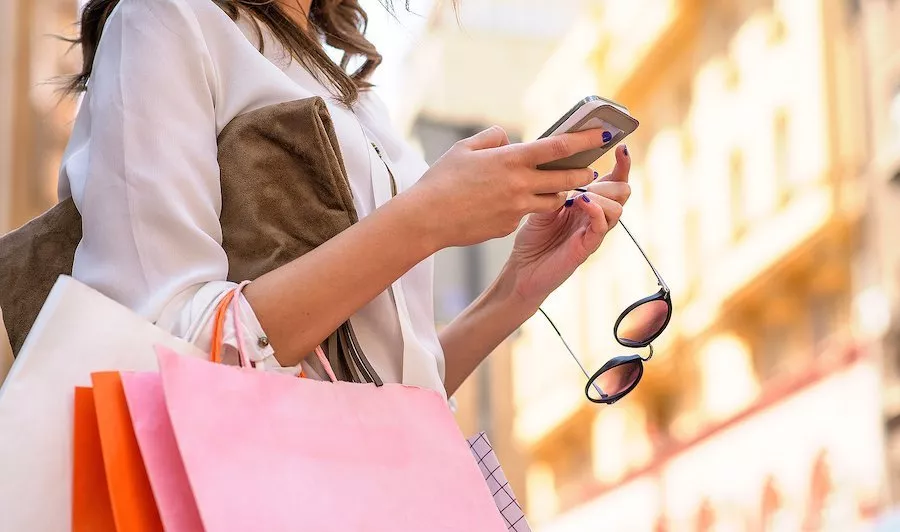
In 2013, a McKinsey report noted that over 85 percent of Amazon sales revenue was generated from personalized recommendations. Since then, the use of personalized recommendations has grown. On average, today’s personalized recommendations account for 27-percent of retail site revenue, according to a recent Salesforce report.
With the development of AI-powered recommendation engines, recent research from Emerald Insight also shows that recommendation engines are becoming more common in the areas of fashion and apparel.
Through our research, we’ve found that current applications claim to allow shoppers to scan clothing, footwear and fashion accessories in order to receive wardrobe suggestions. Shoppers could also receive recommendations based on buying patterns and fitting requirements through various applications.
While some recommendation engine applications are standalone, other applications, with strong affiliations to maquis clients, can be integrated into the retailer-specific mobile apps of fashion brand sites.
Below, we will highlight a variety of fashion-specific recommendation engines which claim they can be implemented or integrated specifically into a retailer’s website or mobile app.
Along with recommendations based on colors and patterns, we have also researched a number of recommendation engines that focus on size, weight and shape.
We have broken these applications up into the following two clusters:
- Personalized Style Recommendations: Based on factors that include color, color pattern or clothing shapes.
- Recommendations for Fitting: An engine that claims to suggests properly fitting clothing and apparel, as well as styles, after using computer vision or customer purchase data to detect a person’s height, weight and shape.
With this article, we aim to give readers insight on AI applications that claim to improve the recommendation process and trigger higher purchases.
Below is a table of quick data comparing the six applications we will discuss in this Head to Head. We’ve included information about funds raised, founding information, and how the applications process data.
Next, we will go through each of the above recommendation-based application to give readers a detailed description of how each claims to help increase sales revenue.
Personalized Style Recommendations
Syte.ai
- Total funds raised: $10.1 Million
- Year founded: 2015
- HQ location: Tel Aviv, Israel
- Number of employees: 33
-
Target user(s): Shoppers
-
Type(s) of data processed: Images
-
Estimated number of current users: Unspecified
Syte.ai is a visual search engine that claims to offer an image recognition tool that large fashion brands and publishers can integrate into their eCommerce sites. Shoppers can upload a photo of an outfit onto the application. Then Syte’s deep learning algorithm searches and presents images of a similar garment or accessory with different styles. These style differences could include a slightly different cut of the garment, as well as another pattern design or color.
Once the shopper selects the look they like best, the application will search for and offer similar items from the retailer’s inventory.
Syte claims to provides image recognition technology to retailers to allow their users to shop from any image. To start using the application, users go to a participating retailer’s site and upload a photo of apparel or an outfit they like using the integrated Syte tool. The application claims that it will find the similar results from within that retailer’s inventory using deep learning and image recognition technologies. Syte claims it breaks the outfit into components, allowing the user to shop the whole ensemble.
Once the user sees a look they like, they click on the chosen image and can proceed to buy similar items within that retailer’s inventory.
Syte claims that it offers another search capability where a user can take a photo of a garment and the feature will use deep learning algorithms to search and present a feed of public posts by influencers photographed wearing similar garments.
