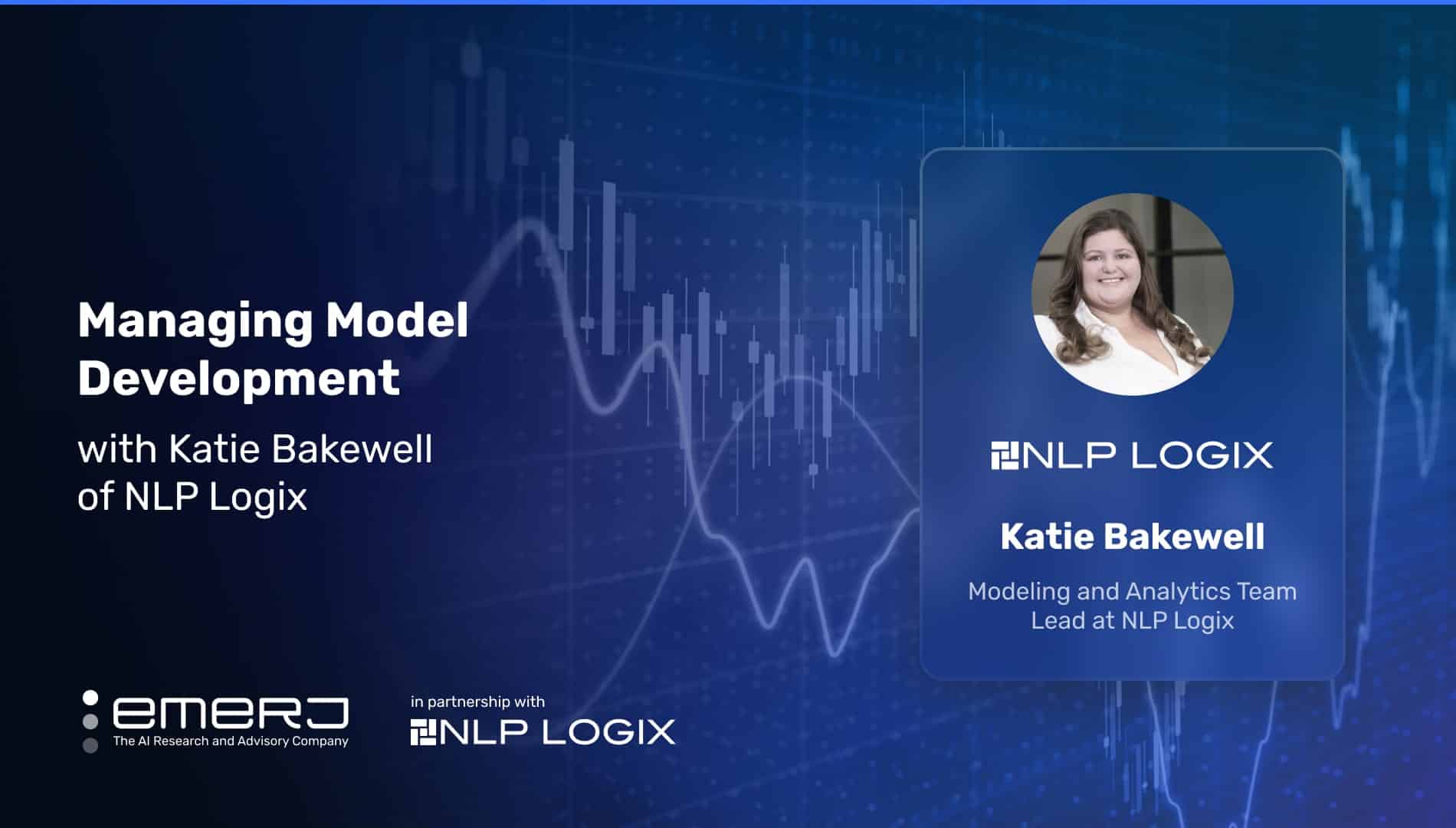
As a business practice, model development aims to create a dataset, tailored through machine learning, that can accurately predict outcomes or classify data based on input variables. By following a structured approach, developers can ensure that the model development process is efficient, effective, and reproducible.
Emerj Senior Editor Matthew DeMello recently spoke with Katie Bakewell, Modeling and Analytics Team Lead at NLP Logix, on the AI in Business podcast to discuss the importance of a structured approach to model development and the challenges in building bespoke models.
Throughout the program, Katie emphasizes that taking a step further and mapping model development after the software development lifecycle (SLDC) helps ensure that these systems are developed with the business’s core goals in mind and deliver information and insights directly tied to return on investment.
In the following analysis of their conversation, we examine two key insights:
- The fundamentals of a structured approach to model development: How to build models in line with the SLDC by identifying the correct initial project through a viable proof of concept (POC), confirming the signal, and then moving the model into production.
