Top 5 AI Startups in Banking by Funding – A Brief Overview
There are numerous AI vendors servicing the banking industry, but many of them lack the kind of funding that these five…
•
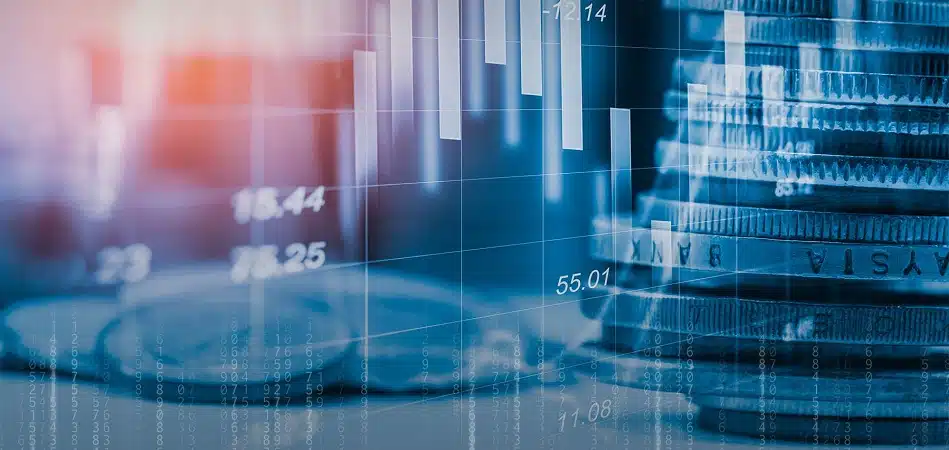
Raghav is serves as Analyst at Emerj, covering AI trends across major industry updates, and conducting qualitative and quantitative research. He previously worked for Frost & Sullivan and Infiniti Research.
There are numerous AI vendors servicing the banking industry, but many of them lack the kind of funding that these five…
•
Banks are starting to deploy natural language processing (NLP) to make use of enterprise and customer data in text mining applications…
•
Business process management (BPM) in banking involves the automation of operations management by identifying, modeling, analyzing, and improving business processes. Many…
•
Automated loan processing and underwriting is not a new concept in the banking and financial services industry. Lenders have consistently faced…
•
Insurers are looking to leverage all of the digital customer data that is now available to them, including one new data…
•
Identity fraud was the number one method of fraud that affected businesses globally according to The Communications Fraud Control Association’s (CFCA)…
•
Robotic Process Automation (RPA) is a rule-based software solution that automates repetitive tasks without any self-learning capabilities. It is not inherently…
•