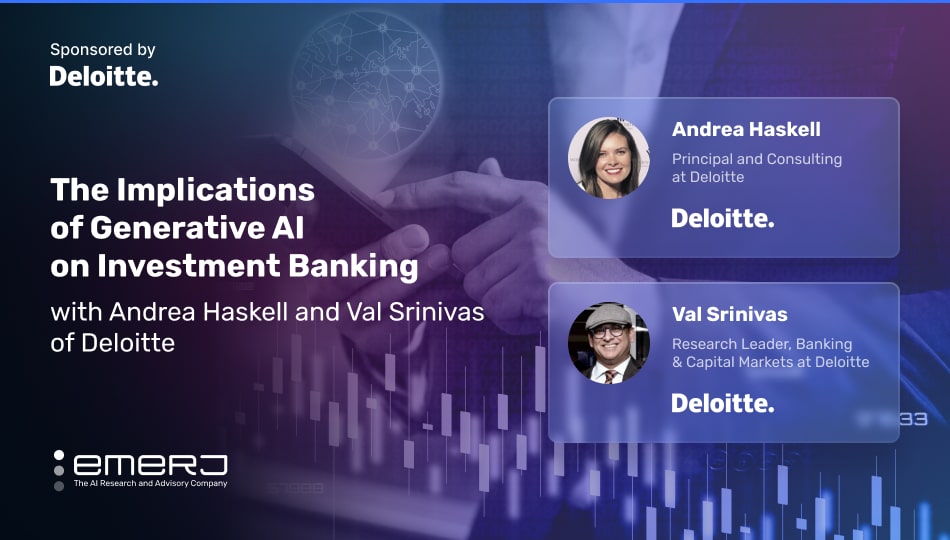
This interview analysis is sponsored by Deloitte and was written, edited, and published in alignment with our Emerj sponsored content guidelines. Learn more about our thought leadership and content creation services on our Emerj Media Services page.
In a recent report by Deloitte titled, “Unleashing a new era of productivity in investment banking through the power of generative AI”, the consultancy firm predicted that the top 14 global investment banks can boost their front office productivity by as much as 27-25%. This increase in productivity would result in an additional revenue of USD 3.5 Million per front-office employee by 2026, per the same report.
Even though the adoption of generative AI has been quick, the inherent risks of AI for international financial institutions must be addressed. According to the International Monetary Fund, these risks include privacy concerns, bias, performance robustness, and other potential cyber threats.
Emerj CEO and Head of Research Daniel Faggella recently sat down with Andrea Haskell, Deloitte Principal in Strategy and Analytics, and Val Srinivas, Head of Research at the Centre for Financial Services at Deloitte, to discuss new, emerging generative AI tools for news analysis and summarization in financial workflows and its uses beyond trading, such as M&A, advisory, and debt issuance. The trio also explores into how to prioritize the use cases for generative AI adoption.
The following article analyzes their perspectives and provides executives with the following critical insights from their conversation:
- Rethinking operating models: Rethinking operating models is essential when transitioning from proof of concept to production to ensure scalability, sustainability, and risk and control responsibly.
- Following a prioritization matrix for generative AI adoption: Mapping use cases on a two-by-two grid based on business value and complexity to help prioritize implementation, choosing between transformative yet complex opportunities and low-hanging fruit with lower complexity.
Listen to the full episode below:
Guest: Andrea Haskell Liptrot, Principal, Strategy and Analytics, Deloitte
Expertise: IT Strategy, Management Consulting, Analytics
Brief Recognition: Andrea Haskell is a principal consultant specializing in strategy and analytics at Deloitte. She has been a part of Deloitte for most of her career since 2009. She completed her Master of Business Administration in Economics and International Business from The University of Chicago Booth School of Business in 2014.
Guest: Val Srinivas, Head of Research, Centre for Financial Services, Deloitte
Expertise: Financial Market and Customer Insights, Thought Leadership, Strategic Market Research
Brief Recognition: Val Srinivas is the banking and capital markets research leader at the Deloitte Center for Financial Services. He leads the development of the company’s thought leadership initiatives in the industry. He has more than 20 years of experience in research and marketing strategy.
Rethinking Operating Models
Val opens up the conversation by mentioning the same Deloitte report on potential front-office efficiencies that can be unleashed for investment banks through generative AI. He then turns the conversation to integrating generative AI technology into existing infrastructure, systems, and processes. Val suggests that the focus is on improving cost efficiencies, productivity, and fostering revenue growth. However, Val acknowledges that, at the current stage, most entities are likely prioritizing efficiency and productivity gains over revenue growth.
Providing a use case, he explains that generative AI can improve productivity, especially for junior analysts at investment banks. These analysts spend a considerable amount of time manually gathering and summarizing information. Generative AI tools can minimize the manual work involved in this process, making it more efficient.
