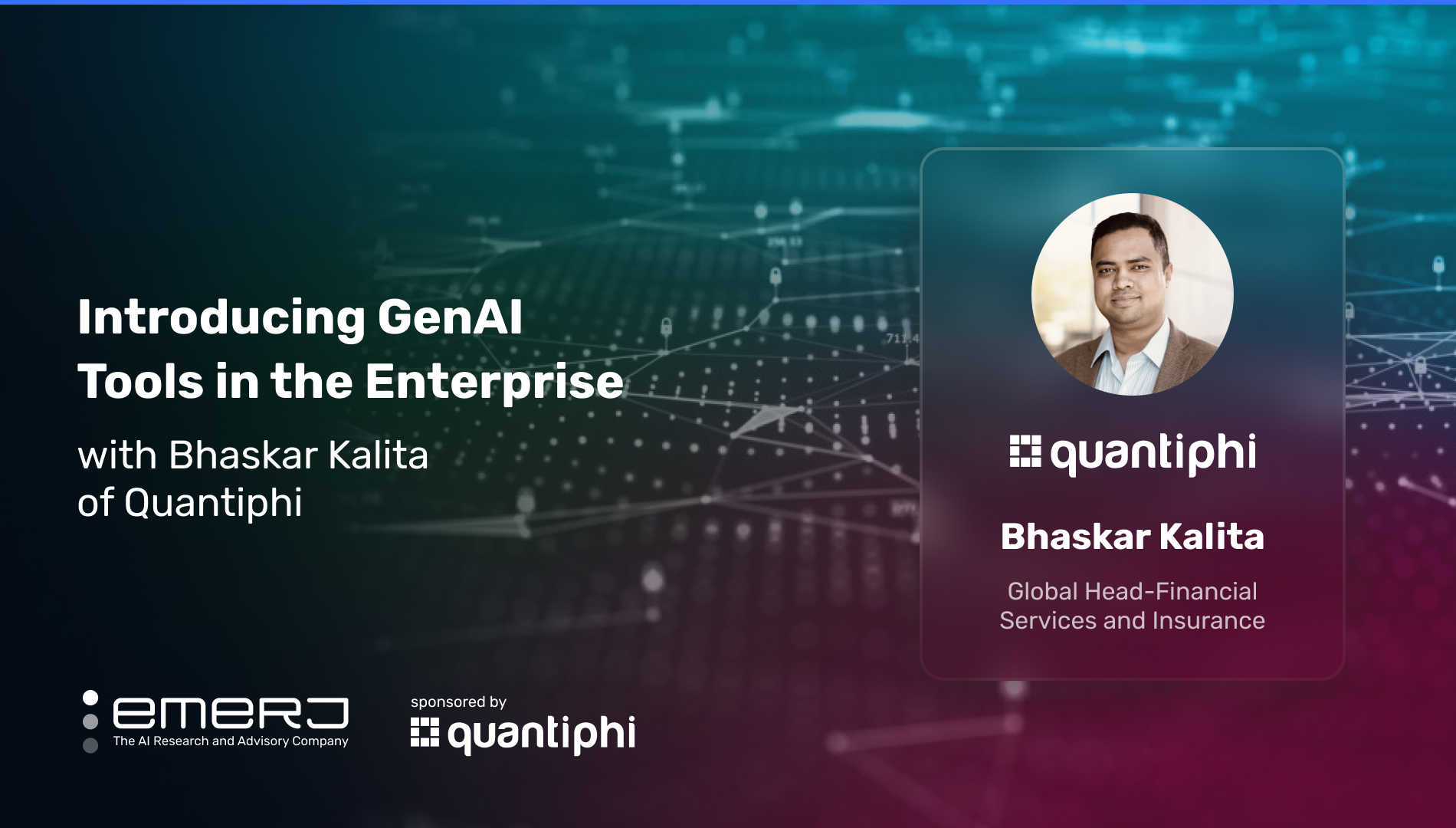
The introduction of GenAI tools in the enterprise signifies a significant shift in how businesses operate in the current technological landscape. These tools enhance various aspects of daily operations, from data analysis to decision-making processes.
