? We address that concern in this article.
Emerj Senior Editor Matthew DeMello recently sat down with Dr. Yossi Sheffi of MIT on Emerj’s ‘AI in Business’ podcast to discuss how AI can solve logistical challenges. In their 30-minute interview, the pair discuss challenges in logistics and manufacturing, such as lack of data due to visibility in supply chains and trust issues as a hurdle to accepting automation.
This article will examine three key insights from our conversation with Yossi relevant to leaders in logistics and manufacturing interested in implementing AI:
- Tracking real-time data on manufactured products: By using sensors, manufacturers can immediately send data to the digital twins of their products.
- Recognizing challenges of using robots: Properly implementing automation with robots requires a change in workflows, a combination of human input, automation, social acceptance and trust.
- Using social media to enhance risk management analysis: Large language models can analyze social media content to help companies manage risk and anticipate future problems.
Listen to the full episode below.
Guest: Dr. Yosi Sheffi, Professor of Engineering Systems and Director of the Center for Transportation and Logistics at the Massachusetts Institute of Technology.
Expertise: Supply chain, risk analysis, logistics management
Brief Recognition: Author of The Magic Conveyor Belt: Supply Chains, AI, and the Future of Work.
Tracking Real-Time Data on Manufactured Products
Obtaining real-time data in logistics and manufacturing is an ongoing challenge. Sheffi mentions how this is still a challenge despite the prevalence of the Internet of Things and the ubiquitous use of sensors. As a result, companies in logistics and manufacturing don’t have access to the needed data to analyze.
He highlights that the data companies are most interested in is data from today; they want to know what is happening with products or shipments en route to their destination. Companies need complete visibility beyond the first two tiers when dealing with suppliers.
When discussing beyond the first two tiers of suppliers that a company interacts with, Sheffi conceded, “In many cases, you don’t even know who they are. So, it’s hard to have complete visibility, and it’s still a challenge.”
Contract manufacturers who sell to other businesses have different considerations when implementing AI than manufacturers who sell directly to consumers. Dr. Sheffi discussed how contract manufacturers use AI-infused programs to build designs. The program takes an initial setup and works on it to improve it iteratively. As Dr. Sheffi explains, “It’s basically a conversation between the AI and the designer.”
The design process is guided by the concept of digital twins, a technological breakthrough that has revolutionized manufacturing. Digital twins foster continuous data connectivity between a physical asset and its digital representation, thereby enabling manufacturers to monitor a product they manufacture throughout that product’s lifecycle. Sheffi differentiated between digital twins and device simulation. “The difference is they allow continuous data connections between the physical asset and the device.”
Recognizing the Challenges of Using Robots
Dr. Sheffi expands upon the challenges of integrating robots into logistics and manufacturing. He explains how warehouses installed robots even before the pandemic and how integrations accelerated significantly after the pandemic. Sheffi also mentions how autonomous trucking is on the horizon and will precede autonomous cars.
Successfully implementing automation is a complex task. Automation and human input are complementary. Industry leaders must prioritize integrating automation into workflows; Amazon represents one example.
Dr. Sheffi describes how Amazon put robots in their warehouses and then hired a million warehouse workers. Sheffi explains how it’s essential to consider how to:
- Train people to work with different workflows
- Train people to operate the robots
- Train people to use autonomous devices
Even after workers are provided opportunities for relearning and upgrading skills, Sheffi mentions how the issue of social acceptance remains. This social acceptance is primarily based on trust and safety.
However, concern about worker replacement is always considered when introducing robots. MIT documented that worker resistance in Europe has kept the adoption of new technology the same because Europe has protections that limit layoffs. It’s not yet evident if the US will follow suit.
Sheffi also mentions how autonomous trucking is on the horizon and will precede autonomous cars. However, autonomous trucking will still require a level of trust that will take a lot of work to achieve. Sheffi asks, “Would you feel comfortable when there’s a big truck with no driver driving behind you on the road?”
He continues describing how the A350 aircraft is a drone and can fly independently. However, he recognizes that most people would not feel comfortable on a plane with no pilot in the cockpit. Even though autonomous technology exists in the aviation industry, human pilots are still needed to reassure passengers.
Using Social Media to Enhance Risk Management Analysis
Sheffi emphasizes how important it is to emphasize forward-looking information in risk management analysis, and he fully recognizes the fact that media sources can provide valuable insights.
Sheffi explains that companies conduct risk management analysis to determine the chance that a supplier will have a problem. He comments on how looking at information such as financial releases comprise backward-looking information, and he further reinforces that companies need to look at forward-looking information, or the very least, current information.
Sheffi offers, “It turns out that one of the best indicators comes from just media.”
Multiple valuable insights can be pulled from social media. The media might mention a particular company repeatedly or have information about an executive leaving a company. Posts on various social media channels can also describe a problem a company has with a bank or a failed merger and acquisition. It also often includes complaints from dissatisfied customers.
The original equipment manufacturer can analyze this information using large language models (LLMs). Media surveillance increases the likelihood that manufacturing leaders can identify supply chain problems.
Sheffi highlights how companies can strategically use chatbots to address concerns and influence public perception. For example, companies can take it one step further by using chatbots to address the negative concerns on platforms. To this extent, they can also influence the marketplace by their responses.
In our discussion with Sheffi, we touched upon the extent to which manufacturing leaders should be thinking about conversational AI. Manufacturers who directly sell to consumers need to hear the voice of the customer and analyze it. Fortunately, they are well-positioned to harness conversational AI. They also can proactively identify issues before safety regulators do. However, he explains that contract manufacturers who build for other companies do not need to worry about conversational AI.
The sheer amount of data customers produce can be overwhelming for human workers to analyze. That is why using AI to capture the voice of the customer by effectively analyzing customers’ emotions, intent, and sentiment. Notably, Harvard Business Review found that companies with the tools to analyze qualitative data from social media can eliminate quantitative customer surveys.
“So things like this are happening simply because of the ability to analyze things that are not numbers. So, text, for example, or the ability to look at an immense amount of data and put it all together.”