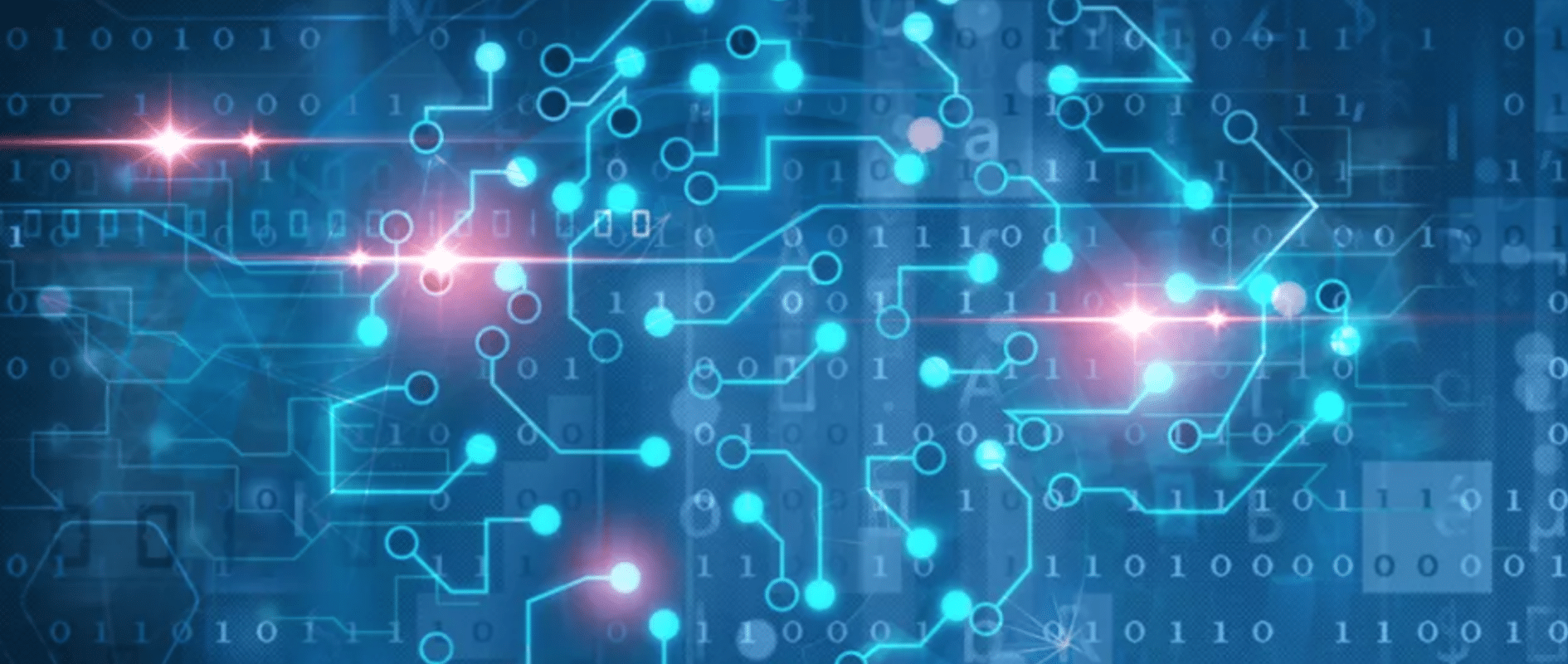
Rather than coming up with completely new processes or products that involve deep learning, companies say they are using this AI technology to expand on functions or tasks that already existed at their organization, according to a new report published by O’Reilly.
The study, titled “How Companies Are Putting AI to Work Through Deep Learning,” surveyed 3.300 of their “AI,” “data,” and “programming” newsletter subscribers to assess how and why their companies were implementing this deep learning, as well as potential roadblocks and future interests in AI. The publication did not note whether subscribers to these three newsletters were enterprise or general subscribers.
To further investigate the use of deep learning in companies, researchers also surveyed participants to gain answers to the following questions:
- What are you looking to apply deep learning?
- What are your deep learning bottlenecks?
- What are you doing for talent and training?
What Are You Looking to Apply with Deep Learning?
According to the survey, most respondents say they use deep learning to make sense of structured or semistructured data or text. O’Reilly notes that this could be because deep learning is seen as an “upgrade” to traditional data analysis techniques.