Even though the initial output of generative AI may produce summaries or paragraphs, it could branch into more divergent use cases as Val explains:
“How much time do we spend consuming audio content, podcasts, or videos, for that matter? As the use of non-text modalities increases around the world, you can imagine them becoming more and more important. Maybe there’s a picture of something happening, or there’s a video of some event – and before it even gets translated into text. How is that embedded into generative AI? And how do you take all that and create new outputs based on a combination of text, audio, video, whatever else?”
-Val Srinivas, Head of Research at the Centre for Financial Services at Deloitte
He then mentions how latency is an essential factor while trading securities. But the real question is if we can trust the output created by generative AI in a minimal period.
Andrea discusses current trends in the adoption of generative AI for investment banks and how the conversation has evolved. Initially, discussions focused on use cases and proving capabilities through proof of concept. Now, the conversation has become more holistic, Andrea tells the Emerj podcast audience, considering broader aspects of adoption.
Secondly, she discusses talent implications, including upskilling and reskilling younger employees. She even raises questions about the skills that the education system is equipping them with, versus what they might require when they enter the workforce.
Thirdly, Andrea points to rethinking operating models and addressing risks and controls responsibly. Addressing the critical considerations when scaling generative AI from a proof of concept to an actual production scenario is non-negotiable. She emphasizes the need for control points to instill confidence in the reliability of the technology’s outputs.
Andrea then provides a real-world example of a ‘doomsday’ scenario that could unfold if generative AI generates a public filing and a banking model extracts content from it to execute buy or sell orders on a mass scale. She discusses the potential risks, such as errors in public filings leading to significant consequences for businesses.
Following a Prioritization Matrix for Generative AI Adoption
When the conversation turns to prioritization, Andrea refers to generative AI as “augment technology,” trying to emphasize that generative AI is meant to augment human work, not replace it entirely. She mentions that it can enhance the value of work by allowing humans to focus on higher-order tasks, as AI would handle the more repetitive or ‘grunt’ work.
Andrea addresses misconceptions about the future role of generative AI and AI in general. She refutes the idea of an autonomous future where machines fully control the world in the near term. She highlights that throughout human history, inventions have been designed to free up human time and not to replace them.
Andrea specifically emphasizes generative AI’s nature as a tool that generates content and summaries. She acknowledges the concern about AI output sounding accurate and competent, leading to potential misinformation or false confidence. She stresses the need for safeguards to protect against such pitfalls, including addressing biases in training data and incorporating human oversight.
“Our data is biased, it’s just a fact. So how do we ensure that — with any data we’re training models on — that we’re using the right techniques and guardrails to fine-tune datasets that are not biased? [All] to try to ensure we arrive at outcomes that are as least biased as possible. Then again, back to the human-in-the-loop component: That’s where you really just cannot have fully autonomous systems in this area, and in the near term, because you need that human higher-order thinking.”
-Andrea Haskell, Principal in Strategy and Analytics at Deloitte
Additionally, she dispels the misconception that AI, including generative AI, possesses proper understanding or anything that can be described as a ‘moral compass.’ Instead, she specifies that these systems excel only at predicting the next word based on training data but lack the higher-order thinking of human agents.
The current focus of the conversation around the adoption of generative AI is strategically prioritizing use cases based on a framework that considers both business value and complexity. She mentions a visual two two-by-two matrix that helps in this prioritization, depicted in Figure 1 below.
Figure 1: 2×2 generative AI prioritization matrix, as described by Andrea Haskell.
The matrix involves mapping use cases against two axes: business value and complexity. High-value, highly complex opportunities (Square B in the figure above) might be transformative, representing a significant business case, but they may take time to implement. These could be part of a roadmap for future adoption rather than the initial starting point.
On the other hand, there are “low-hanging fruit” use cases (Square A) that offer business value with lower implementation complexity. In the context of banking clients, Andrea mentions examples of middle and back-office efficiency use cases that use internal datasets, minimizing reputational risk at an early stage.
By employing this framework, organizations can strategically choose where to begin their adoption of generative AI. As Andrea says, this matrix provides a way to experiment without reputational risk at this early juncture.
Later in the podcast, Andrea discusses the evolving job landscape in the context of generative AI adoption. She emphasizes that while some jobs may see a decrease in demand or even be entirely replaced, there will also be the creation of net new jobs. The key is to recognize that as AI becomes more integral, there is a growing need for fluency within organizations — specifically in data-, technology-, and AI fluency.
She notes a broader trend where every business leader is now expected to become a technology leader as well, highlighting that AI will not be limited to a specific group or department but will be integrated into various aspects of job responsibilities across the enterprise. These integrations will be seen as part of the overall enterprise strategy.
Finally, Andrea underscores the importance of reskilling the workforce to adapt to this changing landscape. The goal is to enable employees to interact with generative AI and similar technologies responsibly, thinking critically about the outputs they receive.