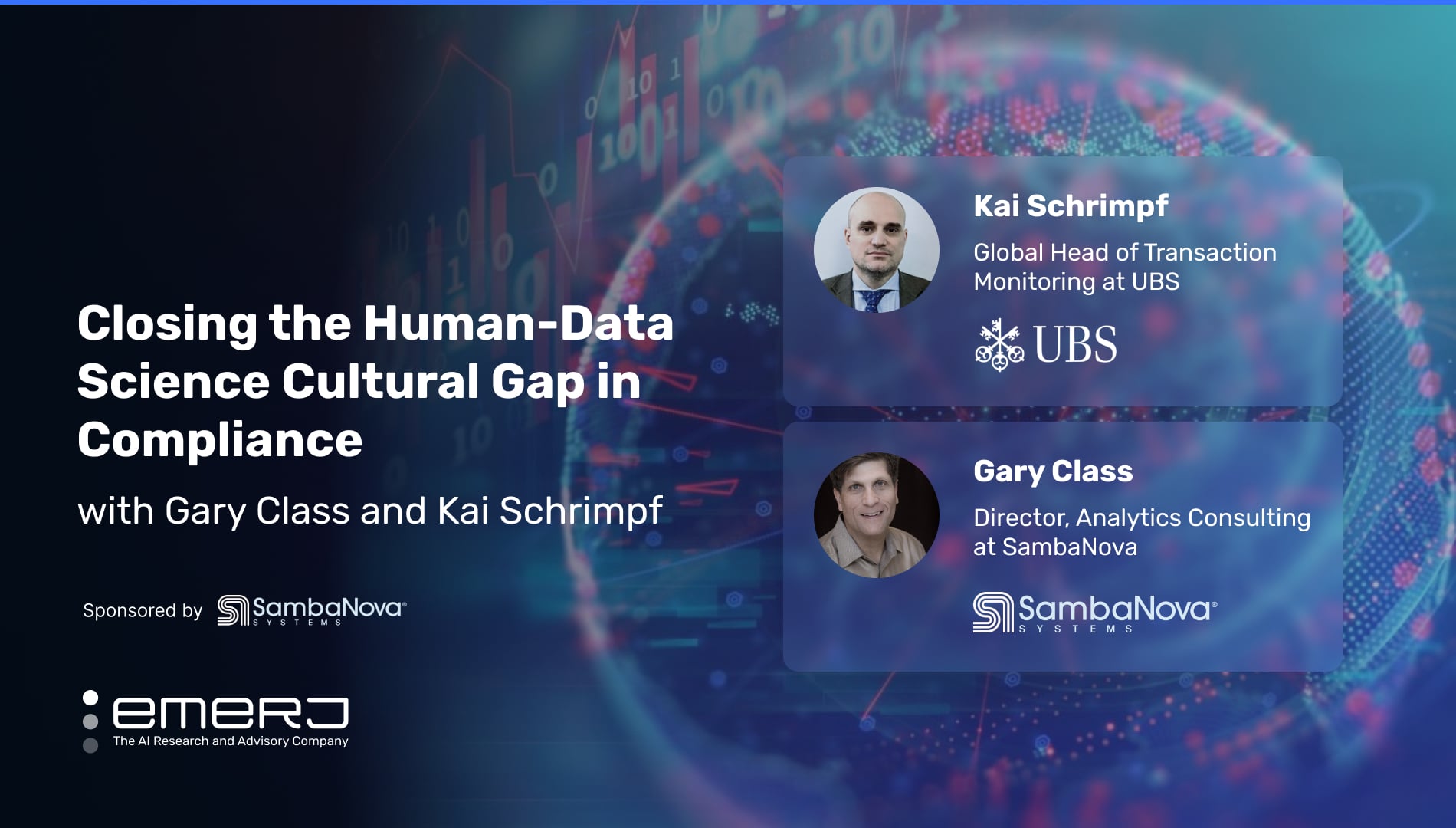
This interview analysis is sponsored by SambaNova Systems was written, edited, and published in alignment with our Emerj sponsored content guidelines. Learn more about our thought leadership and content creation services on our Emerj Media Services page.
Translating great ideas from data science teams into a language business leaders can understand is one of the biggest challenges facing early AI projects for medium and large enterprises. It helps to have shared interests between these groups if shared goals are better realized.
In the financial services sector, compliance is usually the first reason driving data professionals into corporate c-suites. According to a recent report from McKinsey, regulatory penalties are on the rise and directly correlating with how data is becoming a more significant part of the conversation.
