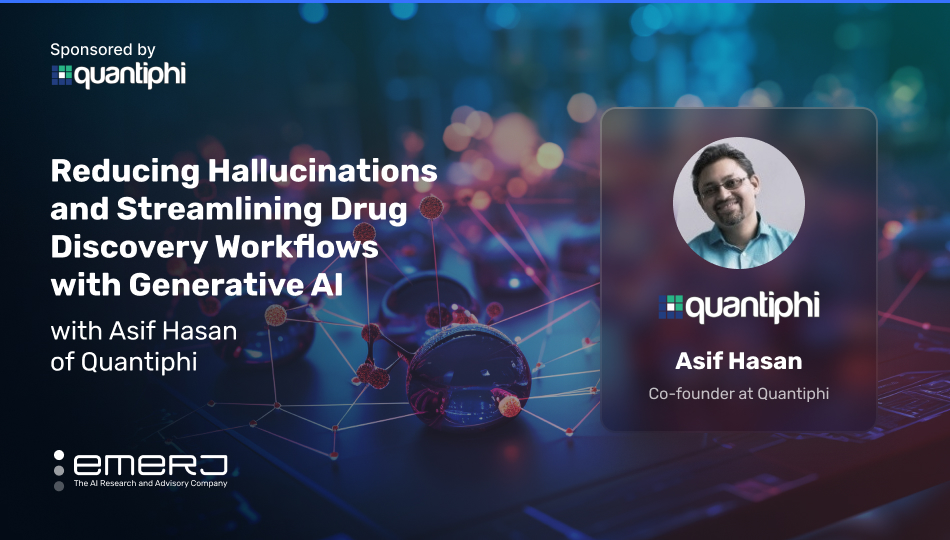
This interview analysis is sponsored by Quantiphi and was written, edited, and published in alignment with our Emerj sponsored content guidelines. Learn more about our thought leadership and content creation services on our Emerj Media Services page.
In the advent of OpenAI’s success with the enormously popular ChatGPT platform in late 2022, the world came to understand that generative AI (GenAI) is fundamentally different from the AI capabilities that came before it.
In terms of technological development, introducing an improvement that reduces the power, cost, labor, and compute needed to complete a task is referred to as a step-change improvement. The step-change improvement that ChatGPT introduced to the world is that the previous AI capabilities – machine learning, predictive analytics, optical character recognition, etc. – could be applied to subjective questions without predetermined answers.
Tasks that were previously deemed only the domain of humans with feelings, complex viewpoints, and creativity could suddenly be automated and streamlined through software. While the subsequent identity crisis for information workers across the world has been apparent, the added risk associated with these probabilistic technologies has been even louder.
Nowhere is the risk more significant than in proximity to the healthcare and life sciences space – where not only are regulatory agencies and international organizations taking a closer look at AI deployments, but the prospect of automated healthcare itself represents almost endless ethical difficulties and concerns.
In turn, GenAI is a substantial economic driver behind how the lines between healthcare and life sciences spaces are becoming increasingly blurred. As Athenahealth Chief Medical Officer Dr. Nele Jessel mentioned during her appearance on Emerj’s ‘AI in Business’ podcast last year, life sciences firms are finding patients are turning to clinical trials more than ever as a form of alternative care as more powerful technologies are used to develop more advanced drugs for more highly targeted patients.
Emerj CEO and Head of Research Daniel Faggella recently sat down with Asif Hasan, co-founder of Quantiphi, on the ‘AI in Business’ podcast to talk about the promise of GenAI, LLMs, and what life sciences leaders can do to prepare their organizations for a future defined by their capabilities.
- Identifying step improvements in life science use cases: A three-factor criteria for assessing features of life sciences use cases that forecast more significant changes for the industry.
- Fighting hallucinations in model development: The essentials of using tools like retrieval augmented generator pipelines to reduce hallucinations in life sciences model development.
Listen to the full episode below:
Guest: Asif Hasan, Co-founder, Quantiphi
Expertise: Machine learning, Big Data and Risk Modeling
Brief Recognition: Asif co-founded Quantiphi 10 years ago as a deep learning and artificial intelligence solutions startup. He has broad experience in machine learning, including computer vision, speech recognition, natural language understanding, risk modeling, churn prevention, supply-chain optimization, predictive maintenance, customer segmentation, and sentiment analysis.
Identifying Step Improvements in Life Science Use Cases
Asif begins his podcast appearance with a reasonably typical sounding claim on the surface that contains a multitude of consequences for the life sciences space going: What makes GenAI and large language models genuinely unique in the development of business technology is that they represent a step improvement in a wide range of business functions across life sciences.
To borrow Asif’s words, these are “problems that were previously thought of as too expensive or seemed impossible to achieve are now much easier for organizations to visualize and achieve.”
Specifically, large language models aim to streamline drug development by leveraging genetic data and AI-powered workflows through advanced capabilities like providing clinical researchers with a deeper understanding of protein and molecule structures. Asif tells the executive podcast audience that he expects these changes alone will revolutionize life sciences departments with new norms for commercialization, manufacturing, and clinical trials.
