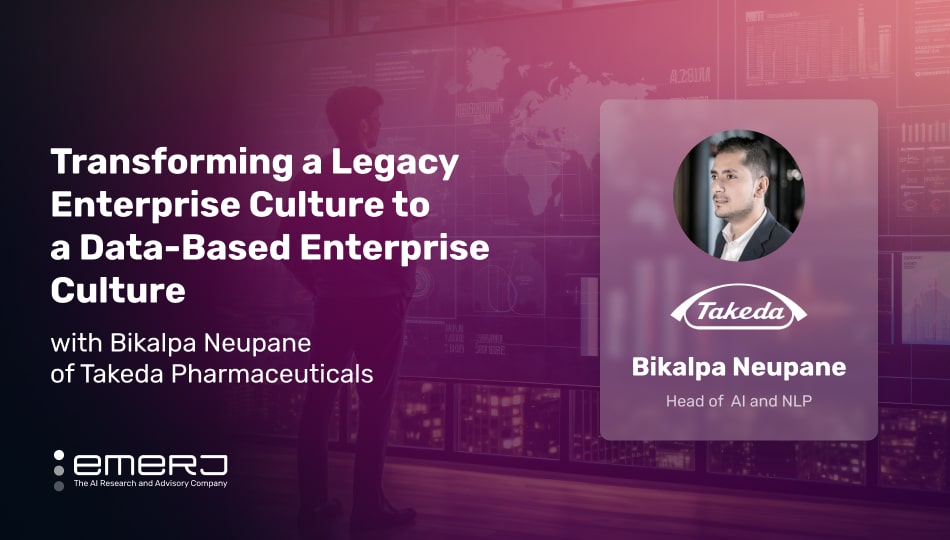
Fostering a culture of experimentation in AI and data science is crucial for organizations to stay competitive and drive innovation. In 2021, an MIT Sloan report found companies that prioritize AI experimentation are 2.7 times more likely to capture new value and improve their operations. By embracing a more experimental mindset, organizations can harness the full potential of AI-driven tools and unlock new opportunities for growth.
Experimentation, and a safe environment for it, is always a challenge for typically defensive enterprises and industries. As highlighted in the Harvard Business Review, the primary obstacle to conducting numerous tests is not technology or tools but culture itself. By creating an environment that nurtures curiosity, values data over opinion, and empowers anyone to conduct tests, companies can leverage AI and data science more effectively, leading to continuous improvement and innovation in their digital products and services.
Bikalpa Neupane, Head of AI/GenAI and NLP at Takeda Pharmaceuticals, recently discussed the importance of fostering a culture of experimentation in AI and data science on the ‘#AIinBusiness’ podcast. His company uses an “experimentation as a service” model in their AI Lab to manage the complexities and costs of scaling AI, handling around 100 use cases.
Neupane identifies core and citizen data scientists as key organizational roles and offers life sciences leaders practical strategies for cultivating a ‘data-driven culture’ within their organizations. The following analysis of their conversation highlights two key insights:
- Prioritizing AI projects based on impact and execution: Using a graph to evaluate AI projects by business impact and ease of execution to prioritize them systematically.
- Enhancing data scientist engagement with supportive infrastructure: Fostering a supportive “data scientist experience” by providing well-developed platforms and encouraging meaningful work to retain and engage your data science talent.
Listen to the full episode below:
Guest: Bikalpa Neupane, Head of AI/GenAI and NLP at Takeda Pharmaceuticals
Expertise: NLP, AI, predictive analytics, leading enterprise-wide Generative AI strategy
Brief Recognition: Neupane previously worked in product development and research at IBM Watson. He received his PhD in Informatics—AI and HCI from Penn State University.
Prioritizing AI Projects Based on Impact and Execution
Bikapla highlights the need for a culture of experimentation in AI and data science, using his company’s “experimentation as a service” in the AI Lab to explore new technologies. Scaling AI poses challenges due to high costs and uncertainty, requiring a balanced approach between experimentation and strategic implementation.
He tells the Emerj executive audience that his team at Takeda manages around 100 use cases and prioritizes projects by establishing AI architecture principles and criteria for demand management.
Bikalpa suggests assessing demand from two perspectives: business impact and ease of execution. By placing business impact on the x-axis and ease of execution on the y-axis, they can plot and evaluate different use cases on this graph. This approach helps in systematically prioritizing projects based on their potential business benefits and the practicality of their implementation.
He then delves deeper into what factors should be considered when assessing the business impact of AI and data science projects. Bikalpa emphasizes using classic product management principles, focusing on alignment with the organization’s key strategic areas and corporate goals. Doing so, he insists, ensures life sciences leaders that new technologies are not adopted merely because they are trendy but because they support the company’s vision.
Key factors to consider include:
- Customer Size: Whether the customers are internal or external, as this affects the project’s scope and impact.