Compliance is also among the leading focuses of early AI projects for financial institutions (FIs) as these concurrent industries emerge from the pandemic in a new wave of digitalization. According to Deloitte, AI capabilities stand to provide FIs new opportunities that extend far beyond cost reduction and efficient operations – including “as a service” offers from business functions previously thought of as cost centers.
Emerj recently spoke to two banking compliance experts – Global Head of Transaction Monitoring at UBS Kai Schrimpf and Gary Class of SambaNova – about significant trends in compliance and data throughout particularly large legacy FIs.
In their brief conversation, they discuss three significant observations about the challenges facing financial services organizations trying to get data science and business leadership teams to work in concert:
Key Insights:
- Compliance as the “front door” of organizational interest in data: The most significant reason non-coding leaders have to be interested in data in the first place is meeting regulatory demands and avoiding penalties
- Communicating data value through rankings and pain points: Data science teams can make data trends meaningful to non-coding leaders by associating visual presentations with specific value rankings and examples of negative customer interactions and outcomes.
- The ‘full integration of data is the future: Data’s future in banking lies in centralizing how core business functions know and trust customers, no longer letting the process be replicated unnecessarily between siloed departments
Guest: Kai Schrimpf, Global Head of Transaction Monitoring, UBS
Expertise: Compliance, anti-financial crime operations
Brief Recognition: Before working with UBS, Kai served in compliance-related responsibilities for various legacy FIs since 2016, including Credit Suisse, Deutsche Bank, and Goldman Sachs.
Guest: Gary Class, Director of Analytics Consulting, SambaNova Systems
Expertise: Data analytics, Customer journey management
Brief Recognition: Gary Class spent 34 years helping to build much of Wells Fargo’s data and security infrastructure before joining Samba Nova. Previously, Class worked as an economist at what is now the worldwide NGO Global Insight.
Compliance as the Front Door of Organizational Interest in Data
Both guests agree banking leaders have become interested in data because of the increased regulatory burden on banks to provide more and higher quality information and avoid penalties. In turn, this has revealed how dispersed essential information is across core business functions of most financial institutions:
“The data is disparate; all different lines of business in the bank have their own data, data processes,” says Gary. “There’s even some issues with how do you integrate that data, like what really is a customer? And how do you define a customer where there’s no struggle, there’s certainly a lot more focus on it.”
In this way, as Kai agrees, banks are in their own way of centralizing the data – and usually, data scientists in the form of compliance officers are stuck with leading the charge and educating their leadership on the wasted energy they see across departments.
He cites when recently UBS was fined $10m for not monitoring their communications, but also did not possess the data fluidity and governance necessary to conduct communications surveillance properly.
“My task is to monitor, to protect the bank from white collar and blue collar crimes and money moving that’s not supposed to be moving,” says Schrimpf. “I shouldn’t be explaining why I need this data. I should not be spending a month preparing PowerPoint decks and having meetings and alignment meetings every time in order to get something that I should already have. And that is tremendously frustrating right now.”
Through that frustration, data scientists are at least able to get leaders from across the organization into the room to take data seriously. Once they take data seriously, they can realize not just penalty-avoiding potential – but taking a long-term strategic view of data’s true revenue-generating potential for FIs.
AI Adoption Relies on Simple Communication in Visualizations
That cultural “gap” in understanding data comes from two distinct sides with a specific communications barrier:
- “Data-rich, intelligence-poor” stakeholders, many with law and humanities-based backgrounds – leaders who are not intrinsically motivated by significant representations of data-based concepts.
- Compliance officers are more accustomed to proposing and defending ideas in academic environments to data science audiences who already share their STEM backgrounds and vantage points.
To overcome this gap, compliance officers must understand how to register meaningful reactions and narratives from their visualizations and other data presentations.
Gary Class brings up his experience developing customer journey analytics for large banks and how the presentations that got the strongest reaction from non-coding stakeholders derived significance from the embarrassment of hearing customer calls gone horribly wrong.
Nothing consolidates what disparate data points between departments mean to the customer experience “more than the embarrassment we all felt when we heard this customer struggling,” Gary tells Emerj.
Customer journey analytics involves three major components:
- Collect enough data from customers
- Developing internal systems from that data to find out when customers have banking tasks
- And help that customer resolve the task ahead of time
From Gary Class’s experience in banking compliance teams, “the more disparate types of data that we can aggregate together, then the sooner we can identify what the customer’s needs were. And the more effective the organization could be in resolving it, which did have a positive spillover effect to some compliance activities.”
Once data professionals can reach stakeholders emotionally in their presentations, they can make a more significant case for how to think of data. Instead of only turning to data in a fraud detection and regulatory compliance context, CDOs arise from where data is understood as a driver of business fundamentals: getting a complete, centralized 360-view of the customer.
