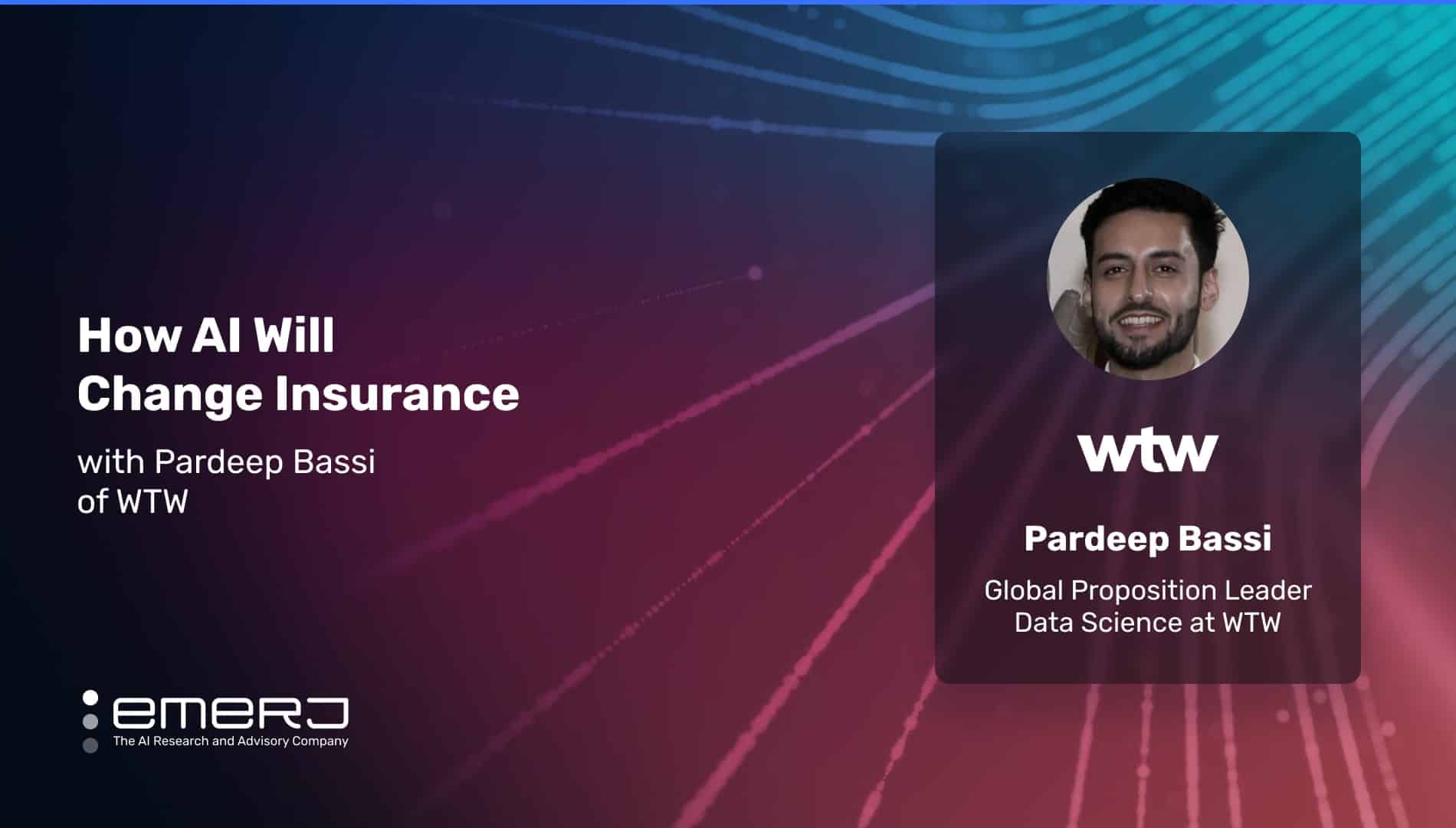
Like other legacy and document-dominated sectors, insurance is experiencing a digital transformation with AI as the main driver. AI and machine learning are enhancing everyday insurance processes, including quote generation, claims processing, personalized policy recommendations, claims fraud detection and prevention, and various customer-facing tasks.
Regulatory compliance is a significant variable in the insurance business model. However, we rarely hear AI and machine learning mentioned in the same breath as regulatory compliance in the insurance industry by comparison to the previously mentioned use cases. Should it be?
To help answer this question, we recently hosted a conversation on the AI in Business podcast with Pardeep Bassi, Global Proposition Leader of Data Science for Willis Towers Watson (or WTW), a publicly-traded financial services firm based in the UK.
This article will analyze our conversation with Pardeep Bassi , breaking down two principle insights relevant to business leaders across a broad range of industries:
- Using machine learning to counteract bias: Emerging AI capabilities can not only help insurers better comply with new regulations across insurance designed to reduce bias in quoting policies—but find ROI as well.
- Optimizing compliance operations with algorithms: Choosing the correct type of algorithm – including newer “bespoke” models – can help insurers balance the dual demand of regulatory compliance and maintain predictive power.
Listen to the full episode below:
Guest: Pardeep Bassi, Global Proposition Leader of Data Science, Willis Towers Watson
Expertise: Data science, machine learning, corporate strategy
Brief Recognition: Before joining WTW, Pardeep held various data science leadership positions at AXALV insurance and others.