- Closing the data training gap: How transformer models and a discerning view of metrics can better identify disparities between data used to train models and the data they process in practice.
Listen to the full episode below:
Guest: Katie Bakewell, Modeling and Analytics Team Lead, NLP Logix
Expertise: Statistical Analysis, Machine Learning, and Business Intelligence
Brief Recognition: Katie has spent the last nine years at NLP Logix, first as a data scientist from 2013 to 2020 before being promoted to her current position as Modeling and Analytics Team Lead. In 2017, she earned her master’s degree in Mathematical Science from the University of North Florida.
Structured Approach to Model Development
When developing machine learning models, Katie tells Emerj that many business leaders make the mistake of putting models into production before they’ve verified signals that will help their model support their core business goals. Instead, she suggests following a structured approach, similar to the software development life cycle (SLDC), which involves starting from the beginning and designing a plan to get into production.
She acknowledges that developing machine learning models is more complex than software development because many unknowns exist at the beginning of the adoption process, such as whether the data being cultivated will fit the final solution.
Bakewell also suggests that starting with a proof of concept or a time box for R&D can help. By starting with a smaller project, the team can determine what they are trying to solve and whether the data and signals are available to solve the problem.
Katie further discusses the importance of defining metrics and expectations in the projects, noting it is crucial to clearly understand the targeted metrics before starting the development process.
She cautions that there may only sometimes be a signal to target the desired metrics, and it’s essential to allow for checkpoints early in the process where the project can be evaluated and adjusted as needed. We will discuss more on how to think of long-term metrics in the following key insight summary.
However, until five years ago, it was common to sit down and add slight changes to models over a weekend, tweaking different parameters until it met the desired accuracy – usually around an 80 percent accuracy rate.
Today, these changes can’t be considered so casually or siloed – AI models are now regarded as business-critical tools where even slight changes can potentially affect the model’s future efficacy and lead subsequent business-critical operations astray. For teams to balance an increasingly intense model development process, Katie advocates breaking it down into smaller phases resembling the SLDC.
To do so, Katie suggests that – before beginning work in building the model officially – business leaders should select an initial project that involves:
- Research and development
- A viable POC
- Dataset analysis
Throughout her appearance on the podcast, Katie emphasizes that the process for model development at NLP Logix starts with a viable POC. From there, adoption teams conduct an initial analysis on a sample subset of data and ensure the signal works before moving on to the complete data set.
Once the signal is confirmed, the model can be built with additional design work. From there, the model can finally be moved into production and supported through general maintenance practices.
She believes this structured approach allows business leaders to understand the process better and evaluate the project’s progress at each phase:
“It gives business leaders the ability to step in and put a toe in the water, especially if it’s that business-critical piece, where you can say, ‘Let’s see what this will look like. And let’s step lightly into this process.’ Rather than just trying to go in swinging, it allows you to build a model that will perform how you expect it. You won’t have that shock where it gets out of the lab, and suddenly your business-critical process isn’t working anymore.”
– NLP Logix Modeling and Analytics Team Lead Katie Bakewell
Closing the Data Training Gap
In discussing how NLP Logix uses metrics to monitor and understand changes in machine learning models over time, Katie cites a specific use case from the debt collection industry.
As a general metric, she notes there are limitations in using credit scores to predict what borrowers will pay their debts on time reliably. While she feels credit scores help measure who can pay their debts but do not consider external factors that may impact a consumer’s ability to pay.
To compensate, she suggests using demographic information such as the consumer’s address, estimated income, and estimated housing payment to estimate their disposable income, a more accurate indicator of their ability to pay debts.
Relating the example to the larger task of model development across industries, Katie insists business leaders can better anticipate when their model is going off track and identify the features that need to be retrained by:
- Taking a skeptical view of traditional metrics (like credit scores)
- Tracking all changes to metrics and model variables from the beginning of development
In taking a ‘skeptical view,’ business leaders should constantly ask themselves whether the metrics their model is built on deliver information that speaks specifically to the factors driving their business. If credit scores can’t tell you who will pay bills on time, you need to find the metric that will – and that may require getting creative.
As Katie puts it, “These are using early warning signs like canaries in a coal mine to detect potential issues and get back on the right course in a much faster way than you traditionally would.”
In her debit collection example, she notes that these insights can be used to develop dashboards and other ways of tracking insights and changes at the core of AI-driven business value. She explicitly mentions that if the data that the model was trained on differs from the data it currently works with, it will cause the model not to function correctly.
She concludes the conversation by discussing how model development may look in the future – emphasizing that model development has changed with the advent of transformer models.
She notes that only a few years ago, business leaders needed to have years of data to build a bespoke model. Now with the transformer models, a large language model is already available without training or coding required that only needs fine-tuning with continued use. For that reason, she believes we will see more sectors seeking transformer models in the future rather than building them from scratch.