In the case study of Boohoo.com, the UK-based client wanted to enhance their users’ shopping experience while driving business growth. The challenge was to implement a more natural search method for the visually driven fashion segment instead of textual search. The case study claims that they also wanted a tool that would engage millennial and Gen Z shoppers.
In September of 2017, the company integrated Syte’s camera button visual search on their site, as well as the carousels of product suggestions called Shop Similar and Shop the Look. According to the case study, group revenue for the last four months of 2017 had nearly doubled compared to that month in the previous year, reaching over $308 million by 2018.
Since implementing this application, Boohoo’s Director of eCommerce Andrew Thomson, claims in the case study that his company achieved 100 percent increase in conversion rates from visitors using the ‘View Similar’ feature than those who do not. The case study also added that the average order value rose by 12 percent, while the average number of pages viewed during a session increased by 135 percent.
According to the case study Boboo has expanded its work with Syte and is in the process of adding the social media stream capability, which will allow users to see influencers wearing similar garments.
Aside from Boohoo, the company also lists Intu, Marks & Spencer and Kohl’s as clients.
Dr. Helge Voss, Co-founder and CTO, is a Doctor of Physics from Rheinische Friedrich-Wilhelms-Universitat Bonn, who specializes in machine learning, deep learning and image recognition. As a physicist at the Max Planck Institute for Nuclear Physics for 10 years, he was responsible for maintaining and operating the Toolkit for Multivariate Data Analysis machine learning package.
ViSenze
- Total funds raised: $14 Million
- Year founded: 2012
- HQ location: San Francisco, California
- Number of employees: 88
-
Target user(s): Shoppers
-
Type(s) of data processed: Images
-
Estimated number of current users: Unspecified
ViSenze’s Search by Image application also claims to use image recognition for various product categories such as clothing, jewelry and furniture. The mobile application finds products using an uploaded image or screenshot of an item from a physical store. This, according to the website, eliminates keyword searches that can sometimes take more time.
The 1-minute video explains how text search defined by the customer does not always accurately describe a product. For this reason, ViSenze developed an automated visual search application which it claims to be more accurate than text search.
ViSenze’s client Uniqlo wanted to provide online customers with a more convenient product search method that would also boost sales. The case study claimed that text-based search did not help customers find products that already existed in the catalog, resulting in lost revenue.
The retailer implemented Search by Image application. By introducing Search by Image, Uniqlo was also able to push their omni-channel approach further, as in-store shoppers could now easily search for same products online. The company reported that this resulted in higher conversion rates. In 2016, the company reported earning over $14 billion in revenue, however, no other figures or dates of implementation were released in the case study,.
Another visual search application offered by ViSense called Visually Similar Recommendations. It is distinguished from Search by Image in that it recommends similar items when the item searched for is out of stock from the retailer’s site.
ViSenze’s clients include Rakuten and Zalora.
Li GuangDa, CTO & Co-Founder, leads computer vision, machine learning and information retrieval at ViSense. He obtained his Ph.D. in Content Based Recognition Search from the National University of Singapore.
FindMine
- Total funds raised: $1.7 Million
- Year founded: 2014
- HQ location: New York, NY
- Number of employees: 23
-
Target user(s): Shoppers
-
Type(s) of data processed: Images, Text
-
Estimated number of current users: Unspecified
FindMine is a search engine that uses machine learning to offer product guidance to shoppers by composing a complete outfit based on a single product that the shopper has purchased.
Retailers can integrate the application into their eCommerce site, in email messages, in-store applications, sale’s associate’s tablets, or mobile sites.
To use the application, a customer enters a FindMine-equipped eCommerce site and types “floral dress” on the search bar. They can also upload an image of a floral dress. After initiating a search, the user will see a feed of matching or similar dresses. When a user clicks on a dress, the product details will be presented along with a “Complete the Look” bar at the bottom of the web page with product recommendations that might go well with the dress’ colors and other style patterns. The recommendations may include a pair of sandals, sunglasses or fashion earrings.
While the site searches for clothing items that may match with colors and patterns, FindMine also says its software also determines results by searching through photos of wardrobes from the retailer’s photo shoots or lookbooks. Among the retailers that use this application are John Varvatos and Milly.
In the 1-minute video below, Co-Founder and CEO Michelle Bacharach explains how AI works to find matching pants, shoes and jackets based on an image of a shirt that was uploaded onto the application.
The company claims that shoppers buy 170-percent more items when they find the right items to match a fashion product. The video also features Scott Lux, Vice President of Digital and Ecommerce at John Varvatos brand, who claims that during discussed a three-month pilot phase with FindMine.
According to the case study, the average order value rose by more than 70 percent, the conversion rate doubled, and revenue increased by six percent. During this three-month pilot, site time was also increased by 107 percent, the study notes.
