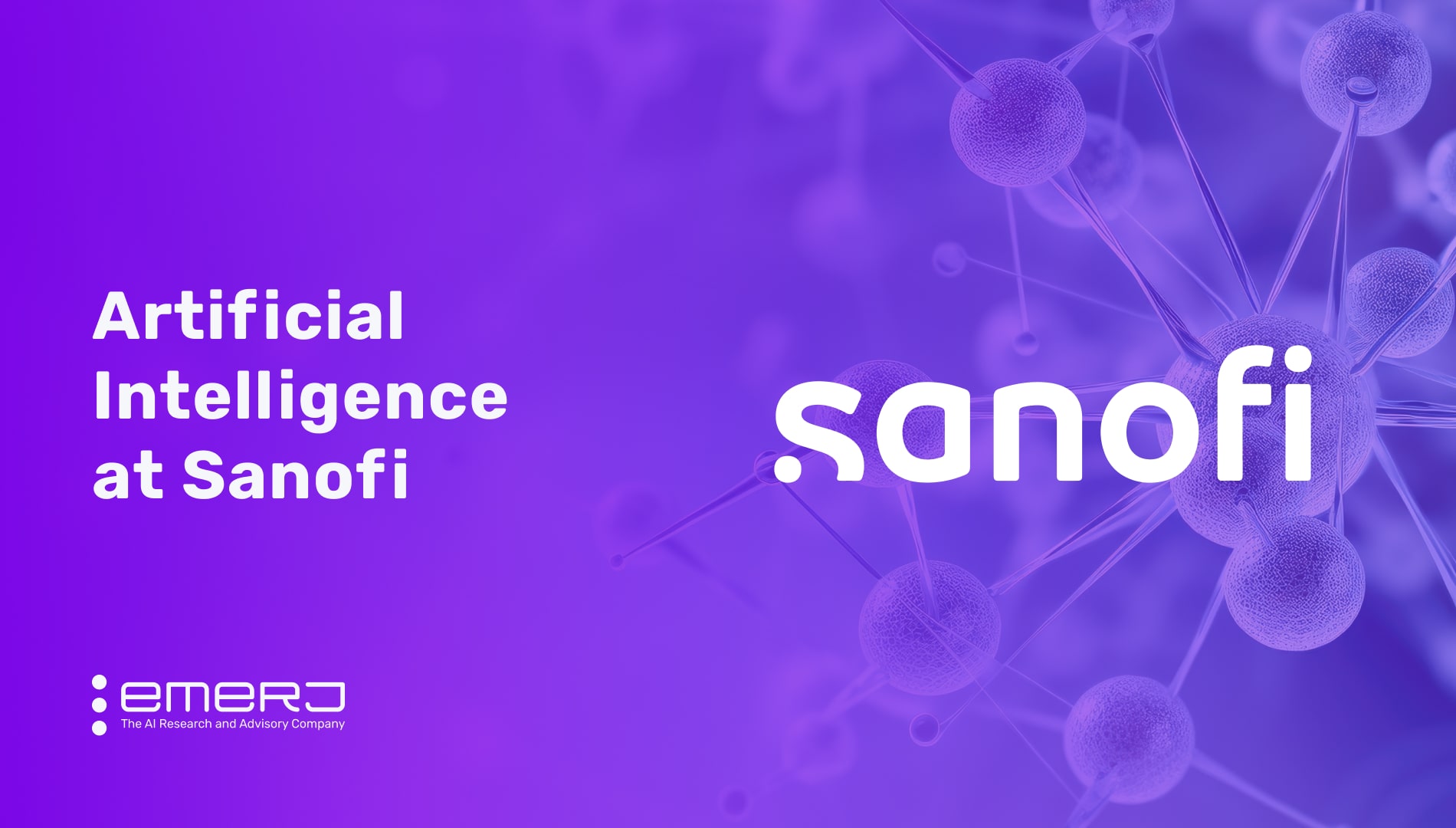
Sanofi is a global healthcare and pharmaceutical company. Founded in 1973, it aims to unlock and maximize the potential of critical medicines, vaccines, and self-care solutions for individuals. It accomplishes this across four global business units: Specialty Care, Vaccines, General Medicines, and Consumer Healthcare.
Sanofi is a Fortune 500 global company and employs over 113K individuals. In 2023, Sanofi reported revenue of over $43.7 billion.
Sanofi has made a commitment to Responsible AI in large part because the company recognizes the need to balance innovation with optimal risk management. Their commitment to Responsible AI is guided by five key principles: “Accountable to Outcomes, Fair & Ethical, Robust & Safe, Transparent & Explainable, and Eco-Responsible.” You can read more in their formal statement, which is available on their website.
Sanofi has implemented AI internally to achieve multiple goals, including accelerating drug discovery and enhancing clinical trial design. To improve internal capabilities, Sanofi established collaborations with leading AI companies, including BioMap, Aily Labs, Insilico Medicine, and Exscientia. They’ve also advanced their AI goals through an equity investment of $180 million in Owkin.