- Value Realization: The tangible benefits such as cost savings, increased productivity, or the introduction of new products.
- Financial Impact: The return on investment (ROI) of the data science work.
Additionally, he stresses the importance of considering future relevance and adaptability, advocating for designs that remain relevant and modular to adapt to future changes. These initiatives ensure long-term viability and avoid the need to scrap work due to rapid technological advancements. He highlights the importance of communicating these considerations to executive leadership to ensure alignment and support.
Bikapla discusses the importance of designing AI and data science solutions with a modular approach, given the rapid evolution of the field. He emphasizes that what is developed today should not become obsolete in six months or a year. By keeping designs modular, companies can easily replace parts without having to discard the entire system.
On the business side, this modularity helps in evaluating AI investments. For the technical execution, beyond the scaling costs, it’s crucial to ensure that projects are feasible within the organization’s technical assets, including data, infrastructure, and talent. Additionally, evaluating the capacity and effort required for data science work is essential.
“But if you look at the technical execution, which – other than the scaling cost that I mentioned earlier – the cost of doing AI makes sure that, when you evaluate the use case and an idea, is it feasible, within the scope of your organization, your technical assets? That means your data, that means infrastructure, and that is your talent. And then, you go about having the conversation on the capacity and sizing. What is the level of afford that you need to be ready for the data science work?”
–Bikalpa Neupane, Head of AI/GenAI and NLP at Takeda Pharmaceuticals
Enhancing Data Scientist Engagement with Supportive Infrastructure
Data science is a broad field that encompasses various roles, similar to software engineering. Bikalpa categorizes data scientists into two main groups:
- Core Data Scientists: These individuals often hold advanced degrees (PhDs) and possess deep technical knowledge and expertise in data science and AI.
- Citizen Data Scientists: These professionals typically work within business units and have strong business domain experience along with unique skills. They contribute by leveraging data science techniques to solve business problems.
Before building a data science function, it’s crucial to understand these different personas and the specific roles they play within the organization. This understanding ensures that the right mix of skills and expertise is brought into the team, aligning with the organization’s goals and needs.
Finally, he emphasizes the importance of hiring and retaining the right talent. Given the current macroeconomic conditions, hiring may be somewhat more accessible due to a larger pool of candidates. However, retention is crucial. To retain talent, companies need to ensure employees feel intellectually stimulated, culturally integrated, and included in the broader data science practice.
He highlights the importance of differentiating between types of data scientists during the hiring process:
- “Rockstar” PhDs: These individuals have advanced expertise in algorithms, mathematics, and problem-solving, often contributing significantly to cutting-edge research and development.
- Tool and Platform Specialists: These professionals are skilled in using specific data science tools and platforms, such as Dataiku and DataRobot.
Confusing these two types of data scientists can create disconnects within the team and harm the overall culture. To foster a cohesive and effective team, Bikalpa insists, it’s essential to recognize and respect the distinct contributions each type of data scientist makes:
“The second component, I would say, is: When you have hired them, then utilize them. I’ve said in so many conversations where I’ve realized that it’s a common occurrence in organizations to hire a bunch of data science talent, but then they quickly go into these frustration cycles when their skill set is not as utilized or accounted for in the decision-making process.
If you look at the mental model of these technical folks – the data scientists and AI engineers – they would like to be mentored by folks who are technical. They would like to have someone who is out there; they can intellectually develop their models with them. As much as they love this whole organizational process, they also have a strong urgency to action. And so make sure that we enable the data scientists that we hired as a part of the whole process, but also prompt them to some kind of action.”
–Bikalpa Neupane, Head of AI/GenAI and NLP at Takeda Pharmaceuticals
Bikapla then highlights the importance of engaging data scientists by tapping into their excitement about models, production outcomes, dashboards, and accuracy metrics. He emphasizes that it’s crucial to address their enthusiasm and provide meaningful work that aligns with their interests to retain and motivate these data science teams.
Next, Bikalpa introduces the idea of “data scientist experience,” paralleling it with “developer experience.” Just as companies have historically invested in improving infrastructure, platforms, and APIs to enhance the developer experience, they should also focus on creating a supportive environment for data scientists.
Life sciences can support such environments by ensuring that platforms, libraries, and frameworks are well-developed and ready for new data scientists to use effectively from day one. This approach helps data scientists become productive and integrated quickly, contributing to their overall satisfaction and success within the company.
“So data science is a lot like venture capital. So you write lots and lots of bets, expecting most of them to fail, but you are betting on that particular one, which is going to provide you with a big payoff. That brings the point home to allow data scientists an environment to experiment. They are so inclined to do things right away. Provide them the data, access to the sandbox environment, an AI lab environment where they can enter the process as early as possible.”
–Bikalpa Neupane, Head of AI/GenAI and NLP at Takeda Pharmaceuticals