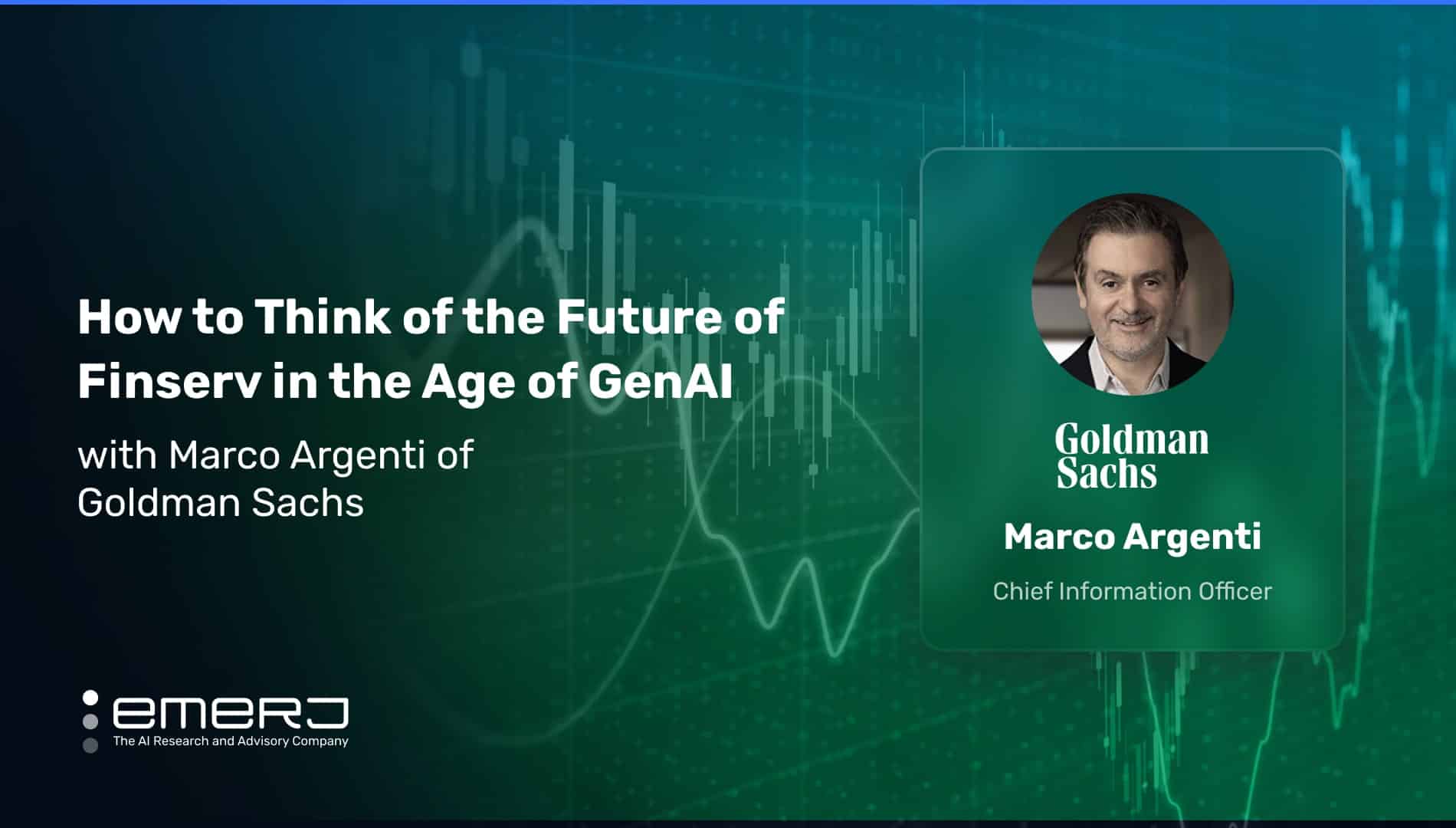
With the rapid proliferation of generative AI tools, there is growing anticipation of their potential to bring about notable changes in the financial and business sectors. Examples such as the creation of “the new Bing” – a combination of Microsoft’s Bing search engine and ChatGPT, which can generate more reliable and comprehensive answers – and other generative AI tools used to create videos for product design, have all contributed to the expectations within various sectors.
Considering the impressive capabilities of generative AI, its adoption within the realms of business and finance is projected to gain wider acceptance.
Emerj Senior Editor Matthew DeMello recently spoke with Marco Argenti, CIO of Goldman Sachs, on the ‘AI in Business’ podcast to discuss the role of AI in the financial services sector and how generative AI could help the sector build adaptability and resilience.
In the following analysis of their conversation, we examine two key insights:
- Adopting a ‘mixture of models’ to protect data and sensitive information: Using both large language and smaller, bespoke models to ensure the privacy and security of sensitive data and knowledge.
- Developing co-pilots to increase employee efficiency: Approaching generative AI “co-pilot” platforms as one would a “GPS” system for employees navigating data repositories in the enterprise.
Listen to the full episode below:
Guest: Marco Argenti, CIO, of Goldman Sachs
Expertise: Serverless computing, Internet of Things and augmented/virtual reality
Brief Recognition: Marco Argenti is Goldman Sachs’s Chief Information Officer. Before joining Goldman Sachs, Mr. Argenti served as Vice President of technology of Amazon Web Services (AWS) since 2013, where he started and ran several AWS businesses. Mr. Argenti serves on the Board of Advisors for the Fred Hutchinson Cancer Center and is a board member emeritus of the Pancreatic Cancer Action Network.
