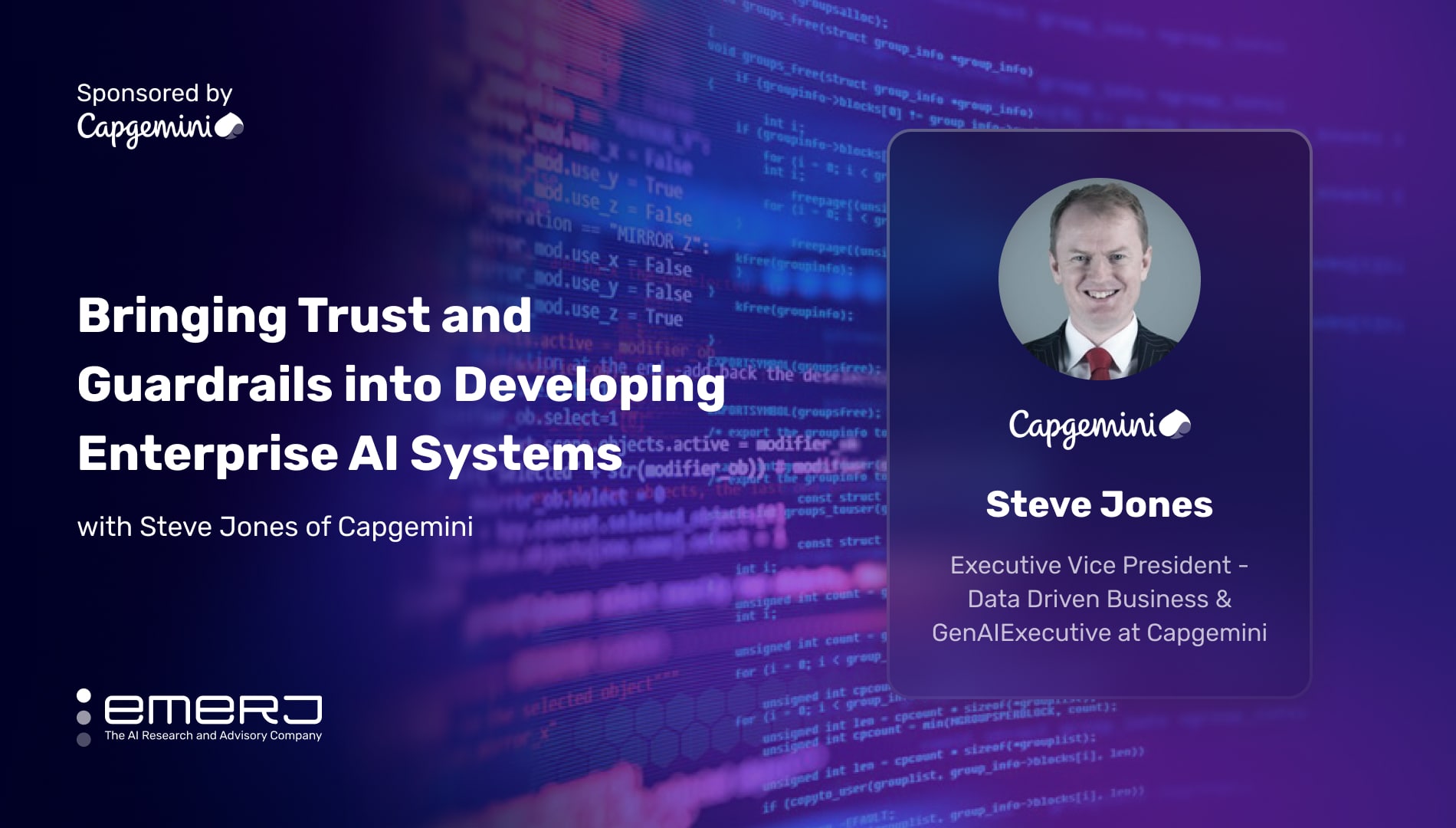
This interview analysis is sponsored by Capgemini and was written, edited, and published in alignment with our Emerj-sponsored content guidelines. Learn more about our thought leadership and content creation services on our Emerj Media Services page.
Two years before generative AI redefined “hallucinations” in the global economy, enterprise leaders focused on explainability, reliability, and transparency when adopting these technologies.
Despite this, a recent Salesforce survey showed nearly two-thirds of executives link trust in AI to revenue growth. Yet, sectors like legal face declining trust as Stanford’s HAI (‘Human-Centered Artificial Intelligence’) program reported that AI hallucinated answers in 75% of court-related queries.
At this year’s VentureBeat Transform event, Capgemini Executive Vice President of Data-Driven Business & Trusted AI Steve Jones highlighted that scaling AI requires tackling issues like bad data and ineffective digital models. He emphasized creating clear AI boundaries, integrating AI into business functions, and promoting human-AI collaboration to mitigate risks.
Steve recently sat down with Emerj Senior Editor Matthew DeMello shortly after the VentureBeat event to talk about the evolving landscape of AI development and the critical need for trust and ethical guardrails in deploying AI systems across enterprises.
Drawing from many of the same insights he shared with VentureBeat, Steve gives his perspective on the state of AI and the challenges that lie ahead.
The following article summarizes Steve’s vision as expressed on the podcast, with special attention given to the following areas for leaders across industries:
- Shifting focus from data quality to data accuracy for effective AI operations: Prioritize managing data accuracy over merely ensuring data quality, as AI applications increasingly rely on real-time, accurate data to operate effectively in dynamic environments.
- Redefining trust in AI across business functions: Explicitly define trust in AI, tailored to the unique requirements of each business function, recognizing that as AI adoption expands, trust becomes an organizational challenge requiring collaboration between human expertise and AI capabilities.