“Upgrading familiar applications with deep learning is a safer investment than starting something new; businesses have lots of structured and semistructured data already; and the number of businesses that can currently make use of computer vision (to say nothing of gaming) is limited.”
When it came to deep learning applications that were most interesting to respondents, 13 percent pointed to computer vision, closely followed by text mining at 11 percent and enhancing existing data and machine learning systems at nine percent. Other options, showing a three-percent interest or less, were finance, forecasting, speech technology as well as health and medical.
The chart below shows a breakdown of how respondents answered the question:
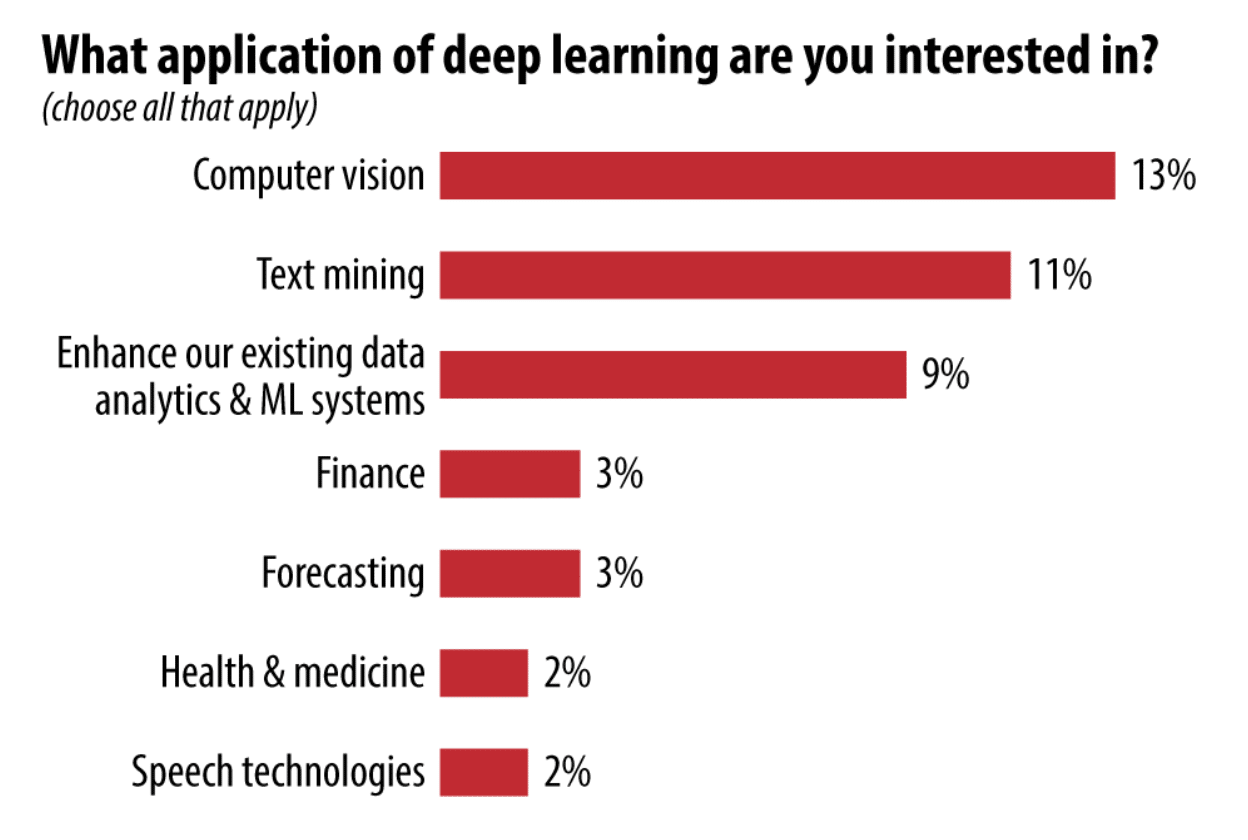
Computer vision – which allows a software to analyze, label and identify patterns and other aspects of a photo – has gained interest in a variety of industries including retail, automotive and agriculture, with companies like Amazon, Google and Tesla releasing products that use the AI technology.
