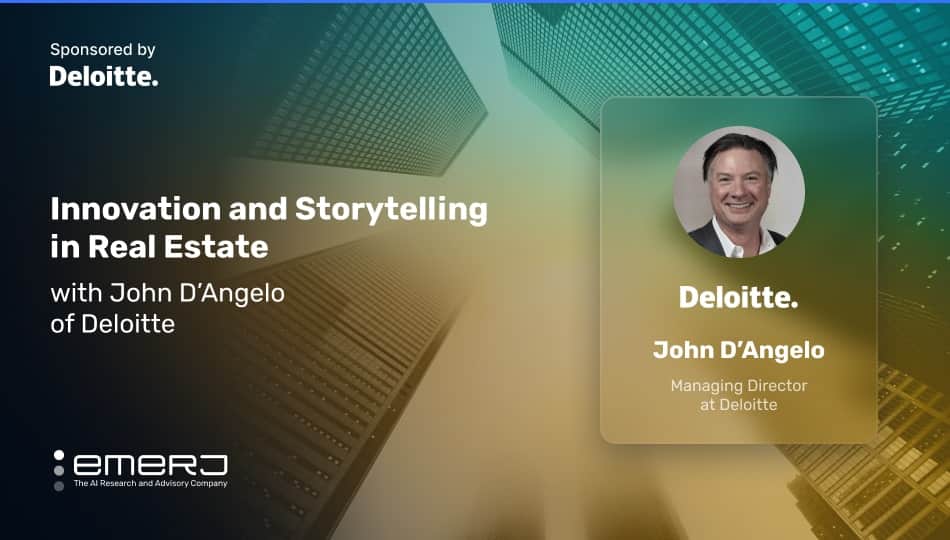
This interview analysis is sponsored by Deloitte and was written, edited, and published in alignment with our Emerj sponsored content guidelines. Learn more about our thought leadership and content creation services on our Emerj Media Services page.
While AI has reached an inflection point in sectors throughout the financial services space, real estate is considered still in its nascent stage of adoption. In other words, real estate entities that deploy machine learning or AI techniques still tend to be rare and sometimes hard to find, as described in the abstract of a recent MIT study on the subject.
In addition to operational improvements, AI can lead to new opportunities and efficiencies in real estate workflows hitherto unrealized, such as reducing carbon emissions through sensorial data collection. As described in a Bloomberg profile of CBRE Chief Digital and Technology Officer Sandeep Davé, implementing these changes has become an industry imperative as more clients are demanding buildings that can help them achieve their global net-zero targets.
Still, the needle continues to move in the opposite direction. According to the Global Status Report for Buildings and Construction 2019, the carbon emissions from real estate were at 39% in 2019, and in 2022, they reached their highest peak in 10 years.
Emerj CEO and Head of Research Daniel Faggella recently spoke with John D’Angelo, Managing Director at Deloitte, on the ‘AI in Business’ podcast to discuss how data and AI can potentially transform the real estate industry and how agents and firms can prepare for a new era.
In the following analysis of their conversation, we examine two key insights:
- Driving data-driven decisions and tailored strategies: Utilizing data in real estate to guide investment decisions, uncover market trends, understand population dynamics, and enable tailored strategies for success.
- Using generative AI for real estate leasing: Leveraging generative AI to streamline negotiations, mitigating risks by understanding agreed-upon terms, and optimizing revenue through data-driven insights into pricing and deal points.
Listen to the full episode below:
Guest: John D’Angelo, Managing Director, Deloitte
Brief Recognition: John D’Angelo is a Managing Director at Deloitte, where he is focused on operational transformation and innovation, working primarily with real estate operating companies, investment managers, and service providers. He is a certified master facilitator and was responsible for the West Coast collaborative work practice at Ernst & Young and then Capgemini.
Expertise: Real estate investment management, understanding and leveraging data, real estate industry organization.
Powering Real Estate Through Data-Driven Decisions and Tailored Strategies
John begins by discussing some challenges and unique aspects of transactions in the commercial real estate sector. He acknowledges that one challenge is the lower volume of transactions, especially in commercial real estate, which involves higher ticket prices.
John then highlights an essential characteristic of commercial real estate transactions, stating that the properties involved are like fingerprints – each one is unique. He emphasizes that one can’t generalize or apply the lessons learned from one commercial property to the entire market or similar properties across different markets. Each commercial property has its factors contributing to its transactability:
“I’ve got to know how long did somebody hold it? What did they buy it for? How much data did they have on it? Did they have leases that were recently signed or that are coming due in the next year? All those things contribute to what makes a building transactable.”
