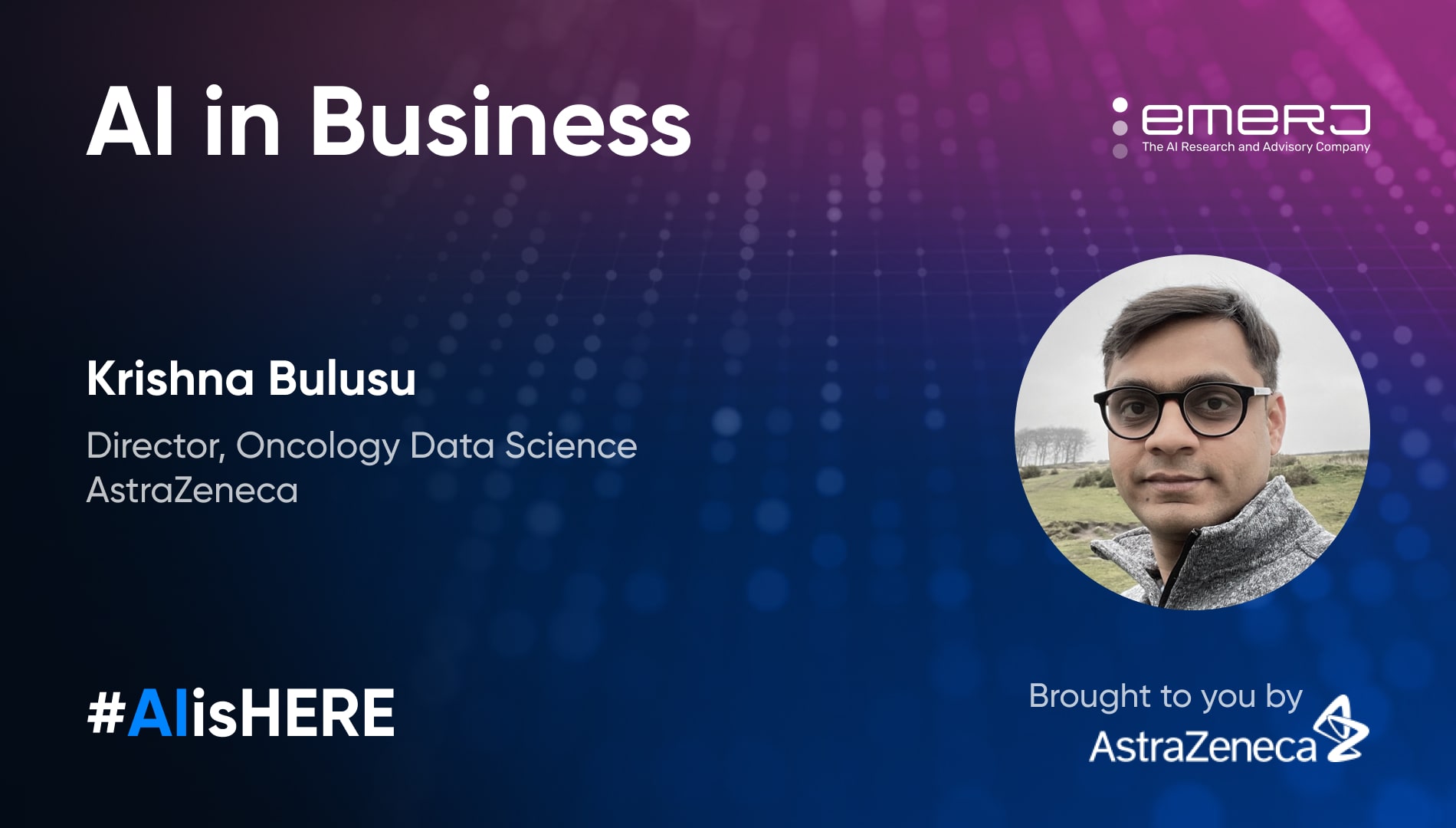
Knowledge graphs have grown into a major trend in life sciences since the term was introduced by Google in 2012. However, despite this recent uptick in use, they significantly impact the space. Knowledge graphs enable several critical functions within life sciences, including drug development, literature review, and regulatory reporting.
Due to the overwhelming amount of information researchers in the biomedical space work with, knowledge graphs make it easier to identify relevant insights much more quickly.
Within artificial intelligence, knowledge graphs are known as semantic networks. Knowledge graphs can complement other machine-learning techniques. Even though they’re relatively new to the biomedical space, knowledge graphs have essential applications.
One example application is recommending the next new drug target and the relevant new patient subpopulation. Knowledge graphs can also be used to guide clinical decision-making and can further the field of personalized medicine.
Emerj recently sat down with Dr. Krishna Bulusu, Director of Computational Oncology of AstraZeneca on the ‘AI in Business’ podcast, where we explore real-world applications of knowledge graphs in life sciences, including minimum information standards for data and how knowledge graphs can integrate multiomics data.
This article will focus on three key takeaways from our interview with Krishna Bulusu of AstraZeneca:
- Drug target discovery: Using knowledge graphs to identify which of thousands of drug targets is worth pursuing.
- Measuring a given endpoint with the FAIR method: Applying knowledge graphs to multiomics using a four-point criteria.
- Identifying the biology driving a specific phenotype: Using knowledge graphs to determine why particular phenotypes resist a specific drug.
Listen to the full episode below:
Guest: Krishna Bulusu, Director of Computational Oncology, AstraZeneca
Expertise: cancer translational research, drug discovery
Brief Recognition: Dr. Krishna Bulusu holds a Ph.D. from the Institute for Cancer Research and completed post-doctoral research at the University of Edinburgh.