Class makes clear that the point is, instead of pointing to solutions, to rethink the problem and come up with the most elegant, sophisticated solution to a “more holistic view of collecting all the data, normalizing the data, interpreting the data about your customers, and their behaviors.”
Class continues: “And then having your family of models goes after those to identify what’s really important, asking: ‘Is that really anomalous?’ ‘What’s this customer trying to achieve?’ ‘Does this transaction look fishy to me?'”
The best strategy is to focus on where data can communicate “cross-functional knowledge” to help transfer non-coding leaders and stakeholders better understand the true capabilities of AI to transform organizations at the level of business fundamentals.
In Class’s words, you want to “Make the lightbulbs go off” when bringing meaning to new metrics that feel like teachable moments to non-coding staff. Fundamentally, he says, “it’s an internal sales process.”
Kai Schrimpf notes that similar results can come from teams sharing value in influential and organization-wide numerical rankings in data visualizations. When these rankings are shown exactly where they can elicit the most meaningful response in the non-coding audience, “even on things that are not comparable,” – the communications links are invaluable.
Numerical values/emotionally compelling visualizations translate to transaction monitoring becoming a big focus – and significant expense – for banks, beyond just where the money’s going, but in meeting rising standards in knowing your customer regulations. The bottom line: Keep communications simple and tell compelling stories with your data.
The ‘Full Integration of Data’ is the Future
Client analytics and mapping the entire client journey through data is a worthy early AI project for a medium to large-sized FI, says Kai Scrhimpf, usually started in the business line, wealth management, IP, “or whoever [in the organization] owns the client relationship.”
This line of business “knows everything about this client,” Kai tells Emerj – with narratives backed by data that many in the compliance space don’t even have their hands on yet at this initial stage of the digitization process.
No matter where the conversation begins, developing that journey is leading many FIs to one destination that Kai Scrimpf describes as the future of data in banking, or what’s referred to as the ‘full integration of data.’
The process involves consolidating and centralizing essential data functions currently spread out through siloed departments in a typical banking organization framework. An example that Kai is familiar with from UBS is in full name identity resolution or trusting that a customer is the same no matter where they are located – a particular concern in the wealth management space.
It is also a critical function for compliance professionals trying to detect anomalous behavior. Still, these business functions are typically siloed, even if they have the same interest in verifying customers’ identities.
New AI capabilities stand to centralize these processes and guarantee functions like name identity resolution across these siloed departments. They are driving many early AI projects for medium and large-sized financial institutions.
In consolidating these functions, organizations will pursue an “architectural thinking of reusability,” Kai tells Emerj.
In the process, a centralized and comprehensive approach to data will produce a 360-view of customer behavior at the organization – and from this, analytics can derive deeper, revenue-driving insights, such as mapping out a customer journey.
Gary Class outlined the following inputs in developing Wells Fargo’s customer journey model:
- Digital banking data
- Branch data
- Call center data, including negative call transcripts and speech analytics
- IVR
With this broader data set, customer journey teams can detect “a nonlinear increase in our ability to understand the signal from the noise,” Class tells Emerj. “Which helps us a lot with everything that we were trying to do with improving customer experience and a collateral impact.”
Realizing the value of data outside regulatory and compliance contexts became a big part of their business strategy: “A precious level was really enhanced by our banker surveillance program — to understand communication between bankers and brokers and customers, which led us and put us in a good spot,” says Class.
Fixing this issue is where Schrimpf feels the focus of bank digitization efforts will be over the next few years, leading to organizations developing “a unified architecture of data enriched by whatever specific AI use case you have.”
For any organization, such a digitization journey will see serious ROI when organizations can make central information processes verifying trust points with the customer available to:
- Control functions
- Audit functions
- Model risk functions
- Other business lines for opportunities
The final point is crucial, Schrimpf tells Emerj, so you can avoid potential partners that will only de-centralize your data.
If your entire organization understands the value of centralized data, says Schrimpf, it will be easier to see past these opportunities and towards a larger vision. You can tell these vendors with confidence that:
“… I’d rather trade with the same shop that I [work with for] wealth management, so that you have all your natural language processing, identity resolution, customer journey, compliance — all of that, and we can all access the same data. Not just a compliance data lake or wealth management-specific data lake, but a firm-wide data lake, which enriches itself to whichever business processes are underlying it. That to me is the future, and that to me will get us forward faster than any isolated solution that I can do in compliance or isolated solution at the front desk by themselves.”
– Global Head of Transaction Monitoring at UBS Kai Schrimpf