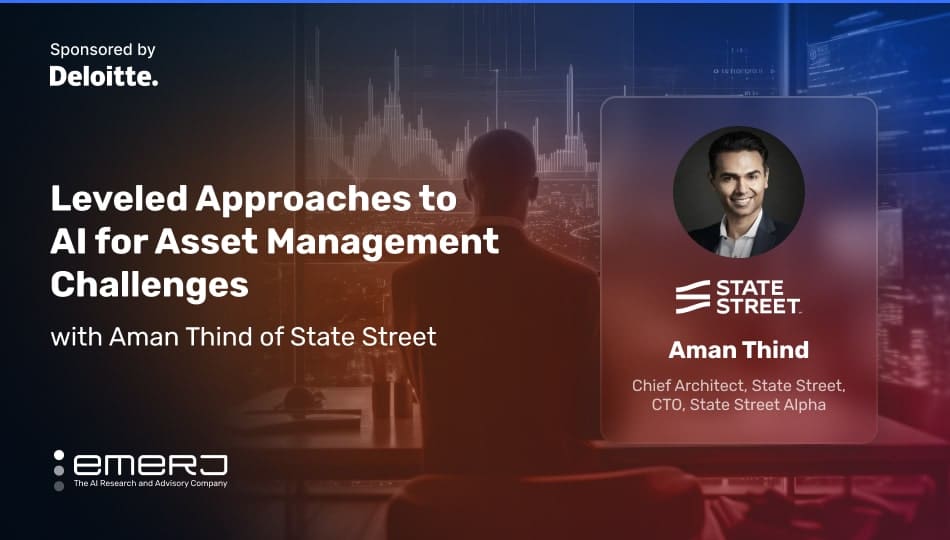
The asset management industry is going through several challenges, like the prolonged low-interest rate environment, dwindling margins, increased cost pressures, and squeezed profitability. Meanwhile, exponential growth in data volumes has overwhelmed legacy data management systems and analytical tools, making it tiring to process and extract valuable insights from the deluge of information.
Several AI techniques like artificial neural networks (ANNs), cluster analysis, decision trees, and genetic algorithms, when used in asset management for tasks such as asset allocation, portfolio optimization, risk management, and trading strategies can conduct insightful financial analysis faster and more efficiently than human beings.
Emerj Senior Editor Matthew DeMello recently spoke with Aman Thind from State Street on the ‘AI in Business’ podcast to discuss how AI applications can help make better decisions at asset management firms and how they can innovate with generative AI.
In the following analysis of their conversation, we examine two key insights:
- Leveraging AI for faster, higher quality decisions: Automating data aggregation and analysis to cut processing times from weeks to minutes, empowering senior staff to make quicker and more informed decisions.
- Unlocking unforeseen insights with generative AI (GenAI): Using GenAI’s unique ability to uncover correlations and offer unexpected insights, unlike traditional AI, to streamline data extraction, enhancing efficiency and accuracy.
Listen to the full episode below:
Guest: Aman Thind, Chief Architect, State Street
Expertise: Artificial Intelligence, Crypto, Data strategies
Brief recognition: Aman is the chief technology officer of State Street Digital Assets, a division dedicated to Crypto Assets and Web 3.0 technologies. Before joining State Street, Aman was co-founder and CTO of BestXSM, a fintech start-up focused on transaction cost analysis. Aman graduated from NIT Kurukshetra with an honors degree in computer science and engineering.
Leveraging AI for Quicker and Better Decisions
Aman opens the podcast by talking about the significant transformation underway in the asset management industry, where companies are increasingly focusing on data management.
The shift, he says, is driven by the realization that data is a crucial indicator of future success. Historical trading data and market sentiment analysis have shown that effective use of data can lead to better decision-making and improved outcomes. As a result, asset management firms are striving to harness the power of data to stay competitive and drive value.
However, Aman points out that there is a significant challenge in this transformation. He uses the analogy of needing to buy bread but growing corn. The complexity lies in figuring out the appropriate models to use, identifying relevant data domains, and ensuring comprehensive access to market data. Integrating all this data into cloud platforms and tying it together to generate value is a daunting task.
He notes that this is a widespread and persistent issue within the industry. Through his conversations with clients, he has observed that asset management companies consistently face these challenges. Some firms, like State Street with its State Street Alpha offering, are making efforts to address these issues, but the journey is far- developing a robust data strategy is just the initial step.
Even with a sound data strategy, Aman highlights that the next big challenge is extracting value from the data. It’s not enough to store data in modern platforms like Databricks or Snowflake.
Companies need to determine how to use this data effectively to enhance their trading patterns and workflows. The goal is to complete the loop by taking the insights gained from the data and applying them back into their operations.
He argues that every company, regardless of its primary business, must incorporate technology into its operations to survive and succeed. To illustrate this point, Aman refers to the significant changes in the composition of the S&P 500 index over two decades.