While Amazon’s patent history is evidence that it will use computer vision, along with augmented reality, to recognize and dress humans on its virtual mirror, Google and Tesla claim their self-driving cars use the technology to detect and avoid obstacles and moving objects.
From our research, we’ve seen that text mining has become of strong interest in the healthcare industry and in regards to regulatory technology (RegTech), as well as other sectors which are associated with tracking, documenting and maintaining backgrounds and records of patients, customers and users.
What Are Your Deep Learning Bottlenecks?
While the report notes that there are now over 22,000 PhDs with a background related to deep learning and AI, it also adds that finding and training AI talent is still the biggest roadblock reported by survey participants. According to the chart below, the number one bottleneck faced by respondents aiming to grow deep learning at their companies is a “lack of skilled people.”
When we spoke with Ben Lorica, O’Reilly’s Chief Data Scientist, he explained why determining required AI talent was key to implementing processes like deep learning or machine learning.
“People focus a lot on the model but when you get to the details it’s about more than building a cool model,” he explained.
While he noted cloud systems that could limit the number of staff needed from a job, he notes that it’s important to analyze who will collect the data and how a machine learning software will be maintained.
“You probably will still need people to make sure data pipelines are flowing and models are working,” he said, adding, ”You can feed data into a library and get a result but you still need experience and training to interpret those results.”
Lorica recommends having a combination of data engineers and data scientists on hand, just incase a model “goes bad.”
“There’s this notion of concept drift where your model gets stale and they need to be retrained. You need someone to recognize that or set up alerts for an automated way of learning.”
Although it seems difficult to find someone with strong machine learning experience, larger companies have still begun to make it into its own role.
“These days there’s even a new job role called the machine learning engineer, which kind of bridges that gap with someone who specializes more in production of these technologies,” he said. “What we’re finding in our surveys is that the companies that have much more experience in AI and machine learning tend to have this role in-house.”
Other Bottlenecks included:
- Hardware and Computer Resources (9%)
- Data Related Challenges (8%)
- Company Resource and Culture (3%)
- Accuracy and Efficiency of Deep Learning Models (1%)
O’Reilly also notes that nine percent of participants reported no current bottlenecks to applying deep learning.
What Are You Doing for Talent and Training?
In response to the most common roadblock of skill gaps, 75 percent of participants reported that their companies have implemented in-house or external training programs for general AI skills. The study did not specify which AI skills were discussed in these programs.
While 49 percent said these training programs were done within their company, 35 percent said formal training came from a third-party source. Over 18 percent reported that they have sought training from individual consultants. Aside from noting that third-party sources could be events or online-resource related, it did not go into detail about other third parties and how they might be different from consultants.
The chart below shows how respondents described their training strategies:
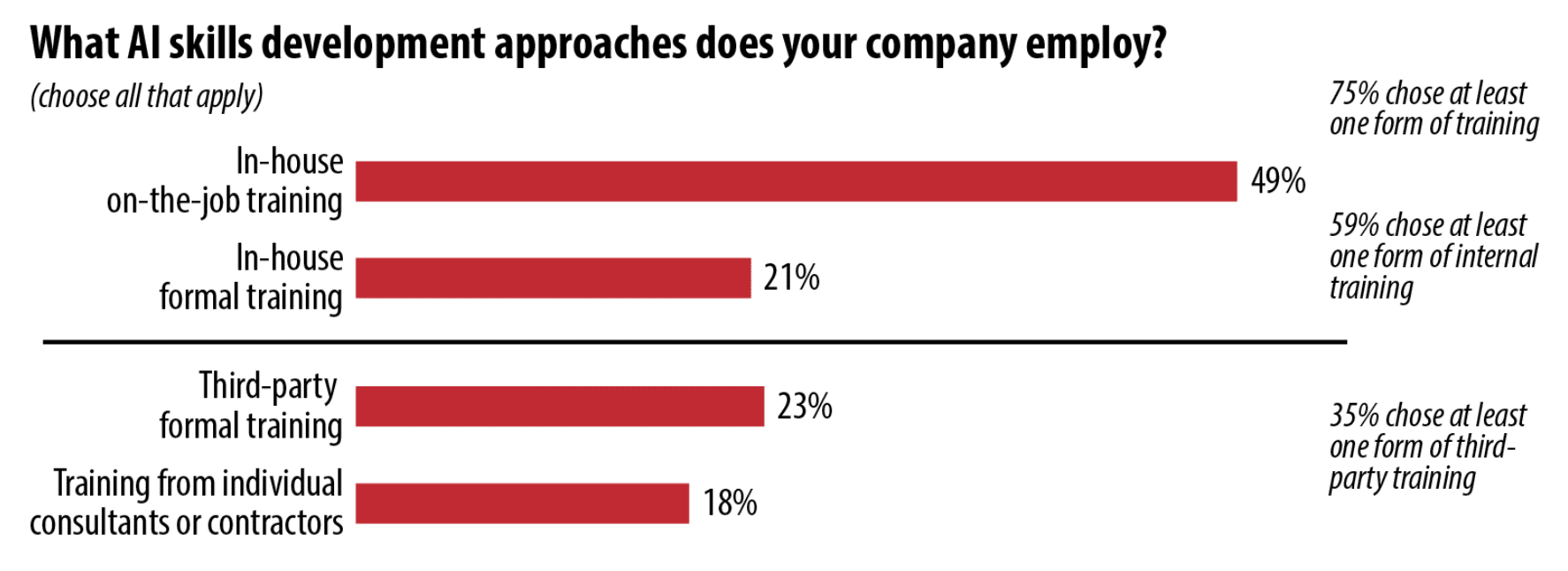
One form of third-party training could include workshops or events. In a recent AI in Industry, we spoke with Nikolaos Vasiloglou from MLTrain about how his history with training events to assist employees in upgrading existing skills to fit AI.
