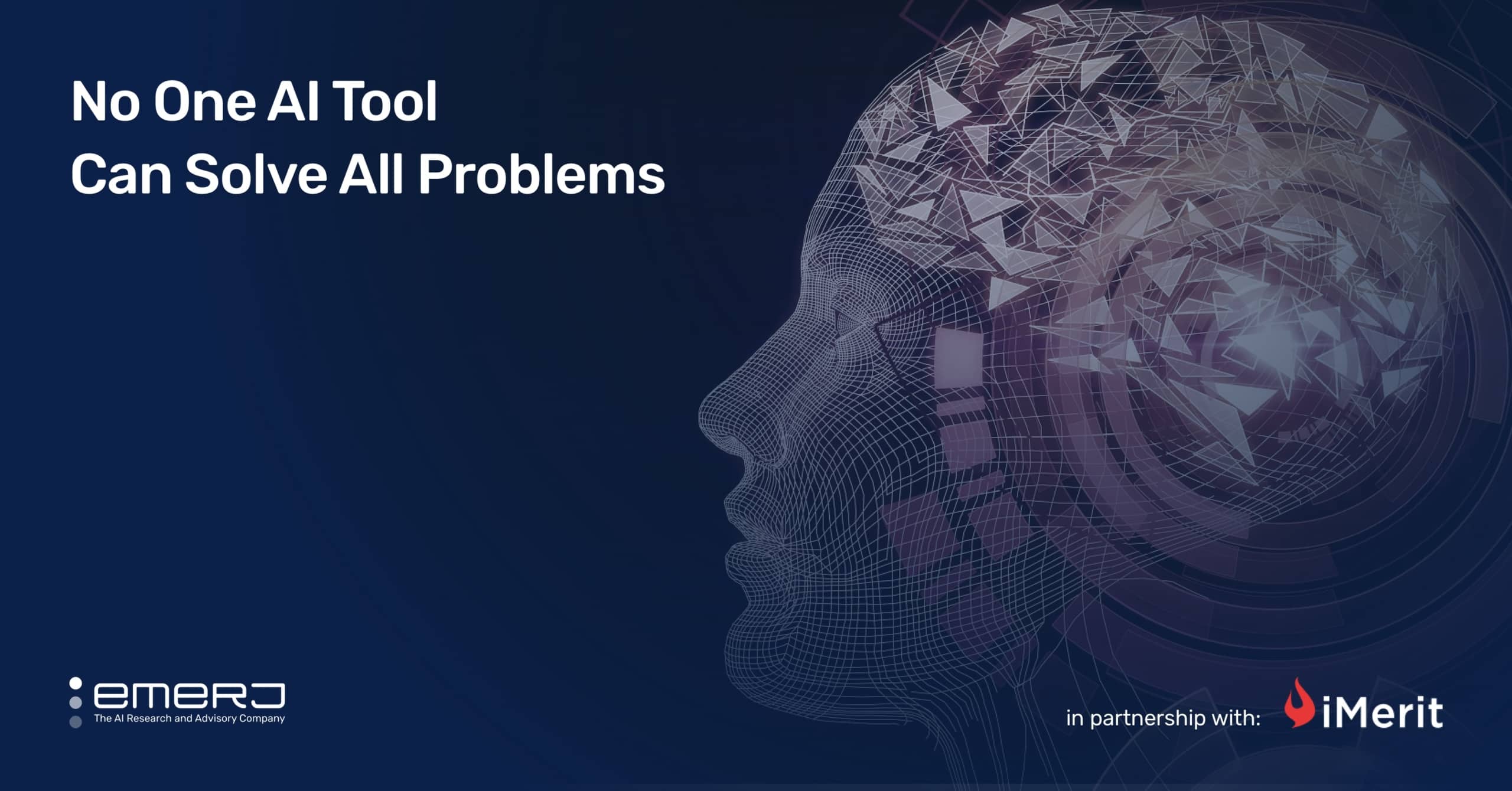
This article has been sponsored by iMerit Technology and was written, edited, and published in alignment with Emerj’s sponsored content guidelines.
When it comes to the realities of AI adoption and deployment, it’s often the service providers – executing the hands-on work within the enterprise – who have an in-depth understanding of the challenges that may arise.
In this episode, we explore what it looks like to define a business problem, determine the right tools, and bring a project to life from the perspective of a business leader at the juncture of data value and workflows. Our guest this week is Jeff Mills, he is the Chief Revenue Officer of iMerit Technology.
iMerit Technology is a firm that works in the data labeling and annotation realm with some of the world’s largest autonomous vehicle companies to help them improve their models for vision, LiDAR, and more. iMerit also works with many well-known enterprises in big tech and legacy enterprises with less AI transformation experience.
We cover two primary topics in this 30-minute interview:
- How to manage data for different types of AI projects
- Regulating human-in-the-loop intervention to ensure data quality
Listen to the full episode below:
Guest: Jeff Mills, Chief Revenue Officer at iMerit Technology
Expertise: Marketing, sales, and management.
Brief Recognition: Before joining iMerit Technology’s team in 2016, Jeff was the Global Vice President of Sales and Marketing for 1-Page which went public on the ASX. Earlier in his career, he held positions at Gengo, Criteo, Yahoo, Sojern, and SideStep (acquired by Kayak). He completed his university degrees at St.Mary’s College of California, Notre Dame de Namur University, and Stanford University Graduate School of Business.
Key Insights
- Two different kinds of AI projects – Depending on your company’s level of AI deployment and maturity, you will encounter vastly different areas of priority. Jeff highlights this, explaining that with companies that are more mature in terms of their AI deployment, their focus is mostly streamlining and validating their workflows. While companies just getting started with AI projects encounter a vastly different reality.
- Where humans in the loop fit into the workflow – Jeff explains that human involvement comes into play at different parts of the workflow, depending on whether or not the project is primarily employing a data-driven or self-learning approach. He explains that when a data-driven approach is being used, human intervention is very important at the beginning of the process, the least necessary in the middle, and slightly important at the end to verify that the quality meets project expectations.