Automated workflows and intelligent data analytics are set to simplify tasks and provide valuable insights, allowing organizations to adapt and thrive in a changing business landscape. In turn, the resulting processes enable previously impossible enterprise capabilities, such as allowing drug researchers to explore the three dimensional space of protein structures virtually.
As businesses increasingly adopt these tools, they position themselves to stay competitive and agile in an environment where human and AI fusion is becoming a standard practice, ensuring a more seamless and innovative future for enterprise operations.
Emerj CEO and Head of Research Daniel Faggella recently spoke with Bhaskar Kalita (who prefers to go by the nickname “BK”), Global Head of Financial Services and Insurance, Quantiphi, on the AI in Business podcast to discuss the transformative power of generative AI and the critical factors and considerations associated with implementing generative AI.
The following analysis examines three critical insights from their conversation:
- Using generative AI To reduce turn around time (TAT): Leveraging generative AI to enhance efficiency and potentially reduce the time for a specific process by 30%.
- Experimenting with smaller pilot generative AI use cases: The value of beginning with smaller pilot projects to understand its practical applications, as waiting may lead to future challenges and a need for rapid catch-up.
- Critical factors associated with implementing gen AI: Understanding the importance of technology infrastructure, skill development and organizational change management when implementing generative AI use cases.
Listen to the full episode below:
Guest: Bhaskar Kalita, Global Head-Financial Services and Insurance, Quantiphi
Expertise: Applied AI, Machine Learning and Business Development
Brief Recognition: BK is a seasoned executive with experience in consulting, business development, client relationship management and account portfolio management. He holds a Bachelor’s Degree from the National Institute of Technology (NIT) in Kurukshetra, India and an MBA from the Booth School of Business, University of Chicago.
Using Generative AI To Reduce TAT
BK begins by explaining how specifically conversational AI has evolved in the banking sector. He starts with a simple example to illustrate this evolution: Traditionally, when customers needed assistance with banking tasks, they interacted with an interactive voice response (IVR) system, where they had to navigate through a menu by pressing numbers.
Then came conversational AI, which allowed users to have more natural and empathetic interactions, making it easier to seek information and assistance. However, the limitation was that users still had to execute tasks manually, such as opening a bank account after receiving instructions from the AI. Here, BK introduces a transformational opportunity of generative AI, which is not explicitly defined in the text.
Today with generative AI, conversational AI can automatically handle the entire process following a user’s conversation. That means that users no longer need to perform the subsequent steps after interacting with the AI-enhanced systems, such as filling out forms or submitting information.
BK further highlights the remarkable impact of this technology. Regardless of a user’s level of education or familiarity with banking processes, they can engage in a conversation with the AI in their language and have the AI take care of multiple steps in the process automatically. He expresses optimism in how generative AI stands to extend access to various services globally and significantly enhance efficiency, reducing the time required for specific processes from days to potentially 30% of the current duration.
BK goes on to point out several concerns and apprehensions related to the introduction of generative AI, particularly in the context of data security and job displacement:
- Data Security: Many people, especially in the financial services sector, are worried about protecting their data when using AI technology. He suggests these concerns may subside as worthwhile guardrails and security measures are developed and implemented.
- Job Security: BK points out that as AI and automation technologies like generative AI advance, specific job roles may become redundant, leading to job displacement. He refers to studies that have indicated the potential replacement of millions of jobs and the emergence of new job profiles in the coming years.
- Biases in AI Results: He also raises concerns about inherent biases in AI-generated results. He questions whether AI, including generative AI, might produce biased responses or outcomes. It is particularly concerning when AI is used in critical functions like credit risk assessment or customer segmentation, where fairness and unbiased decision-making are essential.