Using Machine Learning to Counteract Bias
As a data-rich industry, Pardeep expresses to Emerj how insurance is a good candidate for AI and machine learning on a large scale. Many insurers are already using automation to extract business value and gain an edge over competitors. As a result, there has been a massive increase in AI adoption.
While certainly an excellent candidate for AI adoption, Pardeep cautions that insurers must either balance AI adoption with regulatory requirements or understand where they overlap. “At the same time, insurance is a highly regulated industry, and you need to understand where there is an overlap of [AI and regulation].”
Part of understanding this overlap is distinguishing between two types of regulation:
- How AI is used in the insurance space
- Insurance regulations writ large
One of the main criticisms of AI and machine learning is the potential for bias. In the EU, enough criticism was raised to result in an agency directive requiring insurance companies to omit gender as a factor when pricing insurance policies.
Pardeep explains the industry response: “There was a huge effort to understand ‘How we can do that as part of our modeling while still keeping the same predictive power?'”
This reaction is not surprising; as Pardeep alludes, there’s always inherent bias in the human thought and decision-making process. He makes the cogent point that, as humans are not bias-free, how can we expect the machines that depend on human input in the form of training data to be free of it?
Pardeep explains this point in the context of algorithm design and predictive power. “Algorithms are usually built off existing data of previous experiences. And you use that data to try and predict the future. So…you may already have an element of bias in the data.”
Given the inherent bias in any human-generated dataset and, consequently, data model, was it possible to build a solution without bias? What can be done in such a situation?
To accomplish this, Pardeep says it is necessary to understand what data already exists and use some algorithmic technique to overcome it. He is adamant that machine learning algorithms can reduce bias, and more insurers are using them to do just that.
“There’s an additional focus on specific types of algorithms which actually reduce bias in the model build phase,” Pardeep explains. “We could use machine learning and AI to actually remove bias from our processes.”
Pardeep goes on to argue that machine learning and AI should be seen as potential solutions to the bias problem, not as an impetus for more regulation.
“It was easier to understand what you were doing and be in line with the regulatory requirements as well as your profitability requirements by using machine learning and AI as a tool,” says Pardeep. “Without machine learning and AI as a tool, it would have been very, very difficult.”
Choosing the Right Algorithm for Optimizing Compliance Operations
Pardeep states that designing business processes around regulatory requirements puts the organization in a better position. In so doing, an organization can potentially put itself in a profit-making situation:
“I think we as an industry need to take a step back and say, ‘Our public image is very important, and so is our brand. But it’s our understanding of the regulation with what’s the actual reason, the intention, for the regulation rather than just how do we adhere to it, but maximize our profitability?”
– Global Proposition Leader of Data Science at Willis Towers Watson, Pardeep Bassi
He states that “price walking” compliance is an excellent example of how AI and machine learning can help meet regulatory requirements. Price walking is a business practice where the policy is written at a loss to attract the business and then increases the price on insurers who renew year over year.
In the case of price walking, insurers can reopen their respective “back books,” or renewal books, customer risk segments for which they’ve previously written policies. Underwriters could use AI and machine learning to determine the ideal price to charge a customer or group of customers within a given risk category, given the constraints posed by the new regulation.
In this way, insurers could “charge the customer with the constraint that the new business and renewal price has to be the same, and almost keep as many customers as possible, but still have a reasonably competitive price for new business.”
“Depending on the segment that you have as your back book, and at quite a granular level, you could use machine learning and AI to help you determine what price to set to meet the business requirements, as well as the regulatory requirements of having enough repeat customers, loyal customers, as well and writing new business.”
– Global Proposition Leader of Data Science at Willis Towers Watson, Pardeep Bassi
Pardeep states that the rate at which additional regulations are introduced will only increase. Accordingly, insurers must be able to adapt and demonstrate flexibility in devising new sets of prices and predictions. “It’s that ability to change and adapt which AI and machine learning is a facilitator for.”
Another way it benefits insurers to structure operations around regulations is by balancing interpretability (being able to explain their models and understand the value and accuracy of their findings) with regulatory requirements.
He uses a regulatory facet of GDPR as an example: “One component of GDPR states that you need to be able to explain your decision to the customer. And that can be interpreted in many ways, but one way is, ‘What are the most important influencing factors which have helped the insurer make a certain decision about [someone]?”
What are the AI and machine learning ramifications of such a statute? For the insurer, Pardeep says, it is choosing the correct type of algorithm, allowing the insurer to answer the above question satisfactorily for themselves and the customer.
However, Pardeep emphasizes that choosing the correct algorithm goes beyond regulatory adherence. It contains enough predictive power to ensure competitiveness and profitability.
Designing “bespoke” algorithms with the insurance industry in mind is where he sees things going. These algorithms serve the dual-purpose mentioned earlier: satisfying regulatory requirements while maintaining predictive potency.
“This is where I see the future of insurance and AI, ethics and regulation all overlapping to really push the bounds, which is custom AI algorithms to meet insurance needs, with a specific focus on the ethics and regulatory elements,” Pardeep explains.
When asked for an example of an algorithm that maintains this high level of predictability while offering good interpretability, Pardeep states:
“The shift we’ve seen in more recent times is more of a move towards tree-based models. So a lot of XG boost and various other algorithms. A tree-based model can be thought of as multiple if statements … If you take a combination of your need to interpret as well as keep the predictive power, you can create a balance in terms of what type and when these splits occur.”
– Global Proposition Leader of Data Science at Willis Towers Watson, Pardeep Bassi