This article will examine two use cases demonstrating how Sanofi uses AI to benefit patients and support their core business goals:
- Improving cancer treatment for patients: Optimize clinical design trial and detect predictive biomarkers for diseases and treatment outcomes using federated learning.
- Shorten drug discovery timelines: Accelerating identification of new protein therapies for drug discovery by using automation and integrated workflows to leverage an AI-driven platform that can develop up to 15 novel minor molecule candidates across oncology and immunology.
Use Case #1: Improving Cancer Treatment for Patients
In oncology care, patients’ needs can be challenging to meet, particularly when it comes to cancers that are difficult to treat. For example, triple-negative breast cancer (TNBC) is an aggressive breast cancer subtype that is difficult to treat even when diagnosed early.
Sanofi’s equity investment in Owkin will facilitate a three-year collaboration that will help identify and develop new treatments for the following four types of cancer:
- Non-small cell lung cancer
- Triple-negative breast cancer
- Mesothelioma
- Multiple myeloma
According to press releases from both companies, Sanofi leveraged the Owkin platform in several ways as part of the collaboration, including:
- Find new biomarkers and therapeutic targets
- Build prognostic models
- Predict treatment response from multimodal patient data
Additionally, both partners claim the collaboration helped Sanofi identify the best candidate gene targets with associated subpopulations of interest. The benefit is that it enables Sanofi to understand an individual’s unique biology and design tailored treatments based on that knowledge.
These capabilities go a long way toward supporting the goals of their Managed Access Programs (MAPs), which allow patients who cannot enroll in ongoing clinical trials to access investigational medicines.
The ability to find new therapeutic targets was accomplished through Owkin’s AI target discovery engine. The engine applies interpretable AI models to multimodal patient data to identify new and novel targets. Multimodal data can include clinical data, histology, spatial transcriptomics, and RNA-Seq.
The discovery engine uses a four-step methodology:
- Step 1: Data Access. This preliminary step involves gathering, curating, and preparing multimodal patient data.
- Step 2: Patient Characterization, where AI is applied to multimodal patient data to characterize patients based on disease biology.
- Step 3: Target Characterization, which involves applying AI to prior knowledge for the purpose of using genetic and molecular features to characterize patients.
- Step 4: AI reasoning, where both AI and human reasoning are combined to optimize and prioritize target-subgroup pairings.
The nearly three-minute-long video below gives some deeper insights into how Owkin can predict response to treatment:
While there are no published outcomes from this strategic collaboration, Owkin announced that they are expanding their partnership with Sanofi to include immunology, which suggests a successful result of their proprietary efforts.
Additionally, Owkin has had similar and verifiable successes, as evidenced in their case study on HE2RNA, which focused on predicting gene expression from H&E slides published in Nature.
Owkin developed a model, HE2RNA, that predicts the transcriptome without the need for RNA-Seq. As a result, they were able to identify novel biomarkers in images of specific tumoral regions, which ultimately enhanced their understanding of disease evolution and differentiated outcomes.
Use Case #2: Shorten Drug Discovery Timelines
Making accurate predictions about which biological sources will lead to therapeutic drugs is a challenge. Identifying suitable proteins or biological sources to execute on is crucial because it can take 12 to 15 years to get a drug to market.
Thousands of patients die each year in the U.S. alone, waiting for drugs to be approved. AI and machine learning methods offer the potential to expedite that process by zeroing in on ideal targets in drug discovery.
As BioMap claimed in a press release, the vast amounts of labeled data traditional AI methods require are in short supply.
According to BioMap use case documentation, Sanofi partnered with the startup biotech company to develop AI modules for biotherapeutic drug discovery. The goal of the partnership is to create advanced AI models and protein large language models (LLMs) by leveraging BioMap’s custom-built functional models in conjunction with Sanofi’s proprietary data and computational innovations in protein engineering.
Below are screenshots displaying a state-of-the-art overview of LLMs that are part of BioMap’s large-scale AI engine and specific performance details for a protein binding prediction affinity model.
BioMap’s State-of-the-Art Overview for LLMs from their large-scale AI engine. [Source: BioMap]
BioMap’s State-of-the-Art Overview and Performance for Protein Binding Affinity Model from their large-scale AI engine. [Source: BioMap]
The partnership was just released in October 2023, so tangible benefits or observed results have yet to be reported as development is ongoing.
According to a Sanofi press release, the company also partnered with Exscientia to develop up to 15 novel minor molecule candidates across oncology and immunology using actual patient samples. Exscientia’s platform enables a patient-centric approach by integrating primary human tissue samples into early target and drug discovery research.
While the company has not revealed any specific outcome data regarding the results pertaining to the development of the 15 novel minor molecule candidates, Exscientia has already had success using the same AI-driven drug discovery platform to create two therapeutic agents, an LSD1 inhibitor and a Malt1 protease inhibitor that have reached the IND-enabling phase.