“If you look at the S&P 500, and if you compare the listings from the year 2000 to 2020, just in these two decades, 52% of the companies from the year 2000 no longer exist. They have been replaced by companies that have harnessed the cloud for scalability, internet accessibility, and mobile in order to improve their overall reach. And it is becoming apparent that no matter what industry you are in, you need to harness the capabilities of everything that is around you in terms of technology in order to be able to survive and thrive.”
– Aman Thind, Chief Architect at State Street
Aman then discusses the potential of AI in financial services through the lens of asset management, particularly in efficiency and decision-making:
Enhancing efficiency: The most basic and essential use case for AI is improving efficiency and operating margins. In the financial services sector, risk and control processes often involve multiple layers of checks, sometimes up to 12. By integrating AI into these processes, companies can streamline operations. AI can serve as a reliable “eye,” handling the initial data aggregation and analysis, while humans focus on assessing the outcomes.
Impact on decision-making: Many industries rely on junior employees to gather and assemble data over weeks, which senior staff then review to make decisions. This process is time-consuming and inefficient. If AI could take on the role of junior employees, the time needed for data aggregation would be reduced from weeks to minutes. With AI handling data aggregation swiftly, companies gain valuable time that can be used to either make more frequent decisions or improve the quality of decisions.
Monetizing AI capabilities: Raw data alone has limited value; its worth depends on the buyer’s ability to interpret and use it. Aman uses the analogy of selling oranges versus selling orange juice to illustrate this point. Instead of merely providing raw data, companies should use AI to generate actionable insights and offer these insights to clients. This approach not only adds value for the clients but also enhances the company’s profitability.
Aman further explains how AI can enhance efficiency in the financial services industry through automation and anomaly detection. These automations can involve complex tasks such as anomaly detection. In the financial services sector, there are numerous controls in place to monitor activities like NAV (Net Asset Value) calculations, AML (Anti-Money Laundering), and KYC (Know Your Customer) practices.
Managing these controls often involves sifting through thousands of lines of data, which is time-consuming and labor-intensive:
“If you have been able to train your AI model based on previous alerts, the actions that were taken on top of that, as such that it eventually starts sifting them through and giving you higher quality data, which is directly actionable for you by also simultaneously giving you memory of how those actions were taken last time so that that becomes the press of a button. Then, the thousands of people who are currently employed in these jobs wouldn’t be replaced with those who provide higher value jobs, which is to actually take those alerts and then subsequently act on them, thereby improving the overall efficiency of how you work with these clients. Also, improving the overall throughput will help you do more business.”
– Aman Thind, Chief Architect at State Street
Unlocking Unforeseen Insights with GenAI
Aman then turns to the conversation to focus on how GenAI can help the industry. He explains that unlike conventional AI, which predicts outcomes based on predefined data and specific queries, gen AI has emergent properties, which means that it can identify correlations and provide insights that users may not have anticipated or even known to look for.
GenAI stands apart from traditional AI methods, such as machine learning, which require structured training processes. For example, traditional document extraction involves tagging documents and training models to recognize and extract specific data. GenAI simplifies this process by understanding and querying data more intuitively. Instead of specifying exact locations or keywords, users can ask questions directly, and the AI can extract relevant information based on its training.
Aman also explains how GenAI streamlines the process of retrieving specific information, such as the return on investment from a fund in a historical context. Instead of manually searching through documents and tables or identifying keywords, users can input their query directly, and the AI will extract the relevant information based on its pre-training.
An automated approach significantly reduces the time and effort required for training and improves overall accuracy. Furthermore, through reinforcement learning and human feedback, the AI-enhanced system can continuously refine its capabilities, becoming even more accurate over time. The new iterative process allows for ongoing improvement without the need to start from scratch with each query or task.
“GenAI is like a child; it learns something new every single day when it goes to school. But what this also means is you can ask him a question today, and you can ask another question six months later, and the answers could be different, but I think that is natural because Now it has more information to give you a different answer. So as long as you have a very, very tight feedback loop, such that it continues to give you high-quality responses based on your ability to train it back by grading the output that it is generating, it can be quite revolutionary for almost every business I can think of.”
–Aman Thind, Chief Architect at State Street
Aman emphasizes the transformative nature of AI, likening the current moment to the invention of the internet or mobile technology. He underscores the critical importance of adaptability for long-term business success. He suggests that companies that have thrived over decades are those that have recognized and embraced transformative technological shifts. These companies not only adapted to change but also played a leading role in shaping it to their advantage.
The speaker stresses the need for corporate leadership, including boards, CEOs, and executive committees, to recognize the significance of the current technological landscape. However, he cautions against hasty adoption without considering potential ethical and responsibility concerns. While urging swift action, he emphasizes the importance of proceeding cautiously to avoid overlooking ethical implications or regulatory obligations.