Within the company, Marlies Santos Deas is the data scientist. Prior to FindMine, she spent six years at IBM. She obtained her Master’s degree in Computer Science from Columbia University where she focused in natural language processing.
Aside from its search recommendation engine, FindMine claims to follow through on its offerings by providing marketing support to clients in digital and in-store channels that still leverage the search engine and Complete the Look application.
In-store, FindMine provides retailers with digital displays, installed with the Complete the Look application, which can be used by sales associates to make recommendations to shoppers.
The company website also states that sales associates are given access to the FindMine analytics to suggest the best-selling looks across the store. The company also claims to provide retailers with “magic mirrors” or touchscreen displays on the retail shop’s floor for personalized recommendations, or a sales associate might have this information in a tablet, ready to share it with the customer.
For example, a smart fitting room can offer a touchscreen display (fitted with computer vision camera) that recognizes the dress a shopper is wearing. This activates a “complete the look” option on the screen. If the shopper presses “open,” the screen will display images of shoes, jewelry, a bag and other items that will complement the dress. These images are accessed from the retailer’s catalog, and are be curated to give the shopper information about the items such as sizes, colors, dimensions, price, etc.
To view more items, the shopper swipes right. Once the shopper selects their prefered items, the sales associate will collect these from inventory and deliver to the fitting room.
The shopper’s choice as well as looks provided by the retailer will train the machine learning algorithms will continue to make recommendations by analyze it by color, pattern, titles, description, price, gender, and more.
These parameters are supplied by the retailer, and FindMine uses its internal expertise to suggest additional ones. The final set of processing parameters has the retailer’s blessing. Image processing plays a key role in creating look books that retailers want to present. There’s a lot of artificial intelligence and big-data crunching that happens behind the scenes to deliver those options to you.
The company website reports that personalized emails are automatically triggered as a follow-up to a recent online purchase or when shoppers abandon their carts without finishing the purchase. The templated emails contain style guides that could potentially convince shoppers online shoppers return to the retail site and finish the purchase.
No case studies or demo videos could be found in regards to in-store sales or the “Complete the Look” digital fitting rooms.
Recommendation Engines for Fitting
3DLOOK
- Total funds raised: $1.4 Million
-
Year founded: 2016
-
HQ location: San Francisco, California
-
Number of employees: 32
-
Target user(s): Shoppers
-
Type(s) of data processed: Images
-
Estimated number of current users: Unspecified
Scanning Artificial Intelligence for Apparel (SAIA) Perfect Fit is an eCommerce widget developed by 3DLOOK that claims to recommend clothes based on the shoppers’ body size and shape. The application uses a its own computer-vision scanning technology trained to match 2D images with 3D models. According to the 3DLOOK website, the widget has 98-percent precision with a margin of error of one to two inches.
The application is integrated into a retailer’s eCommerce site or mobile app, where the shopper will be asked to upload two full-body photos with minimum resolution between 480х640 and 2000х4000 to the widget, then select their height and gender. SAIA claims it then measures the shopper’s body height and other statistics to determine clothing size. It then claims it can start suggesting clothes.
In this 3-minute video, 3DLOOK CEO and Co-founder Vadim Rogovskiy explains how the company is training neural networks to enable the technology to detect and measure human body.
He claims that algorithms are trained on a data set that contains 200,000 body images, to reach an accuracy of 98 percent.
The technology is now integrated into the Koviem website, which claims a 20 percent increase in revenue attributed to the 3DLook application. Fitting also claims that about 30 percent of Koviem’s customers have used the software since it was launched. Further details on numbers or time of the implementation were not included in this case study.
3DLook was also featured in the Accenture innovation center for retail, fashion and consumer goods industries in Milan lats September 2017. According to the company, SAIA is part of Accenture Customer Innovation Network fashion workshops.
CTO Ivan Makeev is the company’s research scientist who leads the advancement of algorithms, computer vision, deep learning, and other AI. He holds a Doctorate in Computational and Applied Mathematics from St. Petersburg State University.
True Fit
- Total funds raised: $112.4 Million
- Year founded: 2010
- HQ location: Boston, Massachusetts
- Number of employees: 51-100
-
Target user(s): Shoppers
-
Type(s) of data processed: Text, shopping transactions
-
Estimated number of current users: 55+ Million
Confidence Engine is part of a suite of “True Fit Genome” tools developed for retailers by True Fit. True Fit claims this machine-learning recommendation engine can give users size guidance and help them purchase clothes with the best fit from retail store clients.
When a customer starts browsing on one of True Fit’s retail client sites and clicks to expand a product, they will see a True Fit icon. When they click it, they will see a True Fit window open up which prompts them to make a True Fit size profile. From there, they can either add in detailed sizing information to create a profile, or generate one with recent purchase data that they may have with the retailer.