–John D’Angelo, Managing Director at Deloitte
John also discusses the challenges that large commercial brokerages face in handling vast amounts of information related to real estate transactions. He uses an analogy involving concentric circles to illustrate the layers of information these brokerages deal with daily:
- Size of the Universe: John describes the first circle as the “size of the universe,” representing the sheer volume of information that big commercial brokerages come into contact with regularly.
- Size of the Earth: John points out that only a fraction of the data brokerage firms come into contact with is collected and formalized into systems within this vast universe of information. He likens the amount of data therein to the Earth’s size, indicating a drastic reduction in organized and stored data.
- Size of California: Further narrowing down, John talks about the data connected across what’s collected, which he compares to the size of California. It suggests that interconnectedness and comprehensive analysis limitations exist within the collected data.
- Santa Monica: Finally, he describes the extent to which this data is leveraged, comparing it to the size of Santa Monica. The comparison emphasizes that only a minute fraction of the collected data is actively used and applied to gain insights or make informed decisions.
John then turns to the complexity of real estate transactions, highlighting that much of the crucial information — such as the details of buyer and seller profiles, market dynamics, and bidding competition — is often not fully captured in formal systems.
Instead, this rich information is scattered among individuals involved in the transactions, relying on storytelling and heuristics rather than comprehensive data systems. John tells Emerj that AI and analytics in these areas don’t yet prescribe answers but currently function only as guides by pointing out exciting patterns or opportunities.
John further discusses the intriguing potential of using algorithms to analyze vast data sets in real estate investment. He introduces the idea of reducing investment fees by leveraging algorithms to identify trends and patterns that may have yet to be initially considered. He mentions a common desire among real estate investors to find the next emerging market, drawing attention to the example of Nashville, which experienced growth due to its rising status as a healthcare center.
John further emphasizes that the unique nature of commercial real estate, which cannot be easily relocated, adds complexity to understanding shifts in population dynamics, especially in the post-pandemic landscape. The uncertainty about where people are moving and what they will need from the built environment further underscores the importance of data-driven insights in navigating these changes.
John also talks about the practical application of big datasets and various factors in understanding and making informed decisions in real estate. He mentions using satellite imagery to observe changes in parking lots and identifying trends over time.
By overlaying this information with demographic or migration data, investors can gain valuable insights into the dynamics of a particular area. John gives an example of a potential investment thesis, such as looking for new barista jobs to indicate an appealing neighborhood for walkability and mixed-use developments.
He emphasizes the importance of tailoring data and analytics to specific investment theses, as different real estate developers may have varying priorities and criteria. John finds it particularly encouraging that clients are experimenting with these approaches, suggesting that customization and strategic use of data can lead to more informed and successful real estate investments.
Using Generative AI for Real Estate Leasing
The discussion then moves to the challenges in real estate leasing processes, especially when dealing with retail or office leases, where terms can be unique, and negotiations often involve a significant amount of customized language. John points out the complexity of dealing with varied lease agreements and highlights the traditional heavy lifting required by legal departments to navigate these complexities.
He sees promise in applying generative AI to streamline the leasing process by quickly understanding and extracting information from many leases at once. It includes identifying commonalities or variations in clauses, so long as it is standardized on at least one party’s contracts.
Leveraging generative AI can expedite the leasing process and reduce the manual labor involved in analyzing and negotiating each lease agreement. John emphasizes that while it may seem mundane, using generative AI in this context has the practical benefit of efficiency and time savings.
John identifies three potential benefits or “knobs” that could be adjusted through the use of generative AI in the real estate leasing process.
- Efficiency in Agreement: The first involves reducing the amount of labor required to reach a lease agreement. By leveraging generative AI, the goal is to streamline the negotiation process and make it more efficient, ultimately saving time and resources.
- Risk Mitigation: Understanding and clearly defining what has been agreed upon with the counterparty can help assess and manage risks associated with specific clauses or leases. It involves gaining insights into the risk levels within a lease agreement without manually reviewing extensive documents.
- Revenue Optimization: Generative AI could provide insights into pricing and deal points by analyzing large market data sets. It enables a more informed approach to negotiating leases, tailoring requests based on a deeper understanding of market dynamics, and allowing for better revenue optimization.