- Establishing an AI resources department to manage systemic compliance risks: Create an AI Resources Department to ensure compliance with evolving industry regulations and frameworks, such as the EU AI Act, focusing on managing systemic risks associated with AI technologies.
Listen to the full episode below:
Guest: Steve Jones, Executive Vice President of Data-Driven Business & Trusted AI at CapGemini.
Expertise: AI Safety, AI Development
Brief Introduction: Steve has spent the last 21 years at Capgemini, starting as Head of Enterprise Java in 2003. Before joining Capgemini, Steve worked across various technology and service spaces in various leadership and consulting positions. Logos include Mac UK, Tanning Technology, and Siemens Plessey, going back to 1993. Steve Graduated from the University of York in 1993 with a Bachelor of Engineering honorary degree in Computer Science & Software Engineering.
Shift Focus from Data Quality to Data Accuracy for Effective AI Operations
Steve opens the conversation by discussing the shift in focus from ensuring the quality of individual AI solutions to managing the accuracy of data across multiple AI applications within an organization. He explains that, in the past, the focus was on establishing trust in single AI models. Now, the challenge lies in governing multiple AI solutions and ensuring data accuracy for operational use cases.
He highlights that while “data is the new oil” captures the value of data, it also means that raw data is often unusable without extensive refinement. The problem is that AI is now being used as the source of data (“the wellhead”), making decisions in real-time contexts like call centers and procurement.
Steve notes that ‘drinking’ data in these contexts requires AI to operate on data as it is generated, not after it has been cleaned up. He uses the analogy of GPS routing to emphasize how a routing algorithm based on six-hour-old traffic data is useless—AI needs to work with up-to-date, accurate data:
“But most organizations today, their data governance is set up to make the data good enough to be able to do routing based on the traffic from six hours ago. If we’re serious about AI deployment, then the people who build applications – as I have in my background – have to start caring about data accuracy. Not quality, because quality is what we do when we clean up bad data. Accuracy is getting it right the first time.
AI requires that fundamental mindset shift if we’re going to be able to rely on AI in production and in operations.”
—Steve Jones, Executive Vice President of Data-Driven Business & Trusted AI at Capgemini
Redefine Trust in AI Across Business Functions
Steve explains that as AI adoption becomes more systemic and widespread, organizations need to redefine what “trust” means in different business contexts. He gives the example of how trusted AI has now become relevant in unexpected areas like procurement, where companies must evaluate which AI models to use, where they were sourced, and whether the models have been tampered with. ‘Trusted AI’ is a new dimension of procurement, traditionally focused on supplier stability and product quality.
He argues that current frameworks for AI governance, which allow for subjective application of standards, won’t work when AI is used at scale. Instead, trust needs to be defined explicitly based on each business area’s specific requirements. For example, the level of trust required for an AI handling safety-critical systems is vastly different from that for a customer service chatbot. As AI starts to take on roles traditionally managed by people, trust in AI becomes an organizational challenge, not just a technical one.
Steve emphasizes that this shift means each business function—such as marketing, logistics, or procurement—must be clear about what trusted AI means for them. They need to retain control and not defer to other departments like IT or external vendors. These levels of control require tailoring AI trust frameworks to address specific business needs and ensuring stakeholders understand and feel confident in AI’s role within their processes.
He also talks about the concept of “hybrid intelligence,” which he believes is crucial for businesses aiming to leverage AI effectively. This term refers to the integration of human expertise with AI capabilities. Organizations will need individuals who not only understand their specific fields—like marketing and procurement—but also have a grasp of AI technologies to facilitate collaboration and enhance decision-making.
He further observes that many businesses already have personnel writing SQL and generating reports themselves, indicating a trend where non-technical staff engage with data analytics. With the advent of Generative AI, this trend is likely to accelerate, leading to more employees creating dashboards and utilizing AI tools. However, he stresses that the needs and goals of different departments—such as marketing and procurement—are distinct and must be addressed separately.
Establishing an AI Resources Department to Manage Systemic Compliance Risks
Steve discusses the importance of implementing adequate guardrails in AI solutions, distinguishing between two types:
- Enterprise guardrails: Enterprise guardrails act as overarching security measures that block harmful inputs or prompts at the organizational level, preventing problematic interactions from even reaching the AI system. For instance, he suggests blocking prompts like “Write me a haiku” as these do not align with business objectives.
- Solution-oriented guardrails: These focus on the specific components of a solution. Instead of trying to guard a complex, monolithic AI solution, Steve advocates for breaking it down into smaller, manageable pieces. By decomposing a solution into distinct functions, each with a clear purpose, organizations can create targeted guardrails that define acceptable and unacceptable behaviors for each component. This approach not only simplifies the management of the AI system but also helps prevent it from producing undesired outcomes.
In the end, Steve highlights the evolving landscape of compliance related to AI, which he believes operates on two key dimensions: industry compliance and regulatory frameworks, such as the EU AI Act. He notes that various regions are actively working on establishing regulations for AI, emphasizing the need for organizations to adapt their compliance structures to account for the complexities introduced by AI technologies.
Steve also anticipates the emergence of an “AI Resources Department,” akin to HR, that will focus on managing the systemic risks associated with AI rather than simply solution risks. This department’s role will involve ensuring that compliance with industry regulations and frameworks, like the EU AI Act and guidelines from bodies like NIST, is effectively implemented in AI operations.
He stresses that this shift represents a significant change from traditional technology management, where risk discussions primarily focused on cyber security. As organizations increasingly rely on nonlinear AI systems that make complex compliance decisions, managing systemic risk becomes paramount.
Steve predicts that this redefined focus on risk management will be a critical evolution for businesses in the coming 18 months, requiring a clear distinction between managing systemic risks (related to AI governance) and solution-oriented risks (related to specific AI implementations).