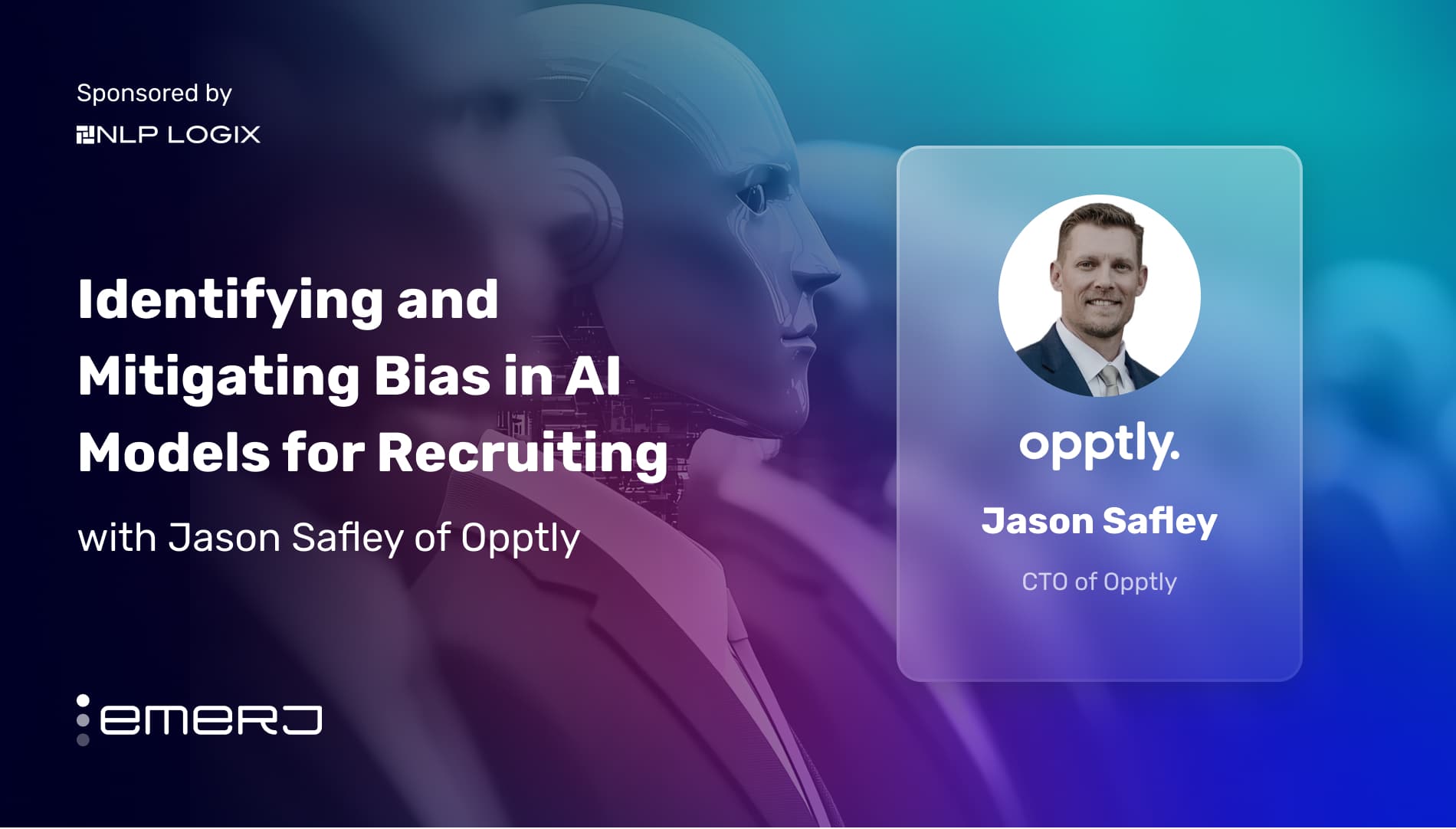
This interview analysis is sponsored by NLP Logix and was written, edited, and published in alignment with our Emerj sponsored content guidelines. Learn more about our thought leadership and content creation services on our Emerj Media Services page.
AI bias has emerged as a critical issue in the development and deployment of AI systems, particularly in recruitment. A Harvard Business Review primer on bias even from as far back as 2019, titled “All the Ways Hiring Algorithms Can Introduce Bias”, highlights that, while AI can enhance efficiency and reduce human error in hiring, it can also perpetuate and even exacerbate existing biases.
In the hiring space, AI bias poses significant risks as companies increasingly rely on automated systems to screen candidates. According to the IBM Global AI Adoption Index 2023, 42% of companies are using AI screening tools, with many hoping to eliminate biases from the hiring process. However, these tools can inadvertently filter out highly qualified candidates based on irrelevant criteria, such as body language or educational background.
Emerj Senior Editor Matthew DeMello recently sat down with Jason Safley, CTO at Opptly, to talk about leveraging AI to create fair, skill-based talent evaluations by minimizing bias, ensuring privacy, and combining automation with human oversight for ethical and effective hiring practices.
Opptly is an AI-based hiring platform that helps businesses find and connect with suitable job candidates.
From their conversation, we bring out the following two key insights:
- Ensuring privacy and minimizing bias in AI models: Adopting a data minimization approach by excluding unnecessary personal information (PII) when training AI models to focus solely on skill-related data.
- Conducting regular model reviews and retraining: Performing monthly model health reviews with teams to evaluate metrics, identify potential biases, and analyze model performance to use the insights to trigger retraining during updates.