He then introduces two promising generative AI use cases emerging across industries:
- Customer Engagement and Marketing: Using AI to analyze customer behavior and historical trends to predict their subsequent actions or preferences. Subsequently, personalized and contextually relevant marketing campaigns are created and sent to individual customers based on their anticipated needs and preferences.
- Claims Processing and Document Analysis: Using an array of capabilities from optical character recognition to machine learning – often referred to as ‘Document AI’ – to streamline core business processes like claims processing. In their typical workflows, claim specialists receive multitudinous documents related to claims. Document AI systems can scan, analyze and extract relevant information from these documents. The extracted metadata is then used to generate qualitative responses or assessments for claims adjusters, expediting the process and providing structured data for informed decision-making.
Experimenting With Smaller Pilot Generative AI Use Cases
BK tells the podcast audience he also believes that generative AI represents a unique and once-in-a-lifetime opportunity for organizations to rethink and redesign their existing processes. He emphasizes that these developments will force decision-makers to reassess their operations and consider alternative approaches to their processes.
In the past, many organizations may have implemented technological changes or improvements in isolated, fragmented ways, yet to address the broader context – as BK explains. However, in the current technological landscape, the capabilities provided by generative AI and similar technologies will prompt a shift in mindset.
He emphasizes that the institutions must embark on their generative AI journey by piloting minor use cases and gaining hands-on experience with the technology. This process allows them to understand AI’s practical applications, advantages and potential challenges in a controlled and manageable environment:
“All financial institutions need to start doing experiments; to start putting certain smaller use cases into pilot phase, to understand or get a better know-how of the tech. The reason I say this is because, again, this tech is also going to evolve. We had Open AI come up, you see all the advancements that Meta is doing and you have the entire stack from Nvidia evolving every other day. So you have powerful LLMs coming out every week, which means we will be in this day-one mode for a while until these developments settle down. It’s important that everybody gets started now because when this evolution advances, you will not feel left behind.”
– Bhaskar “BK” Kalita, Global Head of Financial Services and Insurance at Quantiphi
He says so because the “wait and watch” attitude could have detrimental consequences in the long run. He cautions that by choosing to wait, organizations may need to catch up when they eventually decide to utilize generative AI. The inherent delay might create a sense of urgency and a need to catch up rapidly.
BK also expects that this renewed focus on process redesign and innovation will significantly change how various sectors operate – such as banking, wealth management and insurance operate.
Critical Factors Associated With Implementing Generative AI
He outlines several critical factors and considerations associated with implementing generative AI use cases, emphasizing that it takes time for these elements to be established and refined. These factors include:
- Technology Infrastructure: BK highlights the importance of a robust technology infrastructure to support generative AI use cases. Such infrastructure encompasses security measures, responsible AI guidelines and the necessary hardware and software for tasks such as inferencing (using AI models to make predictions or decisions) and training or fine-tuning AI models. Having a foundation of these fully developed capabilities is critical for the successful deployment of AI applications.
- AI Education and Upskilling: While BK acknowledges Quantiphi’s involvement in supporting customers to get started with generative AI, he also stresses the significance of organizations building their own “muscle” or in-house AI expertise.
- Organizational Change Management: As organizations implement generative AI use cases, they should also focus on managing the changes that arise from adopting this technology. It includes addressing any shifts in roles, processes and procedures, ensuring that employees are prepared for and receptive to the changes.
Lastly, BK advises how stakeholders should approach understanding and adopting this technology. He offers two suggestions for helping executive leaders become intimately acquainted with precisely what generative AI capabilities can and can’t do:
- Attend generative AI workshops to actively witness the results that these technologies can achieve. The hands-on exposure will help stakeholders recognize how the technology can be applied in the context of their organizations.
- Create a blueprint outlining the areas within their ecosystem where generative AI can significantly impact. The process involves identifying a list of potential use cases. Stakeholders should then prioritize these use cases based on their potential impact and begin taking steps to implement and benefit from them.