If the user shops on the retailer site they started on, or another retailer client’s site while logged into True Fit, they will be able to see a fit rating showing how well of a fit the clothing item might be, generated by data from their size profile, shopping history and similarly sized shopper reviews. The True Fit widget can also show the customer other clothing recommendations based on what it indicates might be the best fit.
According to the website, the application’s algorithms are informed by the user’s measurements and past purchases, as well as 600 million style attributes and 130 million consumer profiles to return the best fit rating (a 5-point scale of sizes) and size recommendation for a specific garment or shoe.
As the video below shows, shoppers begin using the application by first creating a profile that includes details such as height, weight, body shape, clothing size and style preferences.
The website also notes that shoppers need not create a profile in the retailer’s eCommerce site if they had previously made purchases from the retailer’s site. Once the profile is created, the application’s AI will access the database of connected retailers and recommend items appropriate to the shopper’s specifications.
ASICS was faced with the growing issue of fitting customers properly. The retailer implemented True Fit’s application across its digital commerce channels which, the case study reports, instantly gave customers a fit recommendation. The case study reports that ASICS achieved a more than 150-percent increase in conversions since implementing True Fit. The study, however, did not indicate the period of time this covered or further revenue numbers.
Aside from Asics, True Fit claims it has more than 200 retail clients worldwide including Kate Spade, Guess, Under Armor, Cole Haan and Saucony. In January, it reported surpassing 55 million users.
Christopher Moore is True Fit’s Chief Analytics Officer, and leads the modelling of algorithms. He obtained his Doctorate of Physics from the Massachusetts Institute of Technology.
Secret Sauce Partners’ Fit Predictor
- Total funds raised: Unspecified
-
Year founded: 2010
-
HQ location: San Francisco
-
Number of employees: 35
-
Target user(s): Shoppers
-
Type(s) of data processed: Text, purchase history
-
Estimated number of current users: 40 million
Fit Predictor, created by Secret Sauce Partners, is a body measurement application that uses customers’ purchase history to develop a fit profile which, the company claims grows with data as users continue to shop.
The company reports that the application is integrated into a retailer’s website and initially asks customers first answer a three-question survey about their general size before it can be used. Fit Predictor claims to combine this data and the customer’s purchase behaviour to continue refining his or her profile.
The widget will then show them clothing recommendations from the retailer that it match with their fitting requirements. They can also click into a clothing item they are interested in and see a prompt saying which size would be the best fitting based on a specific garmant’s details.
While we could not find a demo for Fit Predictor, the image below notes how the integration suggests which dress size will be the best fit for a specific product on a retailer site:
Source: Fit PredictorSimilarly to True Fit, customers without a profile in the retailer website can still be provided clothing recommendations based on their sizing with other brands, according to the company.
The company also claims that it has 40 million active users a month, 70 million people with fit profiles, and is making 320 size recommendations per second on average with product attributes from 7,000 supported brands.
A search of the company website and the internet did not reveal case studies, but the company lists Bloomingdale’s, Saks 5th Avenue, Barney’s New York and Nordstrom as among its clients.
Ioannis Kasampalidis, the company’s Chief Scientific Officer, earned his Doctorate in electrical engineering from the University of Washington. We could not find another member of leadership with a robust AI background.
Concluding Thoughts
While we have seen a strong growth in AI for marketing, cataloging and producing apparel, this research shows that AI recommendations are another commonality.
While some applications claim to recommend based on style, color or influencer photographs using computer vision. Others are using this technology to recommend based on size or fitting, similarly to an in-store human fitting room assistant.
These applications, which all seem to have high funding and marquis clients offer site integration such as widgets or a customizable feature that can be added to their eCommerce app. Many of these applications are also smaller features created by larger, higher-funded companies, in the case of True FIt’s Confidence Engine, Secret Sauce’s Fit Predictor and 3DLOOK’s SAIA Perfect Fit.
Companies behind these applications all also seem to claim that recommendations, such as those that complete a look or offer fitting suggestions, will boost a customer’s confidence when purchasing a product.
Many of these applications, such as Fit Predictor and Confidence Engine, claim they continue to monitor shoppers and grow their preference profiles as a person shops. However, these applications run on data from a pool of their marquis clients, rather than specific data from just one retailer site. Similarly shoppers interested in using these features are not just limited to accessing them on one retail site.
While a shopper might engage with these fit recommendation features on one retail site, which may lead to a purchase, these applications could also continue to track data and give similar recommendations when the customer purchases clothing from other retail clients who’ve added these recommendation engines.
Header image credit: People Magazine