Guest: Jason Safley, Chief Technology Officer, Opptly
Expertise: Artificial Intelligence, Business Intelligence, Data Analytics, Workforce Technology Strategy
Brief Recognition: With over 20 years of distinguished experience in information technology, data science, and workforce solutions, Jason has architected and lead high performing teams responsible for the design, development, and deployment of some of the most innovative persona-based applications and data-driven solutions in the industry. Over the years, he progressed through various technical and leadership roles, demonstrating an exceptional ability to align technology initiatives with business objectives and spearheading transformative projects that have consistently delivered measurable results.
Ensuring Privacy and Minimizing Bias in AI Models
Jason explains that he and his team developed Oppty’s AI-driven applications to address two main industry pain points: helping recruiters identify talent quickly and accurately and providing candidates with job matches tailored to their skills.
He describes a shift in talent evaluation from traditional role-based assessments to a more skills-focused approach powered by AI. In his view, the industry has historically evaluated candidates based on their job titles and backgrounds, like degrees or specific past roles. These traditional methods often introduce biases, such as favoring particular educational backgrounds.
Oppty’s AI models, however, evaluate candidates by matching their contextual skills to job requirements rather than relying on titles or potentially biased attributes. By using AI to understand and assess required job skills, recruiters can avoid manual skill assessments and reduce biases, enabling a more objective and skills-centered hiring process that matches candidates to roles based on genuine capability.
He also explains that Opptly’s AI model is built with a strong emphasis on privacy and bias prevention. First, he notes that they purposefully exclude unnecessary personal PII when training the model, following a data minimization approach that focuses solely on relevant skill-based data. The approach ensures the model is trained only on essential, skill-related information.
To further ensure fairness, they use a tool called LangTest from John Snow Labs, designed to detect potential biases in AI models. The tool injects specific demographic attributes — like nationality, pronouns, or educational background — into test samples to see if the model’s outputs vary when these details are changed.
For instance, if the model’s evaluation of a “Susan Smith” changes when her country of origin or pronouns are modified, it could indicate bias. By analyzing such variations, they can identify and address biases, ensuring the model evaluates candidates consistently based on skills alone.
Jason highlights that the transformative power of their AI models lies in understanding a person’s “skills DNA,” which goes beyond explicitly stated qualifications like degrees or listed skills on a resume. Drawing from his own experience — rising to a CTO role without a college degree — he explains how traditional hiring practices often overlook an individual’s true capabilities in favor of formal credentials. Driving such personal perspective fuels his passion for leveraging AI in talent evaluation.
He shares that Opptly’s AI not only identifies skills explicitly mentioned on a resume but also infers additional, less apparent skills by analyzing a candidate’s work history. By comparing an individual’s career path with others who have similar backgrounds, AI fills in “inferred skills” that might not be explicitly listed.
Delivering a deeper understanding ensures that candidates who may not excel at presenting their skills on a resume are still accurately evaluated, making them more relevant and competitive for job opportunities. In-depth hiring intelligence also bridges the gap between surface-level qualifications and a more comprehensive view of a candidate’s potential.
While Opptly’s AI identifies candidates with the right skills for a role, it leaves the final assessment of competency and fit to humans. A “human-in-the-loop” approach ensures compliance and balances AI efficiency with human judgment.
Conducting Regular Model Reviews and Retraining
Jason tells Emerj about Opptly’s zero-tolerance policy for bias in their AI models while explaining their rigorous approach to detecting and addressing it. They use the LangTest library to identify potential bias, with a 1% threshold serving not as an acceptable limit but as a trigger for deeper analysis. If the threshold is exceeded, they investigate the causes — often related to parsing challenges like poorly formatted documents — and take corrective actions. Bias mitigation involves retraining their AI model during regular updates and conducting monthly health reviews with their team to evaluate metrics and determine if retraining is necessary.
He also talks about the proactive and collaborative nature of Oppty’s partnership with NLP Logix, emphasizing its role in maintaining a responsible AI model. He shared that NLP introduced the LangTest framework to rigorously test their model, demonstrating their commitment to responsible AI. Additionally, NLP also brought in Pacific AI as a third-party auditor to ensure unbiased practices and compliance. Jason views NLP as a strategic and indispensable partner, contributing both to ongoing model testing and enhancing their AI’s accountability and reliability.
“The complexity of AI legislation in our industry lies in its fragmented introduction. In the U.S., regulations are emerging on a state-by-state basis, and occasionally at more local levels, like New York City’s Local Law 144. Managing compliance becomes challenging as we must navigate and align with the strictest regulatory standards across these varied jurisdictions to maintain overall AI compliance. The EU has introduced overarching regional legislation that broadly classifies AI systems used in a hiring and HR as high-risk. That’s where Pacific AI comes into play for Opptly, they continually help us to monitor and adjust our governance framework and AI policies to be able to address any of this new introduction of legislation.”
– Jason Safley, Chief Technology Officer at Opptly
Third-party auditors play a critical role in monitoring legislative changes, proactively advising on updates to their AI policies, governance frameworks, and testing processes to ensure compliance. As laws evolve, organizations must adapt by revising governance, policies, and testing methods to maintain transparency and defensibility in their AI outcomes. Opptly’s partnerships with auditors ensure they stay ahead of regulatory changes and maintain a responsible, compliant AI model.
Towards the end of the conversation, Jason emphasizes the growing importance of automation in processes and the responsible use of AI, especially in light of advancements like ChatGPT. While AI adoption has accelerated, a key concern in the industry is the need for more transparency, governance, and defensibility around how AI is implemented.
He highlights the need for clear compliance measures, including informing individuals when AI is used in evaluations and ensuring they consent to it. Additionally, organizations must provide transparency on how their AI interprets data. These practices are increasingly shaped by legislation, which demands accountability and ethical AI usage.