Vasiloglou says that typically, events he’s attended run for two days, with five hours of training each day. Although he says that with this format, attendees are interested in more training time, strong AI events are focused on basics rather than burning attendees out on information.
When it comes to training events, the respondents and Vasiloglou similarly say that the best strategy involves “upgrading” similar or relatable skills, and sending an employee whose expertise meshes well with AI, rather than training someone from scratch.
“There are two things people are getting out of these events. First of all, it’s a good way to refresh the fundamentals.
He explained that these fundamentals could include topics like linear algebra. “If you don’t know [these fundamentals], you can’t really understand how deep learning — which is the big thing — works,” he explains.
Vasiloglou says events also may allow attendees to begin to learn more about and discuss modern ideas like neural networks. “Basically for them it’s a kickstarter. In two days, they get an idea about where to start and what is important,” he says.
Through various events, he says attendees are able to “get the big picture and connect the dots.”
He added that outside training can be more helpful than individual research.
“if you start reading blogs and disconnected notes of the web, you feel like AI is 100 different things,” he warned. “But, if you just learn the basics and fundamentals, you realize that it’s just one or two concepts.”
Through events he’s attended, he says he’s seen a “super mix” of attendee backgrounds. While he’s noted software engineers that are experienced or fascinated with AI, he’s also seen data scientists who may not have time to research and learn about AI in their own time or in a work setting. Sometimes, he also notes that people with less experience in both areas just attend events to gain “pointers” on the best ways to train their staff.
Concluding Thoughts
From this report and our previous research, we are seeing the following themes in companies applying AI and deep learning:
- The study notes that a majority are interested in computer vision and text mining. Similarly, TechEmergence researchers have noted that computer vision products have increasingly been released in retail and automotive industries, while text and data mining has been used in finance, healthcare and other document or regulation-heavy industries.
- While companies continue to gain interest in deep learning, there also continues to be a talent gap. Study results hint that companies have implemented education processes or invested in outside training. Through our interviews, we have also seen that companies are interesting in finding and pursuing the right talent for machine learning goals.
- Regardless of the training style, rather than training someone from scratch or seeking talent with AI expertise, many companies say that they place employees in training whom already have a background that may relate to the fundamentals of deep learning. These employees could include software engineers and data scientists.
O’Reilly and Lorica both note that there are a number of things to consider when plotting a new deep learning project.
“The move from a pilot to production is more complicated than some people might think depending on mission criticality of the project,” Lorica says. “We are now entering an age where we have to have all these considerations beyond business metrics and machine learning metrics.”
This article was sponsored by O’Reilly Media, and was written, edited and published in alignment with our transparent Emerj sponsored content guidelines. Learn more about reaching our AI-focused executive audience on our Emerj advertising page.
Header image credit: CFA Institute