Drug Target Discovery
Knowledge graphs bring very diverse pieces of information together. In an interview with Emerj, Bulusu explained how a story can be built around a drug discovery journey within a particular knowledge graph. The breadth of information could show:
- A gene communicating with a cell line
- The cell line communicating with a disease
- That disease that connects to a patient
- The patient connecting to a drug
- The drug connecting to a side effect
According to Bulusu, knowledge graphs could fit at every stage of the drug discovery process, from identifying the next drug target, finding the suitable molecule to be used and turning that into a drug, marketing the end result, and putting the end result toward patient benefit.
“Right at the start of the journey, which is the target discovery piece, the ontologies and taxonomy which the graph needs to integrate goes across a piece of biology and chemistry and pharmacology and disease ontologies. So – a huge complex space is coming into a single platform.”
– Director of Computational Oncology at AstraZeneca, Dr. Krishna Bulusu
Identifying whether or not a knowledge graph is the right tool for the job is paramount. AstraZeneca sometimes gets requests which don’t end up needing a knowledge graph. AstraZeneca assesses the value proposition to determine if a knowledge graph is required.
There are two places where the value proposition for AstraZeneca lies:
- Can they identify the same solution in a much faster way? Perhaps they already have an automated system which already captures this prior knowledge.
- Can they identify those hypotheses which they would never otherwise identify if they were using a manual curation process?
When faced with more than 20,000 potential drug targets for a particular disease, knowledge graphs can identify which goals are worth focusing on and can be turned into a possible treatment.
Knowledge graphs can cut through existing research exceptionally well. Bulusu detailed a possible scenario where knowledge graphs could help. If a Ph.D. student interested in conducting an experiment approached him, Bulusu and his team could readily identify relevant research opportunities in the many thousands of existing research papers. His team will answer numerous questions, such as:
- How do I know which opportunities are the most relevant?
- How do I know which opportunities have the most evidence?
- Which opportunities are even actionable in the lab?
To address these concerns. Bulusu and his team build a snapshot of a knowledge graph called a subgraph that is very customized to the particular client problem.
Measuring a Given Endpoint with the FAIR Method
Bulusu explained the FAIR method and how that guides knowledge graph creation. The FAIR is based on an acronym that proceeds as follows:
- Findable: Every piece of data generated should be findable by others.
- Accessible: The data should be available to others.
- Interoperable: The data should have the appropriate cross-references to be interoperable.
- Reusable: Ability to use the data toward a different question than for what the data was initially generated.
Bulusu discussed several challenges that practitioners have in the space. He emphasized how biology is complex in the way it is represented. For example, there can be five different ways to refer to the same gene, and all five are true. He contrasted this to chemistry and mathematics, which follow principles and have a well-defined nomenclature.
Bulusu explained that knowledge graphs are great at building layers and the relationships between layers, and he gave an example of many different layers that might need to come together for a given patient, including:
- What is their lifestyle?
- What drugs has the patient been on in the past?
- What treatments has the patient had, and what was their response?
- What about the patient’s genomics?
- Does the patient carry a specific mutation?
- Has the patient responded to some other treatment in the past but now stopped responding?
The relationships between entities are what define a knowledge graph. Bulusu gave listeners a straightforward explanation of knowledge graphs, “A knowledge graph in a very simple sense is a collection of data and the relationships between individual data points, which can then help us make non-intuitive connections.”
A 2014 study titled Knowledge boosting: a graph-based integration approach with multi-omics data and genomic Knowledge for cancer clinical outcome prediction concluded that integrating multi-omics data and genomic Knowledge resulted in higher performance in clinical outcome prediction and higher stability.
Identifying the Biology Driving a Specific Phenotype
Bulusu discussed many challenges that practitioners have in the space. He emphasized how biology is complex in the way it is represented. For example, there can be five different ways to refer to the same gene, and all five are true. He contrasted this to chemistry and mathematics, which follow principles and have a well-defined nomenclature.
He explained that because there are 200 different cancer types, it can be challenging to categorize a patient population based on their cancer they have, “It’s a whole hierarchy that needs to come together, and the knowledge graph needs to be robust enough to capture this hierarchy.”
Bulusu mentioned AstraZeneca’s internal knowledge as an organization and explained that internal Knowledge can be used: “The knowledge graph is a perfect place to combine the world’s knowledge with our internal knowledge and run some practical AI algorithms on top of it.”
Bulusu went on to discuss the concept of minimum information standards. He touched upon questions such as:
- What is the level of information and annotation of the metadata that will get you started to run an analysis?
- What is the minimum information standard that any piece of data needs to adhere to before it can be used?
Bulusu and his team realized there isn’t one answer to this question; it must be context specific. One knowledge graph might be clean and decipherable enough concerning a particular question but might not be for others.
Any recommendations that result from knowledge graphs do need to be validated by experts through a validation study. Bulusu elaborated:
“When you use AI to come up with a recommendation that immediately impacts a human being -a patient’s life- it will obviously need to go through certain regulations and certain rules and certain guidelines and principles and ethics.”
– Director of Computational Oncology at AstraZeneca, Dr. Krishna Bulusu
He touched upon how that might look different depending on the organization, country, or continent. “So when it comes to the EU, for example, we talk about GDPR, what level of data you can share, what you can use the data for, how much is the individual aware of what their data is being used for, and when it comes to AI and ML as we all know, more data is better to come up with accurate predictions.”