Because the deluge of life science use cases will be difficult for executives to evaluate, Asif talks about an initiative at Quantiphi conducting discovery workshops with over 100 organizations across industries to try and anticipate these new industrial norms. Three features became prominent from the use cases discovered in the process:
- Nearing human levels of performance: Asif gives the example of a Google Metaponto system that can pass the US medical licensing exam at an expert level. Subsequently, it is evident that a variety of tasks involving advanced human expertise are bound to become managed by a supervised LLM.
- Repetitive and time-consuming tasks: Asif emphasizes that GenAI not only expedites the most monotonous and manual tasks but also reduces spend on tasks whose costliness depends on their repetitive or time-consuming nature.
- Simple natural language prompt: If a task can be broken down into a simple set of language-based instructions.
Asif and the Quantiphi team found that if any tasks contained a confluence of these three factors, they were able to uncover highly feasible and impactful use cases that had great potential for becoming new industrial norms.
As an example, Asif notes that in pharmacovigilance, LLMs can detect adverse events at a fraction of the cost and accuracy levels, potentially replacing human agents. He notes that most tier-one farmers encounter over 1 million adverse events for therapies that they have in the market annually, of which only 15% are described in medical literature.
Searches through this information are manual and can take a few hours for a single human, but can easily be modeled and duplicated so the same task can be performed at a fraction of the cost: 50-60 cents as opposed to $40-50 with far higher accuracy levels.
Elsewhere in the life sciences value chain in clinical research, Asif says that LLMs can similarly assist in synthesizing scientific literature. He notes that the most prominent example is in biomarker discovery, where LLMs can screen through vast datasets to identify patterns associated with specific conditions. On the administrative side, LLMs can monitor, update, and maintain regulatory requirements, even automating processes for companies.
Throughout listing these examples, Asif emphasizes that, so long as the use case satisfies those three conditions, they stand to represent new industry norms.
Fighting Hallucinations in Model Development
Unlike their first-generation deterministic counterparts among AI capabilities, GenAI is marked by fundamentally probabilistic technologies. The numerous issues surrounding the accuracy and transparency of these technologies – often referred to in the media as “hallucinations” – disseminate from the fact the it functions by guessing what response will get positive feedback from the user based on training and context data.
To fight hallucinations and other flaws in probabilistic systems like many forms of generative AI, Asif first recommends that leaders ground their prompts “in the facts that are related to that question.” To accomplish this, he instructs business leaders on the essentials of what he calls a ‘retrieval augmented generator pipeline.’
He offers a conversational explanation for how the system works:
“What we are telling the language model is, ‘Forget all of your knowledge in this domain that you have, I will give you some context. Then, I will give you a prompt, and you read only the context that I give you and then ground your response based on that context that I provided…
Once you do that, you’re essentially saying that I will give you the knowledge that you need to know, and I’m going to retrieve it based on my retrieval mechanisms. Then I’ll give you that knowledge, and now you synthesize your response based on this context.”
– Asif Hasan, Co-founder at Quantiphi
Asif notes that not only does grounding the response in the proper knowledge make it much more difficult for the LLM to hallucinate the response, but users can always trace back to their original context to see what went wrong. Even while a world without hallucinations is still a long way off, if even possible, the increased transparency of the system with retrieval augmented generator pipelines helps to build organizational trust.
In the context of clinical trials, where life sciences workflows face the most significant and most direct exposure to patients, Asif recommends that life science leaders adopt three requirements for developing models with maximum accuracy:
- Be intentional about your vision for Gen AI programs: “What does success look like for your organization? Is it purely about cost optimization?” Asif asks the podcast audience. “Is it about finding new markets? Is it about expanding into existing markets? Is it about improving time to value?”
- Much of responsible AI is good data governance: The majority of unintended negative consequences arise if the data being used by Gen AI initiatives are not governed appropriately. Leadership needs to have a clear understanding of risks in managing data governance decisions.
- Identify the champions: Find the stakeholders who are “true believers” and are willing to let teams experiment, learn, and evolve. Asif emphasizes that GenAI leadership teams develop a robust governance structure for data security, ethical considerations, and IP protection.
Once these requisites are satisfied, Asif recommends that organizations consider the following steps for maintaining trustworthy life sciences models:
- Partner with experienced advisors: Finding tested consultants helps in navigating the complex landscape of AI adoption, deciding which trade-offs in tech stacks are suitable for your organization, planing talent development, and establishing a center of enablement.
- In-house skill development and change management: As life sciences organizations grow alongside these models, Asif recommends that leaders develop teams of specialized LLM engineers and architects that can provide platform and personnel continuity across initiatives. These advisors can also help in change management decisions, such as the art of what Asif calls “pumping LLMs broadly through the organization.”
- Establish a Center of Enablement: To center these capabilities, Asif tells the podcast audience that they’ll need to establish a center of enablement so organizations “can address a broad range of opportunities in a repeatable and scalable way.”