Adopting ‘Mixture of Models’ to Protect Data and Sensitive Information
Marco acknowledges that financial services can significantly benefit from AI but emphasizes the importance of being aware of potential dangers and pitfalls that come with its implementation. Marco sees generative AI as the next technological evolution.
He discusses the challenges and risks of using large language models in AI applications. He addresses two specific categories of risk: information-related risk and intellectual property (IP) or data protection risk. The speaker emphasizes that the problem can be viewed from various dimensions. He proceeds to explain two main types of risks:
- Information-Related Risk: It pertains to the accuracy versus plausibility of the information generated by large language models. This risk focuses on the challenge of ensuring that AI-generated content is not only plausible but also accurate and reliable.
- IP and Data Protection Risk: It is about protecting intellectual property and data. The speaker discusses how organizations use large language models, some of which are open source, and how they can run these models on-premise or within a controlled virtual private cloud (VPC) environment. The critical concern is safeguarding proprietary data and IP, especially when using open-source models or third-party services.
Marco acknowledges the challenge of leveraging these advanced capabilities while safeguarding sensitive information. To address these concerns, the speaker mentions that companies like Microsoft and Google have taken steps to create enterprise versions of their public offerings. These enterprise versions prioritize data security by turning off certain features, ensuring data protection and moderating interactions with human agents. These models are inherently stateless, meaning they need to retain information.
He further describes a concept similar to a “mixture of models” where larger AI models, like generative language models, are used to establish a general reasoning framework. These larger models act as orchestrators or high-level decision-makers.In this pattern, these larger models communicate with or call upon smaller, specialized models through function calls or agent-like processes. These smaller models are trained to handle specific topics or tasks and are used to process and distill knowledge in a more focused and specialized way.
This approach aims to strike a balance between the capabilities of large, general-purpose AI models and the need for data protection and specialization in certain areas of financial services. It allows organizations to leverage the power of generative AI while ensuring the privacy and security of sensitive data and knowledge.
He highlights a common challenge where a knowledge gap exists between experienced professionals and newcomers. According to the speaker, AI can act as an equalizer by helping individuals, including those not experts in a given field, understand complex concepts and make effective decisions, potentially leading to a significant boost in productivity.
Marco provides an example of how AI can benefit technologists and coders. He explains that AI can assist in understanding and explaining code, translating it into different programming languages, and even generating new code. The speaker emphasizes that this can save time and enhance efficiency, particularly in organizations with many developers.
The speaker envisions a future where AI can understand internal data sources, APIs, and models. He describes a scenario where AI can generate code in response to natural language queries, summarizing data, augmenting it and presenting it in an easily understandable format.
Developing Co-pilots to Increase Employee Efficiency
Marco discusses workflows, expressing frustration and passion. He describes workflows as complex processes involving state changes, which can occur frequently and rapidly in financial transactions, such as payments, balance changes, credits, debits and equity transactions.
Traditionally, workflows involve digital representations of standardized processes translated into instructions within corporate systems and workflow execution systems. However, the speaker points out a significant challenge in automating workflows: the intrinsic fragility of digital workflows. He uses an analogy of navigation, comparing it to following detailed instructions for every turn when driving, which becomes impractical as processes adapt and change.
Organizations often navigate the complexities and changes in processes similarly to how drivers adapt to road conditions and detours. Automation attempts, such as robotic process automation, can break when faced with unexpected shifts because the systems implementing these workflows lack the flexibility to adapt.
The speaker emphasizes that humans can react to process breaks, adapt and apply quality controls when needed. In contrast, machines are rigidly programmed and struggle to handle unexpected deviations from predefined workflows.
He introduces generative AI as a solution to this problem. He illustrates this with an example where a user can request specific information extraction from incoming documents and data sources by providing a declarative prompt.
The speaker draws a comparison between the capabilities of AI and GPS navigation. He explains that AI can reason and determine appropriate steps, similar to how GPS systems calculate the best route based on real-time information and conditions. AI’s generative nature allows it to be flexible and adapt to changes similarly.
The speaker envisions a future where companies evolve, like the evolution of networking and infrastructure. He mentions concepts like “as a service” and “software-defined infrastructure,” where networks and infrastructure can be reprogrammed and reconfigured as if pushing a new software version.
He proposes applying a similar approach to enterprise processes, where companies can deploy new versions of their operations and train AI to reason dynamically about these processes. This dynamic adaptability would make the processes more resilient to change.
He also emphasizes that AI can bring intelligent process agents into play. These agents would be highly aware of their context and capable of staying updated in real-time, even after their initial training. They could access external data source endpoints and undergo fine-tuning more frequently. This flexibility would enable them to navigate processes efficiently, adapting as needed.
In the end, he highlights his belief in the versatile capabilities of generative AI. He sees a potential for generative AI that goes beyond its traditional role of content generation, such as writing text or generating creative works. Instead, he envisions generative AI being applied to developing and adapting organizational processes like process generation and production processes.
The speaker discusses how generative AI can retrieve and process complex information effectively. He presents a scenario where someone wants to analyze a complex and multifaceted situation. For instance, he mentions various hypotheses, such as the potential disruptive impact of generative AI, the scarcity of GPUs, geopolitical factors and inflation trends.
He describes how generative AI can help in such scenarios by determining which information sources to use. This decision-making process involves training data, document retrieval, prompt extension and API integration.
The speaker introduces the concept of “prompt expansion,” where we provide AI with a document as a prompt and ask for more information or context related to the topic in question.